The growth feature and its diagnostic value for benign and malignant pulmonary nodules met in routine clinical practice
Introduction
According to the global cancer statistics from 2018, there is an estimated 18.1 million new cancer cases and 9.6 million cancer deaths. Among them, primary lung cancer is the most commonly diagnosed cancer, and at the same time the leading cause of cancer death (1). Data from National Institute of Health revealed that the 5-year overall survival of lung cancer was only 19.4% in between 2009 and 2015 and that distant metastasis occurred in 57% of the lung cancers on diagnosis (2). To achieve early diagnosis of lung cancer and therefore improve its prognosis, professionals and health authorities in the field have organized and performed a couple of low-dose computed tomography (LDCT) lung cancer screening trials (3-10). The most famous National Lung Screening Trial found that, compared to radiography, there was a 20% reduction in lung cancer mortality by LDCT (5). However, the main concern regarding LDCT screening is the high false positive rate (11), which consequently leads to overdiagnosis, radiation exposure, medical costs, and psychological anxiety (12).
Given the existing concerns, it is vital to develop optimized protocols for the efficient management of pulmonary nodules. Currently, one of the key characteristics associated with lung cancer probability is nodule size, while another acknowledged characteristic is their growth rate (13). As suggested by the guidelines, if nodule growth occurs during follow-up, clinicians should remain alert and take appropriate actions, such as by reducing the follow-up interval, arranging positron emission tomography-CT (PET-CT) or chest CT with contrast, planning tissue sampling, or even surgery, depending on the probability of malignancy and comorbidities (14-18). Regarding the measurement of nodule growth, volume doubling time (VDT) is well known and accepted (19,20). In a pre-specified analysis of data from the acknowledged Dutch-Belgian randomized lung cancer screening trial (NELSON), Horeweg et al. found that in patients with VDT of 400 days or less, 400–600 days, and 600 days or more, the lung cancer probability was 9.9%, 4.0%, and 0.8%, respectively (21). Similarly, using NELSON data, Xu and colleagues revealed that growth rate either at 3-month or 1-year follow-up was a strong predictor for malignancy (22).
However, while previous studies demonstrated the predictive value of VDT for pulmonary nodules among screening patients, the growth characteristics of nodules in routine clinical practice lack clarity. Lately, increasing numbers of patients have visited the clinic with pulmonary nodule, detected either during an annual examination or during a special examination owing to discomfort. Therefore, our study aimed to clarify the growth characteristics of pulmonary nodules in routine clinical practice and examine whether VDT can predict the malignancy of such nodules.
Methods
Patient selection
The current study enrolled patients with pulmonary nodules measuring 5–30 mm in size that were surgically resected in West China Hospital between 2010 and 2017. Study subjects were selected if the nodule was followed up before surgery and if the follow-up interval was of at least 3 months. A detailed flowchart of patient selection is shown in Figure S1. Clinical data of patients were collected that mainly included demographic characteristics, such as sex, age, smoking status, history of malignancy, and family history of lung cancer. In addition, relevant tumor markers were collected if available, including carcinoembryonic antigen (CEA), cytokeratin 19 fragment (CYFRA21-1), neuron specific enolase (NSE), CA-19-9, and CA-125. The institutional review board of West China Hospital approved this retrospective study, with a waiver of the requirement for patient informed consent.
CT image acquisition and interpretation
Chest CT examinations were performed using one of the three multidetector CT systems: (I) Philips Medical Systems, Eindhoven, the Netherlands; (II) Siemens Medical Systems, Forchheim, Germany; Brilliance 64; and (III) Somatom Definition, Siemens Healthcare, Forchheim, Germany). To avoid artefactual differences in measurements, images with the most similar parameters (thickness and intravenous contrast) as well as long intervals were paired for further analysis. In total, 49% of the paired images were 1 mm and 59% scans were consistently undertaken either with or without intravenous contrast (Table S1). The median follow-up interval of benign and malignant nodules was 230 and 224 days, respectively.
