Tumor density is associated with response to endobronchial ultrasound-guided transbronchial needle injection of cisplatin
Introduction
Over half of all advanced lung cancers will progress or recur despite appropriate therapy, a problem associated with a median overall survival of approximately 5 months (1). The advent of immunotherapy has improved this prognosis for the 20–50% of patients who respond, the majority of patients remain without effective therapies (2-4). Due to the lack of options for these patients, there has been increasing interest in direct delivery of agents into tumors for adjuvant or palliative indications (5-10). The rationale for this approach lies in the opportunity to achieve therapeutic concentrations of the agent within the tumor while minimizing exposure to the systemic circulation and off-target organs.
The most commonly utilized direct delivery approach is endobronchial ultrasound-guided transbronchial needle injection of cisplatin (EBUS-TBNI cisplatin) (11-14). In contrast to intravenous administration, different regional concentrations of cisplatin may be achieved depending on where within the tumor the agent is delivered. Based on initial descriptions (15), the two US institutions that have reported using EBUS-TBNI cisplatin independently adopted a practice of delivering up to 40 mg of cisplatin into the tumor at each treatment (11,13). This dose, however, has been selected on entirely empirical grounds. Furthermore, there are no guidelines as to where within the tumor the cisplatin should be injected to maximize the effective distribution. The administration of cisplatin by EBUS-TBNI could potentially be very heterogeneous depending on the spatial injection pattern that is employed. In contrast to the heterogeneous distribution achieved following IV administration (that in part results from varying blood vessel density) appropriately selecting the dose and delivery sites within the tumor for EBUS-TBNI will allow us to optimize the distribution and efficacy of the agent. However, we must first understand the tumor characteristics that influence the final distribution of the agent.
It is apparent that tumors with large volumes or greater maximum diameters may be more challenging to treat due to the longer diffusion distances and lower effective concentration of a single EBUS-TBNI dose. It also seems reasonable to suppose that regional variations in cisplatin diffusivity could play an important role in the therapeutic effectiveness of EBUS-TBNI by influencing how well cisplatin is able to be distribute throughout the tumor before being cleared into the vasculature by the tumor’s blood supply. Testing this latter idea directly is challenging because cisplatin diffusivity is not easily measured in situ. On the other hand, diffusivity is likely to be reflected by the distribution of mass density of the tumor tissue, which itself can be determined from a 3-dimensional (3D) CT scan. Accordingly, the goal of the present study was to determine how a variety of pre-defined, quantitative, tumor image characteristics, termed “radiomics”, influence the response of lung tumors to EBUS-TBNI cisplatin therapy (16,17). Radiomics features capture both morphologic features, such as maximum diameter, as well as intensity based features, which are markers of tissue density (18). Leveraging data from two centers, we sought to understand how features such as geometric structure and density influence response to EBUS-TBNI cisplatin therapy.
Methods
Study oversight
The study was conducted in accordance with the Declaration of Helsinki (as revised in 2013). This study was approved by the University of Vermont (UVM) Committee on Human Research in the Medical Sciences (CHRMS 17-075) and by The University of Florida (UFL) Institutional Review Board (201700864). Informed consent was not required by these oversight committees due to the retrospective nature of the study.
Study populations
All cases where EBUS-TBNI cisplatin performed between September 2010 and December 2017 were reviewed by the two institutions. All patients included in this series had recurrent lung cancer following radiation therapy. Recurrence was pathologically confirmed. Each case was presented at the local multidisciplinary tumor board and EBUS-TBNI cisplatin recommended by the respective group. The goal of therapy in all cases was local control, either due to a local recurrence or with palliative intent for treatment of a non-stentable airway obstruction. Cases without CT scans (e.g., only PET images) or with CT scans performed more than 5 weeks prior to onset of EBUS-TBNI therapy were excluded. Descriptive data and outcomes from portions of the two cohorts have been published previously (11,13).
CT scans for all included cases were assessed in a blinded fashion. Response to therapy was defined as either complete response (CR) or partial response (PR) based on the Response Evaluation Criteria in Solid Tumors (RECIST) 1.1 criteria (19). An example is show in Figure 1. All other imaging data were acquired via automated quantitative analysis.
