Path to precision: prevention of post-operative atrial fibrillation
Introduction
Post-operative atrial fibrillation (POAF) continues to be a devastating complication following cardiac surgery, affecting 25–40% coronary artery bypass graft (CABG) (1,2) and 30–40% valve patients (3). Although POAF is thought to be transient in nature, peaking on post-operative days 2–4 and resolving within 4–6 weeks (4), there is mounting evidence to suggest POAF increases risk of stroke, respiratory failure, pneumonia, morbidity and mortality, longer intensive care unit and hospital stays, and increased resource use (4-13).
Recent advancements and current research indicate POAF is in part preventable (4); however, progress has been hindered by scarce, conflicting data, and lack of knowledge on independent predictors, effective interventions, and a consistent, all-encompassing clinical and biochemical database. Currently, the use of statistical analysis is common to assess an individual’s risk of developing POAF. However, this method limits plasticity and fails to account for ongoing changes in clinical and functional indices during peri-operative stay. Despite continuing growth in POAF literature, POAF incidence has not changed over the past three decades (12). This poses the question—can we predict and detect POAF more effectively? That is, can we stack patient factors and comorbidities with real-time, continuous data from multimodal streams to better predict, diagnose, and manage patients for improving personalized care?
Whether utilizing advanced neural networks (NN) such as convolutional NN to diagnose skin lesions or automate the classification of radiological reports for clinical care, machine learning (ML) has demonstrated superiority to traditional statistical methods for predictive scoring and diagnosis. Similarly, within the cardiovascular arena, several strategies to identify and detect arrhythmias by constructing a digital atlas of the atria and classifying rhythms from ECG and Holter monitors have been demonstrated over the past two decades (14). Nevertheless, despite defining independent risk predictors and addressing differences in clinical outcomes, heterogeneous data from multiple studies and lack of interoperability among medical devices limit progress in this field. Developing an ecosystem with crosstalk capabilities coupled with building data repositories can expatiate on the underlying mechanisms of POAF. In this review, we will appraise the current literature and evaluate the future direction of risk prediction models for POAF using machine and deep learning.
POAF
Definition and diagnosis
AF following surgery is described as POAF, but the pathogenesis and trigger differentiate it from regular AF. At present, there is a lack of consensus on the definition of POAF. The Society of Thoracic Surgeons (STS) describes it as AF post-surgery requiring treatment while Heart Rhythm Society for clinical diagnosis specifies it as POAF treatment with rate or rhythm control agents, anticoagulation, and or extending hospital stay (4,15). Further, American Association for Thoracic Surgery (AATS) signify POAF as a class 1 diagnosis recommendation with ECG features lasting at least 30 seconds or for the duration of the ECG recording if less than 30 seconds (4). As Hui et al. highlight, the STS definition underestimates nearly 10,000 patients annually who experienced AF that terminated prior to treatment or had contraindications to therapy (15). This thereby neglects increased 30-day mortality risk and warrants a refined definition that not only accounts for continuous monitoring but also is not limited by physician documentation alone.
Pathophysiology
Probable mechanisms contributing to POAF often require a trigger and a vulnerable atrial substrate. However, of note, the mechanism remains elusive (4). Although increasing age seems to be the best-appreciated risk factor, several studies suggest patients with hypertension, myocardial infarction (MI), valvular heart disease, heart failure, obesity, atrial fibrosis or dilation, male sex, and history of arrhythmias have greater propensity to develop POAF (4). Increase in adrenergic drive and change in vagal tone can affect heart rate and shorten atrial wavelength, thereby potentially triggering POAF. Moreover, the local and systemic inflammation from cardiac procedures augments vulnerability of the atrial substrate, which is further supported by a reduction in POAF incidence with off-pump CABG surgeries and decreased duration of cardiopulmonary bypass. Following surgery, POAF develops within the first few days and often returns to sinus rhythm, which is distinctive from other forms of AF, suggesting a different pathophysiology. Through retrieval of retrospective data from health records and monitoring devices, predictable risk factors and prophylactic therapies can be uncovered.
Prevention strategies
EuroSCORE (16) and STS risk models (17) have been utilized for decades to risk stratify cardiac patients. These risk calculators identify the patient’s chance of mortality, renal failure, permanent stroke, prolonged ventilation, infection, length of stay, and reoperation (17). Presently, there is no current standard model to predict the risk of POAF. However, previously cited risk factors help speculate a patient’s risk of POAF and stroke (4). CHA2DS2VASc score is currently used to determine the one-year risk of thromboembolic events in a non-anticoagulated patient with non-valvular AF and indicate a sense of a patient’s long-term stroke risk; the risk factors include congestive heart failure, hypertension, age, diabetes, stroke, vascular disease, and female sex (18). Pre-operative assessment for cardiac surgery routinely includes complete blood count, comprehensive metabolic panel, coagulation studies, blood type and screen, dental screen, pulmonary function test, 12-lead ECG, chest radiograph, carotid ultrasound study, ankle-brachial index, vein mapping, computed tomography scan, and left heart catheterization. These diagnostic studies are weighed with screening questionnaires for functional status and peri-operative risk during pre-operative screening. Further workup is dictated by the patient’s pathology and medical history.
A common practice at most hospitals is continuous physiological (e.g., ECG) monitoring for 48–72 hours post-operatively, which is recommended for patients who exhibit clinical signs or who undergo procedures that pose intermediate to high POAF risk. Efficacy of several agents has been tested in randomized clinical trials (19-32) or suggested through meta-analyses (33-39). AATS recommends that all patients should continue beta-blockers to avoid withdrawal and prevent POAF (class I, LOE A recommendation) (4,33,40). However, based on AATS surveys, this strategy is currently underused. Post-operative beta blocker use has demonstrated a reduction in POAF with high heterogeneity in several studies and was found to be inferior to amiodarone therapy (41,42). Moreover, intravenous (IV) magnesium supplementation may be considered for patients with low total body or serum magnesium level (class IIb) (4,43).