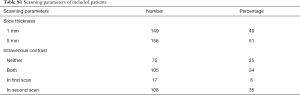
Full table
The following radiological characteristics of the studied pulmonary nodules at initial detection in each patient were recorded: (I) diameter, recorded as the mean of the longest diameter of the nodule and its perpendicular diameter in lung window and rounded to the nearest whole number; (II) location, recorded as “upper” if the nodule was in the upper lobe of the right or left lung, and otherwise recorded as “lower or middle”; (III) regularity, recorded as “yes” when the nodule was regular, and otherwise recorded as “no”; (IV) texture, recorded as “solid” if the whole nodule was composed of a solid component, as “mGGO” if it was a combination of both ground-glass opacity (GGO) and solid component, or as “pGGO” when there was no solid component but only GGO (lesions of homogenous density and with hazy increase in density that did not obscure the bronchovascular structure); (V) spiculation, recorded as “yes” if there were lines radiating from the margins of the tumor, otherwise recorded as “no”; (VI) lobulation, recorded as “yes” if the tumor outline curved, with concave notches between the curves, forming a lobulated shape, and otherwise recorded as “no”. One clinical physician (Panwen Tian) in pulmonary and critical care medicine, with 12 years of experience in reading chest CT, evaluated all the images. The reviewer was blinded to the pathologic outcome of each nodule in order to avoid bias.
Nodule segmentation
We used a commercially available three-dimensional (3D) reconstruction system (IQQA-Chest; EDDA Technology, Princeton Junction, NJ, USA). First, CT images were uploaded to the IQQA Workstation. Then, specialists segmented the nodule either in automatic or manual mode, after which the computer instantaneously automatically computed and displayed quantitative measurements from the target segment. At the same time, a 3D reconstruction of segmentation results was created. Finally, specialists stored the reports with the patients’ corresponding image files in the IQQA system.
To avoid measurement bias, one reviewer (Rui Zhang), who was trained in the use of the IQQA system, initially performed manual segmentation of nodules on the first CT images of all patients. One month later, random codes were generated in an EXCEL table and all cases were reordered accordingly. The same reviewer then manually segmented the nodules on the second CT images of all patients. When segmentation was completed, the parameters of nodule size, volume, and mass were recorded. The reviewer was also blinded to the pathologic outcome of each nodule.
VDT
When all segmentations were finished, we obtained both the first and second diameters, and the volume and mass of the nodules. Then, the increased value of each parameter was calculated by subtracting the first value from the second. The relative change in diameter, volume, and mass for each nodule was equal to the proportion of increased value to the first. VDT was calculated based on the Schwartz formula: VDT = [t log2]/[log Vt/V0], where t is the time between scans, Vt is the second volume, and V0 is the first volume (23). In line with previous literature, VDT for nodules was calculated only if there was a change in volume of at least 25% between the first and second scans (7). Consequently, we only calculated VDT of a nodule when the relative volume change was ≥25%.
Statistical analysis
The distribution of continuous variables was described by the mean and standard deviation, and the difference between two groups was compared using a t-test. In addition, the distribution of categorical variables was described by frequency and proportion, and the difference between two groups was compared using the Chi-square test. VDT was compared between groups using the rank sum test (Mann-Whitney U test or Kruskal-Wallis test). A receiver operating characteristic (ROC) curve was drawn to evaluate the diagnostic value of VDT in lung cancer. All analyses were performed using SPSS software 20.0, with P<0.05 being considered statistically significant.
Results
Enrolled patients
In the current study, 305 patients with pulmonary nodules were enrolled, including 219 patients with malignant nodules and 86 with benign nodules. Forty-one patients underwent preoperative diagnostic procedures, and 12 lung cancers were confirmed by bronchoscopy or percutaneous lung biopsy, while 11 were highly suspected by PET-CT. Among all malignant nodules, adenocarcinomas were predominant in 208 cases, whereas the remaining were 7 squamous cell carcinomas, 2 adenosquamous cell carcinomas, and 2 were other types. Benign nodules included 34 inflammatory pseudotumors, 20 granulomas, 18 benign tumors, 3 enlarged lymph nodes, and 11 were other types. The ratio of males to females was 3:5 (112/193), with an average age of 57 and an average nodule diameter of 13 mm.