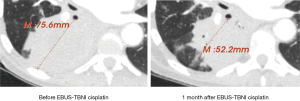
Quantitative CT analysis
All CT scans were evaluated while blinded to the outcome variable of response. Semi-automated segmentation of the treated volume was performed as previously described using the freely available Chest Imaging Platform (https://chestimagingplatform.org) (20-22). A single point was also placed on the right deltoid. Variance of the density of this point was measured to allow for normalization of CT scan reconstruction in the statistical models. Twenty-four radiomics features were then extracted from the segmented lesion based on previously published algorithms for assessment of intensity and morphology (https://pyradiomics.readthedocs.io/en/latest/features.html, accessed 1/20/2019).
Statistical analysis
All statistical analyses were performed using STATA (Version 13, College Station, TX, USA). P values less than or equal to 0.05 were considered significant and all statistical tests were two-sided. In order to limit the degrees of freedom, demographic and clinical features were selected via backwards stepwise selection with an inclusion criteria of 0.10.
The higher dimensionality of radiomics features necessitated using the commonly utilized approach of logistic regression with least absolute shrinkage and selection operator (LASSO) regularization (23,24). This approach allows for selection of features in high dimensionality data while simultaneously penalizing correlated predictors. Two separate LASSO operations were performed, one within each radiomics category (Figure 2). The top three features from each category were selected for evaluation in logistic regression models.
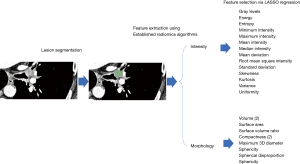
Logistic regression models were constructed with the outcome of response to EBUS-TBNI cisplatin. Models were compared using the likelihood ratio test and Akaike’s information criterion (AIC).
Results
Thirty-eight cases met criteria for inclusion in the analysis (28 UFL, 10 UVM). Characteristics of the cohort by response, are shown in Table 1. There were no significant differences in any of the variables in Table 1 by response. There were also no significant differences in the distribution of any of those variables by institution. There was a significant difference in the range of slice thickness of obtained CT scans between the two institutions (UVM 0.9 mm, UFL 1–3.75 mm). There was no difference in the overall response rate (70% vs. 71%, P=0.62) between the two institutions.
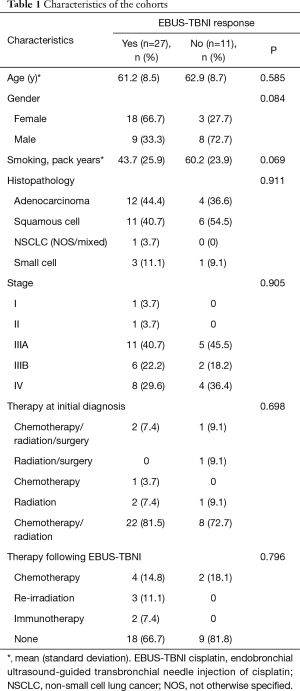
Full table
For construction of the models, we first evaluated clinical variables as predictors of response to EBUS-TBNI cisplatin. Although our primary goal was to evaluate tumor features as predictors of response, clinicoepidemiologic variables were included to account for other important predictors. Backwards stepwise selection resulted in only gender and smoking remaining in the model (P=0.018). Test of interaction for gender and smoking was not significant (P=0.627).
LASSO regression of all intensity features resulted in selection of variance, energy, and kurtosis. A separate LASSO regression of morphology features resulted in selection of maximum 3D diameter, surface to volume ratio, and spherical disproportion as the top three features. No feature alone was significantly associated with response (Table 2). For comparison we also included the selected variables from both the Intensity and Morphology categories in a single LASSO. The top 3 selected features were all related to Intensity (variance, energy, and kurtosis, respectively) followed by surface-to-volume ratio as the highest ranked Morphology feature.
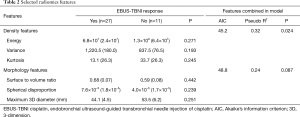
Full table
Each of these sets of features were then included with gender and smoking and the two models compared. Models were also evaluated with inclusion of the deltoid variance measurement.
Evaluation of LASSO selected features demonstrated that inclusion of Morphology features with gender and smoking did not result in a significant chi-square test for the overall model (P=0.087), whereas inclusion of Intensity features did result in a significant model (P=0.024, Table 2). Moreover, inclusion of Intensity vs. Morphology features resulted in a superior AIC (45 vs. 49), and pseudo R square (0.32 vs. 0.24), respectively.