For intermediate to high-risk patients, post-operative administration of diltiazem to patients with preserved cardiac function who are not taking beta-blockers pre-operatively (class IIa, LOE B) or amiodarone IV (class IIa, LOE A) can reduce the incidence of POAF (4,40). Intra-operative left atrial appendage ligation has also been demonstrated as a preventative strategy for patients who are not candidates for anticoagulation (class IIb, LOE C) (4,44). Based on reports from meta-analyses and trials, other therapies such as pre-operative statins (45), n-3 polyunsaturated fatty acids (46,47), colchicine (28), corticosteroids (48), and posterior pericardiectomy (49) have failed to validate efficacy (41).
In Figure 1, we summarize the risk factors, pathogenesis, and prevention recommendations of POAF.
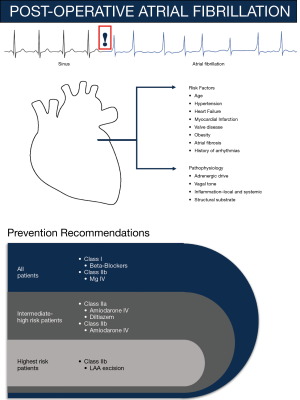
Management strategies
Despite current best practices to prevent POAF, POAF continues to develop in 20–50% of cardiac patients. Management strategies for all patients involve minimizing inotropic agents, optimize fluid status, and correct electrolyte or other metabolic imbalances. Further treatment is guided by the patient’s hemodynamic stability for cardioversion or pharmaceutical therapy and duration of new-onset POAF for rate control or anticoagulation management. Gillinov and colleagues showed that short-term mortality was comparable between rate and rhythm controlled-strategies (50).
Potential data sources
In this section, potential data sources to predict POAF are described.
Electronic health records (EHR)
The EHR maintains an electronic version of a patient medical history including demographics, progress notes, complications, medications, vital signs summary, past medical history, immunizations, laboratory data, and imaging reports. EHR is a common data source for detecting clinical risks and outcomes.
With the help of EHR, the STS National Database, established in 1989, stores over ten years of data from cardiothoracic patients nationwide. Initially started as an initiative for quality improvement and patient safety, the database with over 1,000 variables collected from each patient has now become a treasure chest for researchers and physicians to mitigate surgical complications. While the STS database is a proven, invaluable tool to identify a handful of independent predictors such as age and other cardiac comorbidities, research progress is limited by the vast amount of human capital, resources, and exorbitant costs required to access and handle such data. This along with the possibility of inaccuracy restricts the scope of application. Nevertheless, automating data input into an algorithmic model can expedite real-time evaluation and treatment while limiting erroneous data. Deployment of ML with longitudinal data from EHR has demonstrated feasibility and value in predicting cardiovascular events (51).
Conventional imaging modalities including echocardiography, computed tomography (CT), and magnetic resonance imaging (MRI) from EHR and imaging software can be used to abstract, quantify, and measure tissue matter. This has been previously employed and revealed the association of pericardial fat content with AF (52,53). Similarly, evaluation of the left atrial appendage structure and finding of left atrial thrombus using echocardiography was observed to be a predictor in non-valvular AF (54). Development of a fully automated algorithm for segmenting from imaging modalities to quantify body composition can meet or exceed the accuracy of manual segmentation.
Vital signs
Industry-leading clinical monitoring equipment measures high-fidelity vital signs such as ECG, blood pressure, pulse, oxygen saturation data from bedside monitors and offer customization and assessment entries into the system. These data can be also reviewed at the point of care and incremental data linked to the EHR. Continuous capture of high-fidelity time-stamped data exported to the algorithm model can be assimilated for further accuracy and prediction.
Electrocardiogram and telemetry
For over half a century, the 12-lead electrocardiogram (ECG) has served as the gold standard for arrhythmia detection and screening. Continuous surveillance for bedside and ambulatory cardiac patients with telemetry data and temperature, pressure, and anesthetic gas monitoring capabilities allows arrhythmia analysis, alarm notifications, and event-based examination. Raw and processed data isolating ECG intervals and segments from the device technology can augment predictive models to alert medical providers and patients if POAF is imminent (55). In addition to the traditional 12-lead ECG and continuous surveillance of heart rate and irregular RR intervals to screen for AF, Nihon Kohdens’ 510K cleared AF alarm performs AF detection by using both RR intervals and P waves as input. More precisely, the algorithm uses three features of input ECG to output AF detection result-RR irregularity, PR interval variability, and P wave variability (56). AF lasting at least two minutes is identified and is notified every time it is detected. In addition, an AF end alarm allows the provider to recognize and measure the AF burden per patient. Similar methodologies are applied for Philips and GE Healthcare monitoring systems (57,58).
Holter monitors
Holter monitors can capture ambulatory ECG tracings for 24–48 hours or longer to detect paroxysmal or sub-clinical AF in an outpatient setting. Other archives of ECG data from Holter-like monitors such as from implantable cardiac monitors (59) can further enhance diagnostics and lead to innovative solutions such as online remote monitoring system (60).
Wearable devices
Comparable undertakings have been employed to translate and compare electrical signals from ECG and Holter with photoplethysmography (PPG) from wearable devices.
