The value of preoperative spirometry testing for predicting postoperative risk in upper abdominal and thoracic surgery assessed using big-data analysis
Introduction
Over 230 million patients undergo surgery worldwide each year with reported hospital mortality rates of between 1% and 4% (1). Postoperative complications have an important clinical impact associated with increased rates of morbidity and mortality and longer hospital stay (1). Infectious postoperative complications are the main causes of postoperative morbidity in abdominal and thoracic surgery (2,3). The European Society of Anaesthesiology-European Society of Intensive Care Medicine guidelines of 2015 define infectious postoperative complications as surgical site infection, pneumonia, bloodstream infection, urinary tract infection and infection of unknown source (4). Both patient-related factors (age, pre-existing lung disease, obesity, smoking, malignancy) and procedure-related factors are known to predict postoperative complications (5,6).
Spirometry is used to assess lung function, and has been widely used as a preoperative test in thoracic surgery (7). Spirometry evaluates a patient’s forced expiratory volume in 1 sec (FEV1) and forced vital capacity (FVC), which are used in the diagnosis of obstructive or restrictive ventilatory defects (8). Spirometry is closely associated with chronic obstructive pulmonary disease (COPD), asthma and interstitial lung disease. Airflow limitation (FEV1/FVC <70% predicted) is an independent risk factor for postoperative complications in thoracic surgery (9). One systematic review identified a history of COPD as the major risk factor for postoperative pulmonary complications in non-cardiothoracic surgery (10). However, another reported that mild-to-moderate COPD, as defined by the Global Strategy for Obstructive Lung Disease (GOLD) guidelines, was not a significant risk factor for postoperative pulmonary complications in abdominal surgery (11). However, other studies showed that although FVC was an independent risk factor for postoperative pulmonary complications after abdominal surgery (12,13), FEV1 was not (12). There is no consensus about the value of spirometry for predicting postoperative pulmonary complications and infection.
The use of big data in healthcare may help to identify factors associated with clinical outcomes. The application of data-driven methods to healthcare data has improved our understanding of factors driving outcomes and their relationship with disease comorbidities. Big-data researchers should be able to easily acquire the necessary clinical data from a hospital’s information system to facilitate their research. In this study, we extracted the necessary clinical data from the Asan Biomedical Research Environment (ABLE) (14,15). Using these data, we evaluated spirometry results as independent risk factors for postoperative complications in thoracic and upper abdominal surgery.
Methods
Data source
We performed a retrospective analysis in the Asan Medical Center, a 2,704-bed, university-affiliated, tertiary referral hospital in Seoul. To protect patient privacy, comply with governmental regulations and facilitate research, Asan Medical Center has developed a biomedical research platform, ABLE. Patient medical record data have been stored since 2000, and the ABLE system extracts these data as categorical or continuous values. Comorbidity, smoking status and sex are extracted as categorical variables, and laboratory and spirometry data are extracted as continuous variables. Using this system, we could query patient data according to various conditions, such as date of surgery, surgical procedure or presence of spirometry data. Data cleansing was conducted using MySQL version 8.0.12 (Oracle, Cupertino, California, United States).
Clinical data extracted using ABLE are indexed by de-identified encrypted patient ID number (15,16) so that researchers cannot identify the patient.
Study design
We included patients who underwent general surgery between January 2000 and December 2014 at Asan Medical Center and who had pulmonary function testing within the 3 months prior to their surgery. The following surgical procedures were included, coded by the International Classification of Diseases, Ninth Revision, Clinical Modification (ICD-9-CM): lung lobectomy (32.0–32.4), gastrectomy (43.4–43.9), hepatectomy (50.0–50.4) and pancreaticoduodenectomy (51.5–51.7, 52.7). If a patient underwent more than one operation during the admission period, we included only their first operation. If patients underwent complex surgical procedures such as hepatectomy combined with cholecystectomy or with enterostomy or other operations that did not meet our inclusion criteria, those surgical procedures were defined as additional operations.