Table 1 shows that most patients were found to have pulmonary nodules by annual physical examination (168 cases), and some were found to have respiratory symptoms, such as cough, expectoration, and chest pain (108 cases). A few nodules were accidentally found upon clinical examination (29 cases). When comparing patients with malignant and benign nodules, the differences are mainly reflected in the following aspects. In the malignant group, patients tended to be older (59 vs. 49, P<0.01) and a higher proportion of patients had a history of malignancy (18% vs. 4%, P<0.01). Radiologically, malignant nodules were larger (13 vs. 11 mm, P<0.01) and irregular (66% vs. 47%, P<0.01), with a higher proportion of GGO (67% vs. 48%, P<0.01), and had much more spiculation sign (41% vs. 14%, P<0.01) and lobulation sign (45% vs. 23%, P<0.01). Lung cancer patients also had a higher level of serum CA19-9 (13.76 vs. 9.88 UmL, P=0.03).
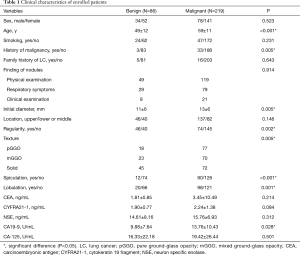
Full table
3D reconstruction parameters
Initial nodule diameter, mass, volume, and changes over the follow-up period were significantly different between the two groups of patients. Malignant nodules were larger and grew over time, whereas the benign nodules remained stable. The mean increased diameter, mass and volume of benign and malignant groups were 0.09 vs. 2.37 mm, 0.10 vs. 0.66 g, and 32.74 vs. 1,871.28 mm3, respectively (Figure 1). As for nodule volume, when conducting subgroup analysis according to nodule texture and follow-up duration, the changing tendency was sustained. For pGGO, mGGO and solid nodules, the mean increased volumes of the benign and malignant groups were 5.89 vs. 333.17 mm3, 189.00 vs. 1,236.16 mm3, and –36.38 vs. 4,133.68 mm3, respectively (Figure 2A). Regarding different follow-up duration periods of 90–180, 180–365 days, and more than 365 days, the mean increased volumes between the two groups were 15.13 vs. 1,234.22 mm3, 179.66 vs. 1,497.07 mm3, and –156.26 vs. 2,950.96 mm3, respectively (Figure 2B). There were more malignant nodules than benign nodules with a change in volume of at least 25% between the first and second scans (malignant, 121/219, 55%; benign, 24/86, 28%). Details are shown in Table 2.
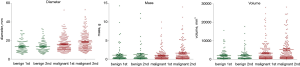
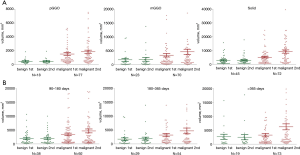
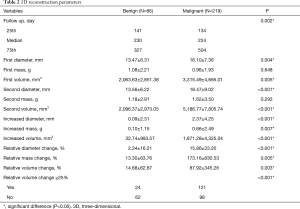
Full table
VDT
Among benign nodules, 62 cases were inert, while the other 24 (28%) cases changed over time. Of these, 6 cases reduced in size, and included 3 granulomas and 3 inflammatory pseudotumors. As for malignant nodules, 98 cases were indolent and the remaining 121 (55%) were altered. Of these 7 adenocarcinomas were reduced in size. Overall, there were 132 growing nodules, 14% benign, and 86% malignant. The median VDT of growing benign and malignant nodules was 389 and 526 days, respectively, (P=0.18, Table 3). When drawing the ROC curve to evaluate the ability of VDT to differentiate between benign and malignant nodules, the area under the curve (AUC) was 0.67 (0.55–0.78), with a sensitivity and specificity of 69% and 58%, respectively (Figure S2).
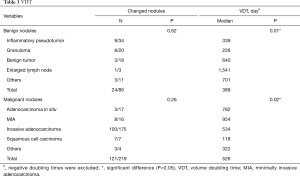
Full table
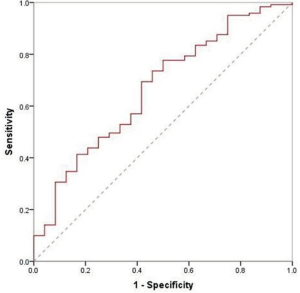
Indolent benign and malignant nodules
Among 86 benign nodules, 62 (72%) were relatively stable, with a median follow-up of 180 days and a mean relative volume change of 12.12%. Among 219 malignant nodules, 98 (45%) were indolent, with a median follow-up of 160 days and a mean relative volume change of 10.31%. Compared to stable benign nodules, indolent malignant nodules showed a higher proportion of GGO (76% vs. 47%, P=0.00) with more spiculation sign (35% vs. 16%, P=0.01). In addition, malignant nodules tended to be larger and more irregular (Table 4).