Similar to background noise signals from ECG and Holter data, PPG signals are limited by implemented sensing setup, probe attachment site and contact pressure, subject movement and posture, poor blood perfusion, ambient light, and environmental temperature (61). Many technology companies, such as iRhythm, Apple, Philips, and AliveCor have invested heavily in healthcare analytics to improve validation systems and provide low-cost wearable technology to facilitate precision medicine (62). Use of smart watches and other wrist devices for pulse monitoring has enabled large-scale screening for undiagnosed arrhythmias such as the Apple Heart study (63). Turakhia et al. conducted a clinical trial to identify the incidence of pulse irregularity in AF patients using the Apple Watch (Apple Inc, Cupertino, CA, USA) and evaluate the accuracy of episodes based on simultaneous recorded ambulatory ECG (63). Findings suggest that pulse irregularity was noted in 0.52% of the participants and was most frequent in those over age 65. Of those who received and wore the ECG patch for monitoring, 34% were identified to have AF (64). As Apple unveils the new features of the Apple watch, further stacked ECG and PPG data will reveal advances and limitations to detect AF, especially at home. Other commercially available smartwatches and devices such as the Polar watch, KardiaBand, and KardiaMobile have demonstrated benefit with variable discriminative ability (65). POAF has been shown to occur even after 30 days following surgery; therefore, wearable devices can allow for detecting arrhythmias. Currently, Ai-Cor (66), an app utilizing continuous Apple watch monitoring, strives to predict AF based on HRV changes. Full integration with Apple Health kit, provision to add medication lists, symptoms, and daily activity, and interaction with Ai-Me, the augmented human intelligence bot, promotes a promising tool for personalized medicine (66).
Omics data
‘Omics refers to big data from biology fields such as genomics, metabolomics, and proteomics that collectively characterize the biological modifications and complex interactions in cell metabolism. Biospecimens collected from patients are processed using high-throughput instruments routinely used in laboratories to generate complex datasets. Multi-layered heterogeneous biological data are then analyzed using various software platforms for data integration. These biochemical networks allow visualization of biological pathways to elucidate further mechanistic processes.
Identification of candidate genes, pathways, and biological responses can reveal predictive biomarkers, deliver novel insights of disease progression, and uncover disease subtyping. As the genesis of POAF is due to an operative trigger and a vulnerable atrial substrate, characterization of the atrial substrate and plasma content from training datasets can support predictive models. Exhaustive examination of discrete components of the POAF temporal phenotype through deep phenotyping can enhance specificity, increase big data yield with high resolution, and bolster disease subtype and genetic variation networks. Currently, the development of open-access computational resources has cultivated further advancements in discovery and understanding of biological systems. This will allow for prediction and actionable insights.
Lifestyle questionnaires
As day-to-day lifestyle behaviors and mental health influence clinical outcomes, it is imperative to evaluate and measure the burden of lifestyle and environmental factors. Although these surveys are provided for patients, the data is often assessed for real-time diagnostics and are seldom stored in EHR. This limits longitudinal capture and measurement of progress. Today, mobile apps to track physical activity, mindfulness, nutrition, and sleep are well utilized. However, we have not exhausted the potential of this massive reservoir of data. Addition of this data to other physiological data can have an immense impact on diagnosis and treatment.
ML for POAF prevention
AF detection
ML algorithms, including traditional ML, deep learning, and their combination, are widely used for automatic arrhythmia detection (67), in both short and long-term ECG analysis. With the popularity of deep learning approaches, several studies have used convolutional neural networks (CNN) and recurrent neural networks (RNN) and categorized ECG signals as sinus rhythm and AF. For example, Acharya et al. were successfully able to classify AF from a 5-second ECG signal at 94.90% accuracy and 99.13% sensitivity. While several others have employed other models to attain higher sensitivity and specificity, for example by using the Jiang method, many require longer ECG signals, which may cause shorter-lived arrhythmias to go unnoticed (68). Endeavors to detect and mitigate this pervasive disease were further incentivized by the PhysioNet challenge conducted in 2017. AI engineers from across the world competed to create AF algorithms from ECG recordings. The highest score was submitted by Teijeiro et al. (69) with a score of 0.85, in which the team used a combined algorithm of XGBoost, RNN, and LDA-Stacker, while other algorithms proposed by Zihlmann (70), Vollmer (71), Rubin (72), Stepien (73), Schwab (74), and Andreotti (75) scored 0.82, 0.81, 0.80, 0.79, 0.79, 0.79 respectively. Results of Physionet/Computing in Cardiology Challenge 2017 on AF detection (76) demonstrates that an automatic detection of AF versus normal sinus rhythm (NSR), other rhythm (O), and noise using ECG is possible. Furthermore, the results of this challenge show that there is no superior algorithm for classification of NSR/AF/O/noise (77) and suggests that a combination of different algorithms through voting can be beneficial (76). Of note, the performance of AF detection algorithms could potentially be improved by the advancement of ML techniques and the availability of larger datasets.
While many describe noise contamination as a limitation, the variability in methodologies and variances in predictability urges the continued use of ECG as a biomarker for AF detection. Nonetheless, noise contamination will need to be addressed to detect POAF, especially in the post-cardiac surgery hospital setting where patients are monitored via telemetry.
Learned model parameters based on ECG and Holter data were tested on PPG data using cross-domain generalizability to assess superiority and improve AF detection performance (78). Gotlibovych and colleagues inputted raw PPG signal data to develop a neural-network-based algorithm by extracting a range of features describing variability in periods of amplitudes as well and morphology of individual heartbeats (79). Results from the study achieved a 0.998 and 0.999 specificity and sensitivity, respectively (79). Deep learning algorithms such as CNN are also used for AF detection captured by a wrist-worn PPG (78,80). PPG data captured by wrist-worn devices (e.g., Fitbit and Apple watch) can be further improved for AF detection.