We extracted patient demographic data including age, sex, smoking history, underlying disease identified by ICD-9 code, spirometry data, laboratory data [haemoglobin, albumin, creatinine, blood urea nitrogen (BUN)], admission ward type (ICU, general ward), surgery date, and type and date of antibiotics administered.
Definitions
The GOLD guidelines define airflow limitation as a post-bronchodilator FEV1/FVC <0.70. However, spirometry using a bronchodilator is not usually performed for screening purposes. Therefore, we defined airflow limitation as FEV1/FVC <70% predicted combined with FEV1 <80% predicted without a bronchodilator (9).
Using the GOLD guidelines, we categorized the severity of patients’ lung function impairment based on the FEV1% predicted: FEV1 >80% (control), FEV1 50–80% (moderate impairment) and FEV1 <50% (severe impairment). There were so few patients with FEV1 <30% in our dataset (1.07%) that we included them in the severe group (FEV1 <50%) (17).
The severity of lung function impairment based on FVC % predicted was categorized as follows: FVC >80% (control), FVC 50–80% (moderate impairment) and FVC <50% (severe impairment).
Our dataset did not include information about whether the index surgery was emergency or elective. However, we could identify whether patients were admitted to the ICU or a general ward. Surgery in patients who had been admitted to the ICU was defined as an emergency operation, because ICU admission indicated that patients had unstable vital signs or other serious conditions.
Laboratory data were recorded as continuous variables; values for creatinine and BUN had skewed distributions. We grouped the laboratory data to indicate their clinical significance as described in previous studies (9,18,19).
Clinical outcomes
The primary outcome of interest was postoperative infection within 7 days. Secondary outcomes included prolonged ICU stay and in-hospital death. We presumed that patients with a postoperative infection would be treated with antibiotics. Thus, we defined a postoperative infectious complication as initiation of new antibiotics within 1–7 days post-surgery in patients who had been administered no other antibiotics except for prophylactic antibiotics (20,21). We defined prophylactic antibiotics according to guidelines (22) and as commonly used in our hospital. They included cefazolin, cefotetan, ceftezole, ceftizoxime, ceftriaxone, cefuroxime, flomoxef and isepamicin. New antibiotics commonly used for postoperative infection included antipseudomonal cephalosporins (cefepime, ceftazidime), antipseudomonal carbapenem (imipenem, meropenem), β-lactam/β-lactamase inhibitor (piperacillin–tazobactam), antipseudomonal fluoroquinolones (ciprofloxacin, levofloxacin), aminoglycosides (amikacin, gentamicin, tobramycin), linezolid or vancomycin.
Some patients underwent postoperative care in the ICU. Usually, patients without any complications or unstable vital signs were discharged from the ICU within 2 days after surgery. Hence, we defined a prolonged ICU stay as >2 days. Another primary outcome was in-hospital death.
Ethics approval
This study was approved by the ethics committee of the Asan Medical Center (approval number 2015-0656), and the need to obtain informed consent was waived because of the retrospective observational nature of the study. This study was conducted in accordance with the Declaration of Helsinki (as revised in 2013).
Statistical analysis
Continuous variables are presented as means and standard deviation (SD); categorical variables are presented as numbers and percentages. Univariate logistic regression was performed to identify associations between postoperative infection and other variables. Multivariable logistic regression was performed using variables with a P value <0.1 in the univariate analysis. Missing values were dropped in multivariable logistic analysis. In multivariable logistic regression, multicollinearity between the predictor variables was assessed by calculating the variance inflation factor (VIF) (23). In general, VIF >4 warrants further investigation, while VIF >10 is an indicator of serious multicollinearity that requires correction. Using a receiver operating characteristic (ROC) curve, we explored the predictive value of variables included in the multiple logistic regression for each outcome. The prediction model was evaluated by the area under the curve (AUC). All tests were two-sided, and a P value <0.05 was considered to indicate significance. Statistical analyses were performed using R version 3.5.1.