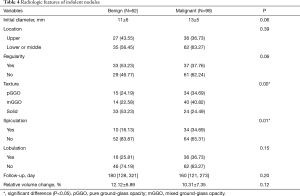
Full table
Discussion
Our study clarified the growth characteristics of pulmonary nodules encountered in routine clinical practice. We found that before surgery, most malignant nodules grew over time, while a high proportion of benign nodules were stable, which was identical in stratified analysis with respect to nodule texture and follow-up duration. As a result, growth is of great importance in the differential diagnosis of lung cancer. However, among the growing nodules, there were still a small number of benign nodules and the VDT could not distinguish them from malignant nodules, with an AUC of 0.67. Moreover, nearly half of the malignant nodules remained stable over time.
Regarding the NELSON trial, the strategy utilized volume and VDT of a noncalcified nodule as the main criteria for deciding on further action (7). In the following pre-specified analysis of NELSON data, Xu et al. found that either 3-month VDT (odds ratio, 15.6) or 1-year VDT (odds ratio, 213.3) was a strong predictor for malignancy for non-smooth, purely intraparenchymal, solid indeterminate nodules between 5 and 10 mm in diameter (22). Similarly, Walter et al. found that VDT had a high discrimination for lung cancer (AUC, 0.91) in terms of persisting new nodules in incidence rounds of the NELSON (24). In addition, Ashraf and colleagues demonstrated that VDT harbored a sensitivity of 71% and specificity of 91% from the Danish Lung Cancer Screening Trial (25). Compared to previous studies, the current study also revealed that growth is critical in the diagnosis of lung cancer, as 52% (114/219) malignant nodules grew over time, while only 21% (18/86) of benign nodules grew. However, we found that the VDT did not have a diagnostic value in growing nodules, and the significant reason was probably due to the predominant diseases. In the current study, 71% of size-changed benign nodules were inflammatory pseudotumors and granulomas, while 60% of size-changed malignant nodules were pGGO or mGGO, which are well known to have longer VDT. Moreover, research subjects were also different from previous studies, which focused more on screening participants.
Our results showed that rapid growth (VDT <400 days) was not exclusive to malignant nodules. Among the rapidly growing nodules in the current study, nine (16%) of 57 were benign (Figure 3). Similarly, de Hoop et al. analyzed 794 cases of pulmonary perifissural nodules and 66 cases of nodules with VDT less than 400 days, but none of them were diagnosed with lung cancer, and one excised nodule was proven to be a lymph node (26). Furthermore, Xu et al. reported that 58 (85%) of 68 rapidly growing solid nodules were found to be benign at a 3-month follow-up and that 5 (50%) of 10 were benign at 1-year follow-up (22). Consequently, the diagnosis of these nodules requires additional attention.
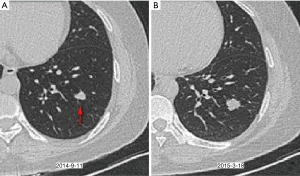
In contrast, malignant nodules can grow slowly (VDT >400 days) or even be stable. In the current study, we identified that 45% (98/219) malignant nodules were basically unchanged during follow-up, and thus, they could not be evaluated based on growth rate. Stable malignant nodules showed a higher proportion of GGO (76% vs. 47%) than stable benign nodules. In addition, 65 (54%) of 121 malignant nodules grew slowly, of which 69% were GGO. Figure 4 shows three examples of indolent malignant nodules. Previous studies have reported a highly variable proportion of slow-growing lung cancers, ranging from 3% to 45% (27,28). It is well known that the indolent course of lung cancer is highly related to their proportion of nonsolid component. Obayashi et al. found that the median VDT of adenocarcinomas with GGO and without GGO were 725 and 177 days, respectively (29). A nonsolid part usually reflects adenocarcinoma in situ (formerly bronchoalveolar carcinoma) or minimally invasive adenocarcinoma (MIA), whereas a solid component usually reflects an invasive component (30,31). Song and colleagues demonstrated that the median VDT of adenocarcinoma in situ and MIA could be as long as 1,240.3 and 1,328.3 days, respectively (32). Given that an increasing number of pulmonary nodules are being detected, it is essential to identify slow-growing or stable malignant lung nodules and manage them with a careful balance of competitive risks related to the natural course of the disease, treatment impact, and life expectancy.