There was no significant change in the overall performance of the models when the deltoid variance measurement was omitted from the Intensity and Morphology models (P=0.0125 vs. P=0.0743, respectively). Because there was a significant difference in the CT slice thickness obtained at the two different centers we also performed a stratified analysis. Stratifying the models to only UFL, did not result in a significant change in overall model performance for either the Intensity or Morphology models (P=0.014 vs. P=0.123).
Discussion
Over the last several years EBUS-TBNI cisplatin has evolved as a potential therapeutic option for patients with recurrent lung cancer, who previously received radiation to that region and are not receiving systemic cytotoxic therapy (8,11-15,25). Despite a robust response rate, the ideal dosing and administration strategy for this therapy remains unknown. Although it is intuitive that the relationship of tumor size (a proxy for the total number of tumor cells) to cisplatin dose may be a driver of overall tumor response there is accumulating evidence that tumor density may also be important. For instance, our group recently completed a computational modeling analysis that demonstrated the critical importance of the diffusion rate in determining the efficacy of the therapy (26).
Multiple factors are known to affect the diffusion of an agent through a tumor. Several of the most prominent include density of the tissue, rate of uptake of the agent to the intracellular compartment, and the distribution and flow of blood through the local vascular bed that serves as a sink for the agent. Tissue density in particular may vary widely among tumors and includes regions of both low-density necrotic tissue and high-density collagen produced by the tumor. These considerations motivated the current investigation.
This is the first multicenter analysis of this therapy, and the largest to date. We leveraged quantitative imaging techniques to perform multiparametric measurements from tumors treated with EBUS-TBNI cisplatin. Surprisingly, measures of tumor volume were not as highly associated with response to EBUS-TBNI cisplatin as measures of density. It is important to note that several of the tumor features found to be important in this analysis are metrics of variation in tumor density, specifically variance and kurtosis. Energy is defined as the square of the mean intensity and is related more directly to the actual density of the lesion. Although not statistically significant by itself, energy was lower in the responders vs. the non-responders. One potential explanation for this finding is that high density regions may decrease diffusion of the agent.
Despite inclusion of two centers, the overall sample size is small due to the focused indication for which the procedure is performed. Thus, it is important to note that models constructed here have not been validated for prediction of response to therapy and are not sufficiently powered to do so. The goal of the models (constructed from both clinicoepidemiologic and radiomics features) was to gain insight into the underlying biology that may partially determine response while accounting for important confounders. Regardless of the performance of the models, LASSO regression of the radiomics features alone selected measures of density as more highly associated with response to EBUS-TBNI cisplatin. Similarly, radiomics features from only two categories, Intensity and Morphology, were evaluated to limit the degrees of freedom. These two were chosen due to their straightforward biological interpretation and preliminary data indicating their potential importance. We cannot comment on the association of other radiomics features. The CT scans evaluated in this investigation were obtained as part of clinical care. Thus, there were differences in CT parameters such as slice thickness between the two centers. However, stratifying the models based on center level variables (e.g., CT slice thickness) did not result in a significant difference in the results. Similarly, adjusting for reconstruction algorithms by including the density variance of the same anatomic regions across the CT scans did not change model performance.
In summary, our analysis of clinical data and radiomics features from a multicenter cohort of patients undergoing EBUS-TBNI cisplatin indicates that tumor density may be an important determinant of response to direct intratumoral therapy. These results have implications for further device development and drug delivery approaches.
Acknowledgments
CMK presented this work in abstract form at the Annual Meeting of the American Thoracic Society 2019.
Funding: This study was supported by NIH HLK23133476 (CMK), UVM Department of Medicine Grant (CMK).