AF prediction
ECG could be used to predict AF and ischemic stroke. For example, Johnson et al. (81) demonstrate that short irregular supraventricular tachycardia without p-waves are associated with incident AF and ischemic stroke. Furthermore, Boon et al. used heart rate variability (HRV) and support vector ML to predict AF (82) with an accuracy rate of 87.7%. Despite research studies in this area, the application of ML for prediction of AF and its complications especially by using biomarkers (e.g., ECG) needs further exploration.
Addition of complementary information from other data sources explained in Section 3 to the ML model created using vital signs (e.g., ECG and PPG) could potentially improve AF detection and prediction. Furthermore, improving the interpretability of deep learning models can facilitate knowledge discovery (e.g., identification of new digital biomarkers) especially after presenting more interpretable results to domain experts to get their insight.
Natural language processing and image processing
Utilization of natural language processing for further analysis of available notes in EHR (e.g., physician notes and radiology reports), image processing, and other ML approaches such as CNN from CT, MRI, and echocardiograms can allow ML to extract millions of data points for risk analysis. Pre-, intra-, and post-operative information from EHRs can be readily available including past medical diagnoses, continuous telemetry data, labs, hemodynamics, and outcome measures. Advances in ML can help reduce the need for routine surveillance by consolidating the information from EHRs. Data can be captured, classified, and scored to predict POAF and improve patient healthcare.
ML for omic data
Data integration from RNAseq, proteomics, and metabolomics involves multiple steps. Raw paired-end reads obtained from high throughput RNA sequencing are assembled after quality trimming. Reads are then aligned to the human genome. Feature-counts allow efficient chromosome hashing and feature blocking techniques to generate a gene counts table. Differential expression analysis is performed based on gene-wise dispersion estimates and fold changes to improve stability and to focus the strength of the estimates. Pathway analysis and gene set enrichment analysis tools support visualization of downstream effects and association of pathways. Proteomic analyses can be performed by one-dimensional gel electrophoresis followed by high-performance liquid chromatography-electrospray ionization tandem mass spectrometry. Bioinformatics is then used to determined protein abundance. Similarly, metabolomic raw data from ultra-performance liquid chromatography– tandem mass spectroscopy can be extracted for peak-identification and quality control processing. Once peaks are quantified, and data are compared to library entries of purified standards, visualization of curated data can elucidate significantly altered biochemicals.
Pipeline tools for data integration from high throughput RNA sequencing, proteomics, and metabolomics allow matching individual omic data matrices and for easier experimentation and reproducibility. Autoencoder-based deep learning, integrative clustering analysis, and principal component analysis can be incorporated to evaluate the performance of integration approaches. This allows classification labeling and evaluation of association to patients’ prognosis. Supervised models with feature combination with the highest predictive accuracy offer prediction of groups (POAF versus no POAF) for internal and external validation sets.
Proposed methodology
Current prognostic practices for many diseases and complications undervalue the significance of multimodal data streams. Use of ML practices to identify drivers during pre-, intra-, and post-operation can boost prediction accuracy for POAF. The following propositions may be prized to create a centralized approach for prognostics in clinical practice, as illustrated in Figures 2 and 3.
During pre-operative management, patients’ medical history, radiology imaging, baseline labs, and omic data can be stored and streamlined into a HIPAA-compliant server that allows for data abstraction and sorting. Multi-stacked, labeled data can then be used to define a personalized, predictive score that comprises the patient’s risk of POAF, recovery, morbidity, and mortality. Based on the initial score, therapy and treatment timeline can be tailored accordingly, and modified clinical management can alleviate the economic burden. Additional intra-operative data such as continuous vital signs, telemetry, hemodynamics, and pre- and post-cardiac bypass omic data can reassess the patient’s risks and progression. As patients continue to be monitored during their post-operative stay, changes in the patient’s telemetry and physiological state can be evaluated with real-time predictive tools to guide therapy. Post-operative complications secondary to POAF such as stroke can be further averted through careful, prognostic management. If a clinical alert requires intervention, treatment alterations can be inputted to modify predictive analysis and modify future clinical course. This continuous revision of clinical indices can catalyze prospective mechanistic and therapeutic advances.
Cost analysis
Predictive models to mitigate the POAF burden can also effectively govern cost management. Recognizing comorbidities and its impact on the overall cost of cardiac surgery and POAF complication is critical in optimizing expenses. POAF accumulates an additional $10,000–20,000 hospital costs per patient (4,5). This compounded with follow-up, potentially lifetime oral anticoagulant treatment of at least $23,000 (83), amasses to a large economic burden. A recent study by Atreya et al. (84) demonstrated peri-operative amiodarone treatment in cardiac surgery patients decrease atrial arrhythmias including AF; however, a higher incidence of ventricular arrhythmias and an increase of $1,866 in cost were observed. With over 150,000 CABG surgeries (85) performed each year, averaging $151,271 and ranging from $44,824–$448,039 (86), coupling the proposed methodology with outcome measurement and cost analysis can identify and tighten major cost drivers. Moreover, this can guide novel diagnostic and therapeutic strategies as well as promote healthcare policy changes. Through transparency in healthcare prices and hospital quality outcomes, policies can be shifted to a more value-based rather than fee-based model to improve patient care while reducing costs.