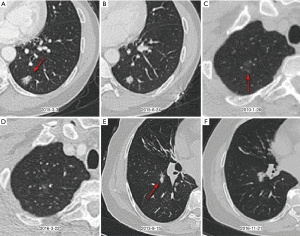
Due to the limitation of growth rate for the differential diagnosis of lung nodules, since benign nodules can grow fast while malignant nodules can grow slowly or even remain stable, other diagnostic markers are required. Recently, radiomics features, a non-invasive method based on radiologic images, have shown potential in lung nodule management. For example, Hawkins et al. established models using 23 radiomics features to assess the risk for development of lung cancer, which outperformed Lung-RADS and nodule volume (AUC, 0.75–0.83) (33). Hanning and colleagues extracted radiomics features from baseline and follow‐up CT scans to predict lung cancer incidence using three nodule size classes (<6, –6–16, and ≥16 mm). They proved that radiomics could be used to improve current size‐based screening guidelines and even worked better when combining baseline and delta radiomics (AUC, 0.84–0.86) (34). Similar deep learning models with high yields were also developed by Ardila et al. (AUC, 0.94) (35). Moreover, researchers have found that radiomics features were also valuable predictors of tumor growth (indolent vs. aggressive, AUC, 0.80–0.86) (36). In short, radiomics is gaining importance in cancer research and will make lung cancer diagnosis more rapid and accurate in the future.
This study had several limitations. First, this was a retrospective, single-center study with inherent selection bias. The sample size was also small and not balanced (86 benign and 219 malignant nodules), which could be another potential reason for bias. Second, when interpreting our findings, it is very important to note the distribution of benign nodules because the percentage of inflammatory pseudotumors was higher than that seen in clinical practice. Third, we did not measure intra-observer variation. The measurement bias was unavoidable and should be considered as it could affect the real growth of a nodule. For automatic segmentation software, the lower limits of agreement range from –21.2% to –19.3%, and the upper limits from 20.4% to 23.8% (37,38). As for manual segmentation, Song et al. revealed that the limits of agreement were –27.3% to 29.5% for volume measurements (32). Nevertheless, the current study did consider intra-observer variation, and the real change was defined as a change in volume of at least 25% between the first and second scans, which was also applied in the NELSON trial (7). Finally, review of CT scans and segmentation by a single physician are not sufficient. To decrease artefactual errors, more than one physician is always preferred.
In conclusion, for pulmonary nodules in routine clinical practice, compared with benign nodules, much more malignant nodules grew over time, which was true in stratified analysis regarding nodule texture and follow-up duration. Nevertheless, there was a limitation in the differential diagnosis of growing nodules by VDT because benign nodules could also rapidly grow. Furthermore, although some nodules were malignant, many grew slowly or remained stable over time.
Acknowledgments
Funding: This work was supported by the National Key Development Plan for Precision Medicine Research (2017YFC0910004), and the National Science Foundation of China (81700095).
Footnote
Conflicts of Interest: All authors have completed the ICMJE uniform disclosure form (available at http://dx.doi.org/10.21037/jtd-19-3591). The authors have no conflicts of interest to declare.
Ethical Statement: The authors are accountable for all aspects of the work in ensuring that questions related to the accuracy or integrity of any part of the work are appropriately investigated and resolved. The institutional review board of West China Hospital approved this retrospective study (No. ChiCTR1800015700), with a waiver of the requirement for patient informed consent.
Open Access Statement: This is an Open Access article distributed in accordance with the Creative Commons Attribution-NonCommercial-NoDerivs 4.0 International License (CC BY-NC-ND 4.0), which permits the non-commercial replication and distribution of the article with the strict proviso that no changes or edits are made and the original work is properly cited (including links to both the formal publication through the relevant DOI and the license). See: https://creativecommons.org/licenses/by-nc-nd/4.0/.