Results
Baseline characteristics
We included 31,827 patients who underwent thoracic or upper abdominal surgery between January 2000 and December 2014 and spirometry within the 3 months prior to their surgery. There were 514 patients with a history of physician-diagnosed COPD and 3,514 patients with airflow limitation.
The overall incidences of postoperative infection were 4.4% (n=1,414), of prolonged ICU care (>2 days) 5.4% (n=1,712) and of in-hospital death 0.8% (n=244). The mean age of patients was 59.3 years, and 28,037 (88.1%) had cancer: most of the operations were performed for cancer resection. The mean FEV1 predicted was 90.3% and 7,049 (22.1%) patients had a FEV1 predicted <80% (Table 1). Thoracic surgery accounted for approximately 20% of all operations, and abdominal surgery accounted for 80% (Table S1).
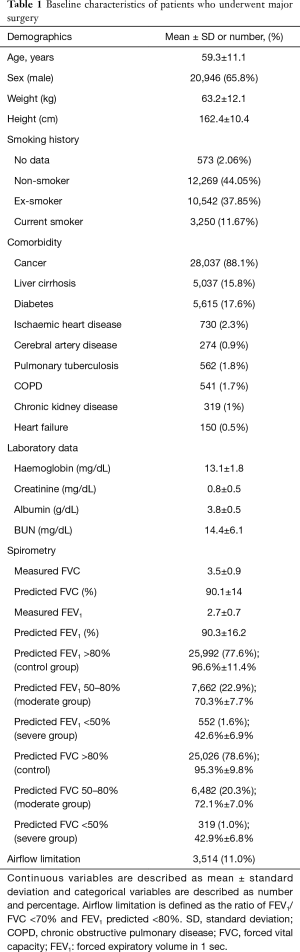
Full table
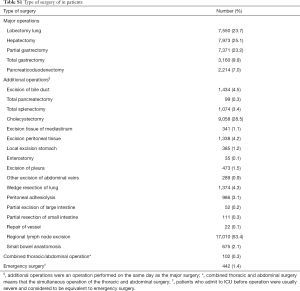
Full table
Spirometry and other risk factors for postoperative infection
The results of univariate logistic regression analysis are shown in Table 2. The overall postoperative infection rate was 4.4%. Risk factors identified in the univariate analysis included type of operation, sex, older age (>65 years), laboratory results and grades of FEV1% predicted and FVC % predicted. In multivariable logistic regression, the independent risk factors for postoperative infectious complications were sex, age, smoking, operation type, laboratory data (albumin, BUN) and spirometry data (Table 3). The number of missing values were 1,055 (3.3%). Lower FEV1 and FVC were both independent risk factors for postoperative infection [OR (95% CI): for FEV1 50–80% 1.86 (1.52–2.27); FEV1 <50% 3.99 (2.86–5.57); FVC 50–80% 1.49 (1.31–1.69); FEV <50% 2.93 (2.02–4.24)]. The VIF for FEV1 was 4.25 when all of the variables including FVC were included in the analysis. Therefore, separate multiple logistic regression analyses were performed including either FEV1 or FVC. The effect of other risk factors was robust but the risk of airflow limitation differed between FEV1 [OR (95% CI): 0.71 (0.57–0.88)] and FVC [OR (95% CI): 1.11 (0.96–1.28)]. Evaluation of the predictive power of these factors for postoperative infection showed that the 95% CI of the AUC was 0.643–0.673 (Table 2).