Discussion
POAF has continued to be the most common complication seen in cardiac surgical patients, and an aging population, a major factor of POAF, compounds the increased prevalence. Prediction and prevention of POAF can help alleviate both the clinical burden of stroke and morbidities as well as the economic burden from increased length of stay and treatment management. Furthermore, despite continued research and formation of taskforces, advancements have been limited by a lack of integration of heterogeneous big data.
To address these gaps in knowledge, the development of an effective and robust platform that utilizes ML state-of-the-art computational tools and statistical models can accelerate understanding of divergent POAF mechanistic hypotheses, help define novel functional POAF phenotypes, and guide medical therapy. Integration of EHR data with continuous real-time data from medical devices and wearables, along with imaging and omic big data can transform our understanding of this disease as well as translate such models to other medical illnesses. While this will require crosstalk features between devices and EHRs and therefore time and capital, we trust our review of current developments and enormous potential of such capabilities will inspire, engage, and unite multidisciplinary teams to translate into practice.
Acknowledgments
Funding: None.
Footnote
Conflicts of Interest: All authors have completed the ICMJE uniform disclosure form (available at http://dx.doi.org/10.21037/jtd-19-3875). RS previously completed an internship at Philips Research North America and reports other from null, outside the submitted work; SP works at Philips Research North America and reports personal fees from Philips Research North America, outside the submitted work; SZ works at Philips Research North America and reports personal fees from null, outside the submitted work; GD works for Nihon Kohden America and reports other from null, outside the submitted work; ZK’s spouse is a member of Ai-Cor and reports other from null, outside the submitted work. The other authors have no conflicts of interest to declare.
Ethical Statement: The authors are accountable for all aspects of the work in ensuring that questions related to the accuracy or integrity of any part of the work are appropriately investigated and resolved.
Open Access Statement: This is an Open Access article distributed in accordance with the Creative Commons Attribution-NonCommercial-NoDerivs 4.0 International License (CC BY-NC-ND 4.0), which permits the non-commercial replication and distribution of the article with the strict proviso that no changes or edits are made and the original work is properly cited (including links to both the formal publication through the relevant DOI and the license). See: https://creativecommons.org/licenses/by-nc-nd/4.0/.
References
- Kaw R, Hernandez AV, Masood I, et al. Short- and long-term mortality associated with new-onset atrial fibrillation after coronary artery bypass grafting: a systematic review and meta-analysis. J Thorac Cardiovasc Surg 2011;141:1305-12. [Crossref] [PubMed]
- Cox JL. A perspective of postoperative atrial fibrillation in cardiac operations. Ann Thorac Surg 1993;56:405-9. [Crossref] [PubMed]
- Omae T, Kanmura Y. Management of postoperative atrial fibrillation. J Anesth 2012;26:429-37. [Crossref] [PubMed]
- Frendl G, Sodickson AC, Chung MK, et al. 2014 AATS guidelines for the prevention and management of perioperative atrial fibrillation and flutter for thoracic surgical procedures. J Thorac Cardiovasc Surg 2014;148:e153-93. [Crossref] [PubMed]
- Mathew JP, Parks R, Savino JS, et al. Atrial fibrillation following coronary artery bypass graft surgery: predictors, outcomes, and resource utilization. MultiCenter Study of Perioperative Ischemia Research Group. JAMA 1996;276:300-6. [Crossref] [PubMed]
- Attaran S, Shaw M, Bond L, et al. Atrial fibrillation postcardiac surgery: a common but a morbid complication. Interact Cardiovasc Thorac Surg 2011;12:772-7. [Crossref] [PubMed]
- Al-Khatib SM, Arshad A, Balk EM, et al. Risk Stratification for Arrhythmic Events in Patients With Asymptomatic Pre-Excitation: A Systematic Review for the 2015 ACC/AHA/HRS Guideline for the Management of Adult Patients With Supraventricular Tachycardia: A Report of the American College of Cardiology/American Heart Association Task Force on Clinical Practice Guidelines and the Heart Rhythm Society. J Am Coll Cardiol 2016;67:1624-38. [Crossref] [PubMed]
- Yadava M, Hughey AB, Crawford TC. Postoperative Atrial Fibrillation: Incidence, Mechanisms, and Clinical Correlates. Heart Fail Clin 2016;12:299-308. [Crossref] [PubMed]
- Zakkar M, Ascione R, James AF, et al. Inflammation, oxidative stress and postoperative atrial fibrillation in cardiac surgery. Pharmacol Ther 2015;154:13-20. [Crossref] [PubMed]
- Villareal RP, Hariharan R, Liu BC, et al. Postoperative atrial fibrillation and mortality after coronary artery bypass surgery. J Am Coll Cardiol 2004;43:742-8. [Crossref] [PubMed]
- Shen J, Lall S, Zheng V, et al. The persistent problem of new-onset postoperative atrial fibrillation: a single-institution experience over two decades. J Thorac Cardiovasc Surg 2011;141:559-70. [Crossref] [PubMed]
- Greenberg JW, Lancaster TS, Schuessler RB, et al. Postoperative atrial fibrillation following cardiac surgery: a persistent complication. Eur J Cardiothorac Surg 2017;52:665-72. [Crossref] [PubMed]
- Phan K, Ha HS, Phan S, et al. New-onset atrial fibrillation following coronary bypass surgery predicts long-term mortality: a systematic review and meta-analysis. Eur J Cardiothorac Surg 2015;48:817-24. [Crossref] [PubMed]
- Hannun AY, Rajpurkar P, Haghpanahi M, et al. Cardiologist-level arrhythmia detection and classification in ambulatory electrocardiograms using a deep neural network. Nat Med 2019;25:65-9. [Crossref] [PubMed]
- Hui DS, Lee R. Do we need yet another way to classify atrial fibrillation? J Thorac Cardiovasc Surg 2017;154:1267-8. [Crossref] [PubMed]
- EuroSCORE Interactive Calculator. 2011. Available online: . 2019.http://www.euroscore.org/calc.html
- Online STS Risk Calculator. 2017.