References
- Bray F, Ferlay J, Soerjomataram I, et al. Global cancer statistics 2018: GLOBOCAN estimates of incidence and mortality worldwide for 36 cancers in 185 countries. CA Cancer J Clin 2018;68:394-424. [Crossref] [PubMed]
- NIH. Cancer Stat Facts: Lung and Bronchus Cancer. Available online: https://seer.cancer.gov/statfacts/html/lungb.html
- Becker N, Motsch E, Gross ML, et al. Randomized study on early detection of lung cancer with MSCT in Germany: results of the first 3 years of follow-up after randomization. J Thorac Oncol 2015;10:890-6. [Crossref] [PubMed]
- Infante M, Cavuto S, Lutman FR, et al. Long-term follow-up results of the DANTE trial, a randomized study of lung cancer screening with spiral computed tomography. Am J Respir Crit Care Med 2015;191:1166-75. [Crossref] [PubMed]
- National Lung Screening Trial Research Team, Aberle DR, Adams AM, et al. Reduced lung-cancer mortality with low-dose computed tomographic screening. N Engl J Med 2011;365:395-409. [Crossref] [PubMed]
- Paci E, Puliti D, Lopes Pegna A, et al. Mortality, survival and incidence rates in the ITALUNG randomised lung cancer screening trial. Thorax 2017;72:825-31. [Crossref] [PubMed]
- van Klaveren RJ, Oudkerk M, Prokop M, et al. Management of lung nodules detected by volume CT scanning. N Engl J Med 2009;361:2221-9. [Crossref] [PubMed]
- Wille MM, Dirksen A, Ashraf H, et al. Results of the randomized danish lung cancer screening trial with focus on high-risk profiling. Am J Respir Crit Care Med 2016;193:542-51. [Crossref] [PubMed]
- Zhao SJ, Wu N. Early detection of lung cancer: low-dose computed tomography screening in China. Thorac Cancer 2015;6:385-9. [Crossref] [PubMed]
- Yang W, Qian F, Teng J, et al. Community-based lung cancer screening with low-dose CT in China: results of the baseline screening. Lung Cancer 2018;117:20-6. [Crossref] [PubMed]
- Henschke CI, Salvatore M, Cham M, et al. Baseline and annual repeat rounds of screening: implications for optimal regimens of screening. Eur Radiol 2018;28:1085-94. [Crossref] [PubMed]
- Anderson IJ, Davis AM. Incidental pulmonary nodules detected on CT images. JAMA 2018;320:2260-1. [Crossref] [PubMed]
- Mohamed Hoesein FA, de Jong PA, Mets OM. Optimizing lung cancer screening: nodule size, volume doubling time, morphology and evaluation of other diseases. Ann Transl Med 2015;3:19. [PubMed]
- Baldwin DR, Callister ME. Guideline Development Group. The British Thoracic Society guidelines on the investigation and management of pulmonary nodules. Thorax 2015;70:794-8. [Crossref] [PubMed]
- Gould MK, Donington J, Lynch WR, et al. Evaluation of individuals with pulmonary nodules: when is it lung cancer? Diagnosis and management of lung cancer, 3rd ed: American College of Chest Physicians evidence-based clinical practice guidelines. Chest 2013;143:e93S-120S.