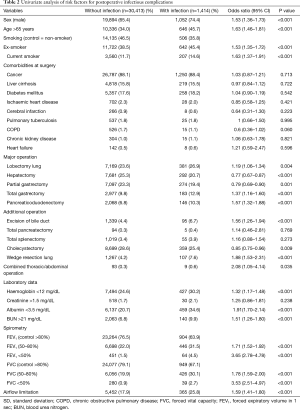
Full table
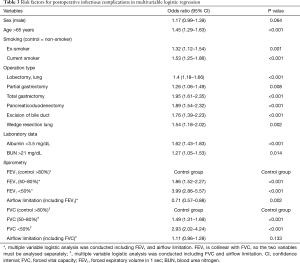
Full table
Spirometry and other risk factors for prolonged ICU stay and in-hospital death
The overall rate of prolonged ICU care was 5.3%. Univariate logistic analysis showed that the type of operation and patient demographic data were associated with a prolonged ICU stay (Table S2). In multivariable logistic analysis, laboratory data (haemoglobin, creatinine, albumin, BUN), type of surgery, emergency surgery and grades of FEV1% predicted and FVC % predicted were independent risk factors for a prolonged ICU stay (Table 4). The OR of airflow limitation for prolonged ICU care was significant for FEV1 [OR, 95% CI: 0.68 (0.56–0.81)] but not for FVC [OR, 95% CI: 0.98 (0.85–1.13)]. In this model, the 95% CI of the AUC was 0.839–0.862.
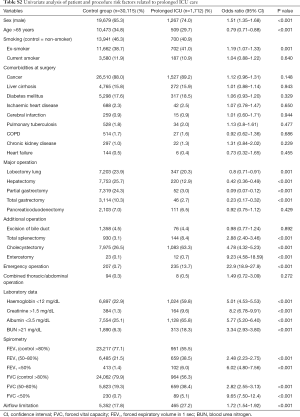
Full table
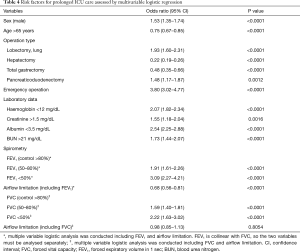
Full table
The overall rate of in-hospital death was 0.8% (Table S3). Multivariable logistic analysis showed that most of the variables produced results similar to those for ICU stay. However, enterostomy was an additional independent risk factor for in-hospital death (Table 5). Patients with lower grades of FEV1 or FVC had a higher risk of in-hospital death. However, airflow limitation was not a significant risk factor for in-hospital death in the multivariable model [OR, 95% CI: 0.72 (0.49–1.07) for FEV1; 1.24 (0.91–1.67) for FVC]. In this model, the 95% CI of the AUC was 0.803–0.852.
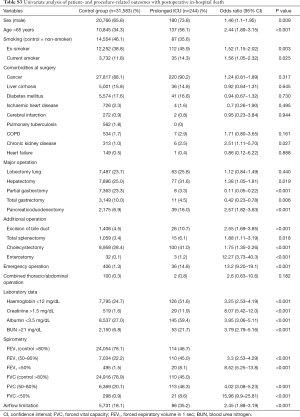
Full table
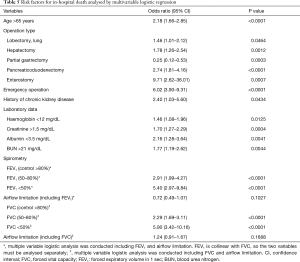
Full table
Discussion
Because the process of extracting big data has evolved, our hospital has developed an extraction system called ABLE (14). Using this system, we can easily search and extract data while protecting patient privacy. The purpose of this study was to use big-data analysis to investigate whether spirometry can be used as an independent predictor of morbidity and mortality. We found that FVC was an independent risk factor for postoperative infection and prolonged ICU care, regardless of airflow limitation. FEV1 also showed a significant relationship with postoperative infection and prolonged ICU care. However, the airway limitation of the lower FEV1 group had a protective effect against postoperative infection. Therefore, patients with low FEV1 but normal FVC have a lower risk of postoperative infection and prolonged ICU care than patients with low FEV1 and FVC. Thus, we suggest that low FVC is a more reliable indicator of the risk of postoperative infection and prolonged ICU care.