- Lip GY, Nieuwlaat R, Pisters R, et al. Refining clinical risk stratification for predicting stroke and thromboembolism in atrial fibrillation using a novel risk factor-based approach: the euro heart survey on atrial fibrillation. Chest 2010;137:263-72. [Crossref] [PubMed]
- Amar D, Roistacher N, Rusch VW, et al. Effects of diltiazem prophylaxis on the incidence and clinical outcome of atrial arrhythmias after thoracic surgery. J Thorac Cardiovasc Surg 2000;120:790-8. [Crossref] [PubMed]
- Haghjoo M, Saravi M, Hashemi MJ, et al. Optimal beta-blocker for prevention of atrial fibrillation after on-pump coronary artery bypass graft surgery: carvedilol versus metoprolol. Heart Rhythm 2007;4:1170-4. [Crossref] [PubMed]
- Halonen J, Hakala T, Auvinen T, et al. Intravenous administration of metoprolol is more effective than oral administration in the prevention of atrial fibrillation after cardiac surgery. Circulation 2006;114:I1-4. [Crossref] [PubMed]
- Mitchell LB, Exner DV, Wyse DG, et al. Prophylactic Oral Amiodarone for the Prevention of Arrhythmias that Begin Early After Revascularization, Valve Replacement, or Repair: PAPABEAR: a randomized controlled trial. JAMA 2005;294:3093-100. [Crossref] [PubMed]
- White CM, Caron MF, Kalus JS, et al. Intravenous plus oral amiodarone, atrial septal pacing, or both strategies to prevent post-cardiothoracic surgery atrial fibrillation: the Atrial Fibrillation Suppression Trial II (AFIST II). Circulation 2003;108 Suppl 1:II200-6. [Crossref] [PubMed]
- Gomes JA, Ip J, Santoni-Rugiu F, et al. Oral d,l sotalol reduces the incidence of postoperative atrial fibrillation in coronary artery bypass surgery patients: a randomized, double-blind, placebo-controlled study. J Am Coll Cardiol 1999;34:334-9. [Crossref] [PubMed]
- Serafimovski N, Burke P, Khawaja O, et al. Usefulness of dofetilide for the prevention of atrial tachyarrhythmias (atrial fibrillation or flutter) after coronary artery bypass grafting. Am J Cardiol 2008;101:1574-9. [Crossref] [PubMed]
- Patti G, Chello M, Candura D, et al. Randomized trial of atorvastatin for reduction of postoperative atrial fibrillation in patients undergoing cardiac surgery: results of the ARMYDA-3 (Atorvastatin for Reduction of MYocardial Dysrhythmia After cardiac surgery) study. Circulation 2006;114:1455-61. [Crossref] [PubMed]
- Halonen J, Halonen P, Jarvinen O, et al. Corticosteroids for the prevention of atrial fibrillation after cardiac surgery: a randomized controlled trial. JAMA 2007;297:1562-7. [Crossref] [PubMed]
- Imazio M, Brucato A, Ferrazzi P, et al. Colchicine for prevention of postpericardiotomy syndrome and postoperative atrial fibrillation: the COPPS-2 randomized clinical trial. JAMA 2014;312:1016-23. [Crossref] [PubMed]
- Kingsley JD, Figueroa A. Acute and training effects of resistance exercise on heart rate variability. Clin Physiol Funct Imaging 2016;36:179-87. [Crossref] [PubMed]
- Mozaffarian D, Marchioli R, Macchia A, et al. Fish oil and postoperative atrial fibrillation: the Omega-3 Fatty Acids for Prevention of Post-operative Atrial Fibrillation (OPERA) randomized trial. JAMA 2012;308:2001-11. [Crossref] [PubMed]
- Jakobsen CJ, Bille S, Ahlburg P, et al. Perioperative metoprolol reduces the frequency of atrial fibrillation after thoracotomy for lung resection. J Cardiothorac Vasc Anesth 1997;11:746-51. [Crossref] [PubMed]
- Bayliff CD, Massel DR, Inculet RI, et al. Propranolol for the prevention of postoperative arrhythmias in general thoracic surgery. Ann Thorac Surg 1999;67:182-6. [Crossref] [PubMed]
- Burgess DC, Kilborn MJ, Keech AC. Interventions for prevention of post-operative atrial fibrillation and its complications after cardiac surgery: a meta-analysis. Eur Heart J 2006;27:2846-57. [Crossref] [PubMed]
- Andrews TC, Reimold SC, Berlin JA, et al. Prevention of supraventricular arrhythmias after coronary artery bypass surgery. A meta-analysis of randomized control trials. Circulation 1991;84:III236-44. [PubMed]
- Crystal E, Connolly SJ, Sleik K, et al. Interventions on prevention of postoperative atrial fibrillation in patients undergoing heart surgery: a meta-analysis. Circulation 2002;106:75-80. [Crossref] [PubMed]
- Buckley MS, Nolan PE Jr, Slack MK, et al. Amiodarone prophylaxis for atrial fibrillation after cardiac surgery: meta-analysis of dose response and timing of initiation. Pharmacotherapy 2007;27:360-8. [Crossref] [PubMed]
- Wijeysundera DN, Beattie WS, Rao V, et al. Calcium antagonists reduce cardiovascular complications after cardiac surgery: a meta-analysis. J Am Coll Cardiol 2003;41:1496-505. [Crossref] [PubMed]