- Bai C, Choi CM, Chu CM, et al. Evaluation of pulmonary nodules: clinical practice consensus guidelines for Asia. Chest 2016;150:877-93. [Crossref] [PubMed]
- ACR. Lung CT Screening Reporting & Data System. Available online: https://www.acr.org/Clinical-Resources/Reporting-and-Data-Systems/Lung-Rads
- NCCN Guidelines for Lung Cancer Screening, Version 2.2019. Available online: https://www.nccn.org/
- Mets OM, Chung K, Zanen P, et al. In vivo growth of 60 non-screening detected lung cancers: a computed tomography study. Eur Respir J 2018. [Crossref] [PubMed]
- Heuvelmans MA, Vliegenthart R, de Koning HJ, et al. Quantification of growth patterns of screen-detected lung cancers: the NELSON study. Lung Cancer 2017;108:48-54. [Crossref] [PubMed]
- Horeweg N, van Rosmalen J, Heuvelmans MA, et al. Lung cancer probability in patients with CT-detected pulmonary nodules: a prespecified analysis of data from the NELSON trial of low-dose CT screening. Lancet Oncol 2014;15:1332-41. [Crossref] [PubMed]
- Xu DM, van der Zaag-Loonen HJ, Oudkerk M, et al. Smooth or attached solid indeterminate nodules detected at baseline CT screening in the NELSON study: cancer risk during 1 year of follow-up. Radiology 2009;250:264-72. [Crossref] [PubMed]
- Schwartz M. A biomathematical approach to clinical tumor growth. Cancer 1961;14:1272-94. [Crossref] [PubMed]
- Walter JE, Heuvelmans MA, Ten Haaf K, et al. Persisting new nodules in incidence rounds of the NELSON CT lung cancer screening study. Thorax 2019;74:247-53. [Crossref] [PubMed]
- Ashraf H, Dirksen A, Loft A, et al. Combined use of positron emission tomography and volume doubling time in lung cancer screening with low-dose CT scanning. Thorax 2011;66:315-9. [Crossref] [PubMed]
- de Hoop B, van Ginneken B, Gietema H, et al. Pulmonary perifissural nodules on CT scans: rapid growth is not a predictor of malignancy. Radiology 2012;265:611-6. [Crossref] [PubMed]
- Sone S, Hanaoka T, Ogata H, et al. Small peripheral lung carcinomas with five-year post-surgical follow-up: assessment by semi-automated volumetric measurement of tumour size, CT value and growth rate on TSCT. Eur Radiol 2012;22:104-19. [Crossref] [PubMed]
- Henschke CI, Yankelevitz DF, Yip R, et al. Lung cancers diagnosed at annual CT screening: volume doubling times. Radiology 2012;263:578-83. [Crossref] [PubMed]
- Obayashi K, Shimizu K, Nakazawa S, et al. The impact of histology and ground-glass opacity component on volume doubling time in primary lung cancer. J Thorac Dis 2018;10:5428-34. [Crossref] [PubMed]
- Austin JH, Garg K, Aberle D, et al. Radiologic implications of the 2011 classification of adenocarcinoma of the lung. Radiology 2013;266:62-71. [Crossref] [PubMed]
- Lederlin M, Puderbach M, Muley T, et al. Correlation of radio- and histomorphological pattern of pulmonary adenocarcinoma. Eur Respir J 2013;41:943-51. [Crossref] [PubMed]
- Song YS, Park CM, Park SJ, et al. Volume and mass doubling times of persistent pulmonary subsolid nodules detected in patients without known malignancy. Radiology 2014;273:276-84. [Crossref] [PubMed]
- Hawkins S, Wang H, Liu Y, et al. Predicting malignant nodules from screening CT scans. J Thorac Oncol 2016;11:2120-8. [Crossref] [PubMed]
- Cherezov D, Hawkins SH, Goldgof DB, et al. Delta radiomic features improve prediction for lung cancer incidence: a nested case-control analysis of the National Lung Screening Trial. Cancer Med 2018;7:6340-56. [Crossref] [PubMed]
- Ardila D, Kiraly AP, Bharadwaj S, et al. End-to-end lung cancer screening with three-dimensional deep learning on low-dose chest computed tomography. Nat Med 2019;25:954-61. [Crossref] [PubMed]
- Lu H, Mu W, Balagurunathan Y, et al. Multi-window CT based radiomic signatures in differentiating indolent versus aggressive lung cancers in the National Lung Screening Trial: a retrospective study. Cancer Imaging 2019;19:45. [Crossref] [PubMed]
- Wormanns D, Kohl G, Klotz E, et al. Volumetric measurements of pulmonary nodules at multi-row detector CT: in vivo reproducibility. Eur Radiol 2004;14:86-92. [Crossref] [PubMed]
- Gietema HA, Schaefer-Prokop CM, Mali WP, et al. Pulmonary nodules: Interscan variability of semiautomated volume measurements with multisection CT-- influence of inspiration level, nodule size, and segmentation performance. Radiology 2007;245:888-94. [Crossref] [PubMed]