Spirometry is not recommended for patients undergoing nonthoracic surgery, except for those with preoperative asthma or COPD (24). Huh et al. support this recommendation because they found that preoperative spirometry results could not be used to stratify the risk of postoperative complications in older patients undergoing laparoscopic gastrectomy (25). Other studies have attempted to evaluate the predictive power of FVC or vital capacity for postoperative outcomes; however, FEV1 did not show a significant relationship with postoperative outcomes (12,13). In our study, the mean predicted FEV1 and FVC were 90.2 and 90.0, respectively, which were lower than those in previous studies. Over 20% of our patients were classified with moderate or severe airway limitation. Therefore, this study has greater strength than previous studies for evaluating the risk of lower FEV1 and FVC.
COPD (26) and airflow limitation (27,28) have been reported to be independent risk factors for postoperative pulmonary complications. Although airflow limitation correlates with lower FEV1, these studies did not consider the relationship between FEV1 or FVC and airflow limitation. In the present study, we found that although airflow limitation was positively correlated with postoperative infection in univariate analysis, in multivariable analysis it was negatively correlated with postoperative infection when FEV1 was included in the multivariable model.
Infectious complications are the main causes of postoperative morbidity in abdominal and thoracic surgery (3,29). Previous studies used varying definitions of postoperative infectious complications (29-31). Although it is important to classify postoperative infections, a diagnosis for postoperative complications was rarely coded in our electronic medical records. However, if an infection occurs after surgery, the antibiotics administered to the patient should be modified. Thus, we used this to define postoperative infections.
Shifting the definition of a variable between continuous and categorical may have clinical and statistical significance. In previous studies, patient age and laboratory data used to predict postoperative complications, such as creatinine, albumin and haemoglobin, were defined using varying cut-off values (10,18,19). The cut-off values chosen affect the descriptive power by changing the distribution of binary data. For outcome prediction, we used the most appropriate cut-off values based on the distribution of our data.
It was previously reported that emergency surgery was an independent risk factor for postoperative outcomes (2), with a more than fourfold higher risk of postoperative complications compared with elective surgery. Our electronic medical record system did not allow us to distinguish emergency from elective surgery. However, we assumed that admission to ICU before surgery had the same degree of risk as an emergency operation and found that presurgical ICU care was a risk factor for prolonged ICU stay and in-hospital death.
This study had some limitations. First, we defined postoperative infection based on antibiotic use. Identification of postoperative infections such as pneumonia or surgical site infection requires clinical judgement. However, patients infected after surgery should be treated with antibiotics, and the overall incidence of postoperative complications in our study was similar to those in previous studies (32-34). Thus, it is reasonable to assume that this factor has clinical significance. Second, there is some uncertainty regarding the clinical data because the patient’s history of comorbidity and the procedure code were often omitted or miscoded. In the future, natural language processing could be used to identify a history of comorbidity from the descriptive electronic medical records.
In conclusion, this study confirmed that FVC is a useful indicator of postoperative complications, regardless of airflow limitation.
Acknowledgments
The authors would like to thank the ABLE team for accessing the electronic medical records for this study.
Funding: None.
Footnote
Conflicts of Interest: All authors have completed the ICMJE uniform disclosure form (available at http://dx.doi.org/10.21037/jtd-19-2687). CMC serves as an unpaid editorial board member of Journal of Thoracic Disease from Oct 2019 to Sep 2021. The other authors have no conflicts of interest to declare.
Ethical Statement: The authors are accountable for all aspects of the work in ensuring that questions related to the accuracy or integrity of any part of the work are appropriately investigated and resolved. This study was approved by the ethics committee of the Asan Medical Center (approval number 2015-0656), and the need to obtain informed consent was waived because of the retrospective observational nature of the study. This study was conducted in accordance with the Declaration of Helsinki (as revised in 2013).
Open Access Statement: This is an Open Access article distributed in accordance with the Creative Commons Attribution-NonCommercial-NoDerivs 4.0 International License (CC BY-NC-ND 4.0), which permits the non-commercial replication and distribution of the article with the strict proviso that no changes or edits are made and the original work is properly cited (including links to both the formal publication through the relevant DOI and the license). See: https://creativecommons.org/licenses/by-nc-nd/4.0/.