- Liakopoulos OJ, Choi YH, Haldenwang PL, et al. Impact of preoperative statin therapy on adverse postoperative outcomes in patients undergoing cardiac surgery: a meta-analysis of over 30,000 patients. Eur Heart J 2008;29:1548-59. [Crossref] [PubMed]
- Miller S, Crystal E, Garfinkle M, et al. Effects of magnesium on atrial fibrillation after cardiac surgery: a meta-analysis. Heart 2005;91:618-23. [Crossref] [PubMed]
- January CT, Wann LS, Alpert JS, et al. 2014 AHA/ACC/HRS guideline for the management of patients with atrial fibrillation: executive summary: a report of the American College of Cardiology/American Heart Association Task Force on practice guidelines and the Heart Rhythm Society. Circulation 2014;130:2071-104. [Crossref] [PubMed]
- Kirchhof P, Benussi S, Kotecha D, et al. 2016 ESC Guidelines for the management of atrial fibrillation developed in collaboration with EACTS. Eur J Cardiothorac Surg 2016;50:e1-88. [Crossref] [PubMed]
- Arsenault KA, Yusuf AM, Crystal E, et al. Interventions for preventing post-operative atrial fibrillation in patients undergoing heart surgery. Cochrane Database Syst Rev 2013;1:CD003611. [PubMed]
- Anderson JL, Halperin JL, Albert NM, et al. Management of patients with atrial fibrillation (compilation of 2006 ACCF/AHA/ESC and 2011 ACCF/AHA/HRS recommendations): a report of the American College of Cardiology/American Heart Association Task Force on Practice Guidelines. J Am Coll Cardiol 2013;61:1935-44. [Crossref] [PubMed]
- Johnson WD, Ganjoo AK, Stone CD, et al. The left atrial appendage: our most lethal human attachment! Surgical implications. Eur J Cardiothorac Surg 2000;17:718-22. [Crossref] [PubMed]
- Zheng Z, Jayaram R, Jiang L, et al. Perioperative Rosuvastatin in Cardiac Surgery. N Engl J Med 2016;374:1744-53. [Crossref] [PubMed]
- Wu JH, Marchioli R, Silletta MG, et al. Plasma phospholipid omega-3 fatty acids and incidence of postoperative atrial fibrillation in the OPERA trial. J Am Heart Assoc 2013;2:e000397. [Crossref] [PubMed]
- Costanzo S, di Niro V, Di Castelnuovo A, et al. Prevention of postoperative atrial fibrillation in open heart surgery patients by preoperative supplementation of n-3 polyunsaturated fatty acids: an updated meta-analysis. J Thorac Cardiovasc Surg 2013;146:906-11. [Crossref] [PubMed]
- Viviano A, Kanagasabay R, Zakkar M. Is perioperative corticosteroid administration associated with a reduced incidence of postoperative atrial fibrillation in adult cardiac surgery? Interact Cardiovasc Thorac Surg 2014;18:225-9. [Crossref] [PubMed]
- Fawzy H, Elatafy E, Elkassas M, et al. Can posterior pericardiotomy reduce the incidence of postoperative atrial fibrillation after coronary artery bypass grafting?dagger. Interact Cardiovasc Thorac Surg 2015;21:488-91. [Crossref] [PubMed]
- Gillinov AM, Bagiella E, Moskowitz AJ, et al. Rate Control versus Rhythm Control for Atrial Fibrillation after Cardiac Surgery. N Engl J Med 2016;374:1911-21. [Crossref] [PubMed]
- Zhao J, Feng Q, Wu P, et al. Learning from Longitudinal Data in Electronic Health Record and Genetic Data to Improve Cardiovascular Event Prediction. Sci Rep 2019;9:717. [Crossref] [PubMed]
- Thanassoulis G, Massaro JM, O'Donnell CJ, et al. Pericardial fat is associated with prevalent atrial fibrillation: the Framingham Heart Study. Circ Arrhythm Electrophysiol 2010;3:345-50. [Crossref] [PubMed]
- Cha MJ, Oh S. The Relationship Between Pericardial Fat and Atrial Fibrillation. J Atr Fibrillation 2013;5:676. [PubMed]
- Wang F, Zhu M, Wang X, et al. Predictive value of left atrial appendage lobes on left atrial thrombus or spontaneous echo contrast in patients with non-valvular atrial fibrillation. BMC Cardiovasc Disord 2018;18:153. [Crossref] [PubMed]
- NK C. inventor Nihon Kohden Afib Detection Program QP-039P 510(k) Summary 2016.
- Kohden N. EC1 Arrhythmia Monitoring Analysis Algorithm 2016.
- Healthcare G. The GE EK-Pro Arrhythmia Detection Algorithm for Patient Monitoring 2011.
- Philips. ST/AR ST segment and arrhythmia monitoring 2004.
- Wechselberger S, Kronborg M, Huo Y, et al. Continuous monitoring after atrial fibrillation ablation: the LINQ AF study. Europace 2018;20:f312-20. [Crossref] [PubMed]
- PocketECG. 2018. Available online: https://www.pocketecg.com/
- Rezaei Yousefi Z, Parak J, Tarniceriu A, et al., editors. Atrial Fibrillation Detection from Wrist Photoplethysmography Data Using Artificial Neural Networks. Singapore: Springer Singapore 2019.