References
- Weiser TG, Regenbogen SE, Thompson KD, et al. An estimation of the global volume of surgery: a modelling strategy based on available data. Lancet 2008;372:139-44. [Crossref] [PubMed]
- Fernandez-Bustamante A, Frendl G, Sprung J, et al. Postoperative Pulmonary Complications, Early Mortality, and Hospital Stay Following Noncardiothoracic Surgery: A Multicenter Study by the Perioperative Research Network Investigators. JAMA Surg 2017;152:157-66. [Crossref] [PubMed]
- Jang HJ, Song JW, Cho S, et al. Prognostic Implications of Postoperative Infectious Complications in Non-Small Cell Lung Cancer. Korean J Thorac Cardiovasc Surg 2018;51:41-52. [Crossref] [PubMed]
- Jammer I, Wickboldt N, Sander M, et al. Standards for definitions and use of outcome measures for clinical effectiveness research in perioperative medicine: European Perioperative Clinical Outcome (EPCO) definitions: a statement from the ESA-ESICM joint taskforce on perioperative outcome measures. Eur J Anaesthesiol 2015;32:88-105. [Crossref] [PubMed]
- Kim HJ, Lee J, Park YS, et al. Impact of GOLD groups of chronic pulmonary obstructive disease on surgical complications. Int J Chron Obstruct Pulmon Dis 2016;11:281-7. [PubMed]
- Jeong BH, Shin B, Eom JS, et al. Development of a prediction rule for estimating postoperative pulmonary complications. PLoS One 2014;9:e113656. [Crossref] [PubMed]
- Brunelli A, Kim AW, Berger KI, et al. Physiologic evaluation of the patient with lung cancer being considered for resectional surgery: Diagnosis and management of lung cancer, 3rd ed: American College of Chest Physicians evidence-based clinical practice guidelines. Chest 2013;143:e166S-90S.
- Ranu H, Wilde M, Madden B. Pulmonary function tests. Ulster Med J 2011;80:84-90. [PubMed]
- Yoshimi K, Oh S, Suzuki K, et al. Impact of Airflow Limitation on Comorbidities and Postoperative Complications in Patients Undergoing Thoracic Surgery: A Retrospective Observational Study. Ann Thorac Cardiovasc Surg 2016;22:146-52. [Crossref] [PubMed]
- Smetana GW, Lawrence VA, Cornell JE. Preoperative pulmonary risk stratification for noncardiothoracic surgery: systematic review for the American College of Physicians. Ann Intern Med 2006;144:581-95. [Crossref] [PubMed]
- Kim TH, Lee JS, Lee SW, et al. Pulmonary complications after abdominal surgery in patients with mild-to-moderate chronic obstructive pulmonary disease. Int J Chron Obstruct Pulmon Dis 2016;11:2785-96. [Crossref] [PubMed]
- Oh TK, Park IS, Ji E, et al. Value of preoperative spirometry test in predicting postoperative pulmonary complications in high-risk patients after laparoscopic abdominal surgery. PLoS One 2018;13:e0209347. [Crossref] [PubMed]
- Tajima Y, Tsuruta M, Yahagi M, et al. Is preoperative spirometry a predictive marker for postoperative complications after colorectal cancer surgery? Jpn J Clin Oncol 2017;47:815-9. [Crossref] [PubMed]
- Shin Y, Choi C, Lee J, et al. First Step to Big Data Research in Hospital. Stud Health Technol Inform 2015;216:924. [PubMed]
- Shin SY, Lyu Y, Shin Y, et al. Lessons Learned from Development of De-identification System for Biomedical Research in a Korean Tertiary Hospital. Healthc Inform Res 2013;19:102-9. [Crossref] [PubMed]
- Shin SY, Park YR, Shin Y, et al. A De-identification method for bilingual clinical texts of various note types. J Korean Med Sci 2015;30:7-15. [Crossref] [PubMed]
- Pellegrino R, Viegi G, Brusasco V, et al. Interpretative strategies for lung function tests. Eur Respir J 2005;26:948-68. [Crossref] [PubMed]