- Krittanawong C, Zhang H, Wang Z, et al. Artificial Intelligence in Precision Cardiovascular Medicine. J Am Coll Cardiol 2017;69:2657-64. [Crossref] [PubMed]
- Turakhia MP, Desai M, Hedlin H, et al. Rationale and design of a large-scale, app-based study to identify cardiac arrhythmias using a smartwatch: The Apple Heart Study. Am Heart J 2019;207:66-75. [Crossref] [PubMed]
- Cardiology ACo. Apple Heart Study Identifies AFib in Small Group of Apple Watch Wearers. 2019.
- Bumgarner JM, Lambert CT, Hussein AA, et al. Smartwatch Algorithm for Automated Detection of Atrial Fibrillation. J Am Coll Cardiol 2018;71:2381-8. [Crossref] [PubMed]
- Ai-Cor. 2019. Available online: https://ai-cor.com/
- Parvaneh S, Rubin J, editors. Electrocardiogram Monitoring and Interpretation: From Traditional Machine Learning to Deep Learning, and Their Combination. 2018 Computing in Cardiology Conference (CinC); 2018 23-26 Sept. 2018.
- Xia Y, Wulan N, Wang K, et al. Detecting atrial fibrillation by deep convolutional neural networks. Comput Biol Med 2018;93:84-92. [Crossref] [PubMed]
- Teijeiro T, Félix P, Presedo J, et al. Heartbeat Classification Using Abstract Features From the Abductive Interpretation of the ECG. IEEE J Biomed Health Inform 2018;22:409-20. [Crossref] [PubMed]
- Zihlmann M, Perekrestenko D, Tschannen M, editors. Convolutional recurrent neural networks for electrocardiogram classification. 2017 Computing in Cardiology (CinC); 2017 24-27 Sept. 2017.
- Vollmer M, Sodmann P, Caanitz L, et al., editors. Can supervised learning be used to classify cardiac rhythms? 2017 Computing in Cardiology (CinC); 2017 24-27 Sept. 2017.
- Rubin J, Parvaneh S, Rahman A, et al., editors. Densely connected convolutional networks and signal quality analysis to detect atrial fibrillation using short single-lead ECG recordings. 2017 Computing in Cardiology (CinC); 2017 24-27 Sept. 2017.
- Stepien K, Grzegorczyk I, editors. Classification of ECG recordings with neural networks based on specific morphological features and regularity of the signal. 2017 Computing in Cardiology (CinC); 2017 24-27 Sept. 2017.
- Schwab P, Scebba GC, Zhang J, et al., editors. Beat by beat: Classifying cardiac arrhythmias with recurrent neural networks. 2017 Computing in Cardiology (CinC); 2017 24-27 Sept. 2017.
- Andreotti F, Carr O, Pimentel MAF, et al., editors. Comparing feature-based classifiers and convolutional neural networks to detect arrhythmia from short segments of ECG. 2017 Computing in Cardiology (CinC); 2017 24-27 Sept. 2017.
- Lehman LH, Silva I, Clifford GD, et al. AF Classification from a Short Single Lead ECG Recording: the PhysioNet/Computing in Cardiology Challenge 2017. Comput Cardiol 2010;2017:44.
- Parvaneh S, Rubin J, Rahman A, et al. Analyzing single-lead short ECG recordings using dense convolutional neural networks and feature-based post-processing to detect atrial fibrillation. Physiol Meas 2018;39:084003. [Crossref] [PubMed]
- Shashikumar SP, Shah AJ, Li Q, et al., editors. A deep learning approach to monitoring and detecting atrial fibrillation using wearable technology. 2017 IEEE EMBS International Conference on Biomedical & Health Informatics (BHI); 2017 16-19 Feb. 2017.
- Gotlibovych I, Crawford S, Goyal D, et al. End-to-end Deep Learning from Raw Sensor Data: Atrial Fibrillation Detection using Wearables. ArXiv 2018;abs/1807.10707.
- Poh MZ, Poh YC, Chan PH, et al. Diagnostic assessment of a deep learning system for detecting atrial fibrillation in pulse waveforms. Heart 2018;104:1921-8. [Crossref] [PubMed]
- Johnson LSB, Persson AP, Wollmer P, et al. Irregularity and lack of p waves in short tachycardia episodes predict atrial fibrillation and ischemic stroke. Heart Rhythm 2018;15:805-11. [Crossref] [PubMed]
- Boon KH, Khalil-Hani M, Malarvili MB. Paroxysmal atrial fibrillation prediction based on HRV analysis and non-dominated sorting genetic algorithm III. Comput Methods Programs Biomed 2018;153:171-84. [Crossref] [PubMed]
- López-López JA, Sterne JAC, Thom HHZ, et al. Oral anticoagulants for prevention of stroke in atrial fibrillation: systematic review, network meta-analysis, and cost effectiveness analysis. BMJ 2017;359:j5058. [Crossref] [PubMed]
- Atreya AR, Priya A, Pack QR, et al. Use and Outcomes Associated With Perioperative Amiodarone in Cardiac Surgery. J Am Heart Assoc 2019;8:e009892. [Crossref] [PubMed]
- D'Agostino RS, Jacobs JP, Badhwar V, et al. The Society of Thoracic Surgeons Adult Cardiac Surgery Database: 2017 Update on Outcomes and Quality. Ann Thorac Surg 2017;103:18-24. [Crossref] [PubMed]
- Giacomino BD, Cram P, Vaughan-Sarrazin M, et al. Association of Hospital Prices for Coronary Artery Bypass Grafting With Hospital Quality and Reimbursement. Am J Cardiol 2016;117:1101-6. [Crossref] [PubMed]