- Simões CM, Carmona MJC, Hajjar LA, et al. Predictors of major complications after elective abdominal surgery in cancer patients. BMC Anesthesiol 2018;18:49. [Crossref] [PubMed]
- Schumacher C, Eismann H, Sieg L, et al. Preoperative Recipient Parameters Allow Early Estimation of Postoperative Outcome and Intraoperative Transfusion Requirements in Liver Transplantation. Prog Transplant 2018;28:116-23. [Crossref] [PubMed]
- Platt R, Kleinman K, Thompson K, et al. Using automated health plan data to assess infection risk from coronary artery bypass surgery. Emerg Infect Dis 2002;8:1433-41. [Crossref] [PubMed]
- Miner AL, Sands KE, Yokoe DS, et al. Enhanced identification of postoperative infections among outpatients. Emerg Infect Dis 2004;10:1931-7. [Crossref] [PubMed]
- Bratzler DW, Dellinger EP, Olsen KM, et al. Clinical practice guidelines for antimicrobial prophylaxis in surgery. Surg Infect (Larchmt) 2013;14:73-156. [Crossref] [PubMed]
- Vatcheva KP, Lee M, McCormick JB, et al. Multicollinearity in Regression Analyses Conducted in Epidemiologic Studies. Epidemiology (Sunnyvale) 2016;6:227. [Crossref] [PubMed]
- Qaseem A, Snow V, Fitterman N, et al. Risk assessment for and strategies to reduce perioperative pulmonary complications for patients undergoing noncardiothoracic surgery: a guideline from the American College of Physicians. Ann Intern Med 2006;144:575-80. [Crossref] [PubMed]
- Huh J, Sohn TS, Kim JK, et al. Is routine preoperative spirometry necessary in elderly patients undergoing laparoscopy-assisted gastrectomy? J Int Med Res 2013;41:1301-9. [Crossref] [PubMed]
- Bapoje SR, Whitaker JF, Schulz T, et al. Preoperative evaluation of the patient with pulmonary disease. Chest 2007;132:1637-45. [Crossref] [PubMed]
- Mikami Y, Matsuzaki H, Ishimori T, et al. Evaluation of postoperative pulmonary complications in FEV1/FVC. European Respiratory Journal 2017;50:PA645.
- Jeong O, Ryu SY, Park YK. The value of preoperative lung spirometry test for predicting the operative risk in patients undergoing gastric cancer surgery. J Korean Surg Soc 2013;84:18-26. [Crossref] [PubMed]
- Pessaux P, Msika S, Atalla D, et al. Risk factors for postoperative infectious complications in noncolorectal abdominal surgery: a multivariate analysis based on a prospective multicenter study of 4718 patients. Arch Surg 2003;138:314-24. [Crossref] [PubMed]
- Herbert G, Perry R, Andersen HK, et al. Early enteral nutrition within 24 hours of lower gastrointestinal surgery versus later commencement for length of hospital stay and postoperative complications. Cochrane Database Syst Rev 2018;10:CD004080. [PubMed]
- Ferrante M, de Buck van Overstraeten A, Schils N, et al. Perioperative Use of Vedolizumab is not Associated with Postoperative Infectious Complications in Patients with Ulcerative Colitis Undergoing Colectomy. J Crohns Colitis 2017;11:1353-61. [Crossref] [PubMed]
- Carvalho RLR, Campos CC, Franco LMC, et al. Incidence and risk factors for surgical site infection in general surgeries. Rev Lat Am Enfermagem 2017;25:e2848. [Crossref] [PubMed]
- Chughtai M, Gwam CU, Khlopas A, et al. The Incidence of Postoperative Pneumonia in Various Surgical Subspecialties: A Dual Database Analysis. Surg Technol Int 2017;30:45-51. [PubMed]
- Stéphan F, Sax H, Wachsmuth M, et al. Reduction of Urinary Tract Infection and Antibiotic Use after Surgery: A Controlled, Prospective, Before-After Intervention Study. Clin Infect Dis 2006;42:1544-51. [Crossref] [PubMed]