Footnote
Data Sharing Statement: Available at http://dx.doi.org/10.21037/jtd-20-674
Conflicts of Interest: All authors have completed the ICMJE uniform disclosure form (available at http://dx.doi.org/10.21037/jtd-20-674). CMK reports grants from NIH, during the conduct of the study; personal fees from Olympus America, grants and personal fees from Johnson and Johnson, personal fees from Boston Scientific, personal fees from Gala Therapeutics, outside the submitted work. In addition, CMK has a patent Methods for Computational Modeling to Guide Intratumoral Therapy pending and Equity holder of company, Quantitative Imaging Solutions, the performs image analysis work in lung cancer. RSJE reports grants from NHLBI, personal fees from Chiesi, personal fees from LeukoLabs, grants and personal fees from Boehringer Ingelheim, personal fees from Eolo Medical, outside the submitted work; and he is also a founder and co-owner of Quantitative Imaging Solutions which is a company that provides image based consulting and develops software to enable data sharing. Dr. JHTB reports personal fees from Johnson and Johnson, outside the submitted work. In addition, Dr. JHTB has a patent Methods for computational modeling to guide intratumoral therapy (U.S. Patent Application No. 62/542,623. Filed: August 8, 2017 pending). GW reports grants from NIH, grants and other from Boehringer Ingelheim, other from Quantitative Imaging Solutions, other from PulmonX, grants from BTG Interventional Medicine, grants and other from Janssen Pharmaceuticals, other from GlaxoSmithKline, other from Novartis, other from Vertex, outside the submitted work; and Dr. GW’s spouse works for Biogen. The other authors have no conflicts of interest to declare.
Ethical Statement: The authors are accountable for all aspects of the work in ensuring that questions related to the accuracy or integrity of any part of the work are appropriately investigated and resolved. The study was conducted in accordance with the Declaration of Helsinki (as revised in 2013). This study was approved by the University of Vermont (UVM) Committee on Human Research in the Medical Sciences (CHRMS 17-075) and by The University of Florida (UFL) Institutional Review Board (201700864). Informed consent was not required by these oversight committees due to the retrospective nature of the study.
Open Access Statement: This is an Open Access article distributed in accordance with the Creative Commons Attribution-NonCommercial-NoDerivs 4.0 International License (CC BY-NC-ND 4.0), which permits the non-commercial replication and distribution of the article with the strict proviso that no changes or edits are made and the original work is properly cited (including links to both the formal publication through the relevant DOI and the license). See: https://creativecommons.org/licenses/by-nc-nd/4.0/.
References
- Fossella FV, DeVore R, Kerr RN, et al. Randomized phase III trial of docetaxel versus vinorelbine or ifosfamide in patients with advanced non-small-cell lung cancer previously treated with platinum-containing chemotherapy regimens. J Clin Oncol 2000;18:2354-62. [Crossref] [PubMed]
- Borghaei H, Paz-Ares L, Horn L, et al. Nivolumab versus docetaxel in advanced nonsquamous non-small-cell lung cancer. N Engl J Med 2015;373:1627-39. [Crossref] [PubMed]
- Brahmer J, Reckamp KL, Baas P, et al. Nivolumab versus docetaxel in advanced squamous-cell non-small-cell lung cancer. N Engl J Med 2015;373:123-35. [Crossref] [PubMed]
- Reck M, Rodríguez-Abreu D, Robinson AG, et al. Pembrolizumab versus chemotherapy for PD-L1-positive non-small-cell lung cancer. N Engl J Med 2016;375:1823-33. [Crossref] [PubMed]
- Lee JM, Lee MH, Garon E, et al. Phase I trial of intratumoral injection of CCL21 gene-modified dendritic cells in lung cancer elicits tumor-specific immune responses and CD8+ T-cell infiltration. Clin Cancer Res 2017;23:4556-68. [Crossref] [PubMed]
- Harris K, Puchalski J, Sterman D. Recent advances in bronchoscopic treatment of peripheral lung cancers. Chest 2017;151:674-85. [Crossref] [PubMed]
- Ost DE, Sterman DH. Interventional bronchoscopy in 2015. Removing endoluminal and methodological obstructions. Ann Am Thorac Soc 2015;12:1265-6. [Crossref] [PubMed]
- Li X, Liu X, Rao X, et al. A case report of local treatment of inoperable squamous cell lung carcinoma with convex-probe endobronchial ultrasound-guided intratumoral injection of cisplatin in a patient with severe COPD. Medicine 2017;96:e7070. [Crossref] [PubMed]
- Marabelle A, Andtbacka R, Harrington K, et al. Starting the fight in the tumor: expert recommendations for the development of human intratumoral immunotherapy (HIT-IT). Ann Oncol 2018;29:2163-74. [Crossref] [PubMed]
- Melero I, Gato M, Shekarian T, et al. Repurposing infectious disease vaccines for intratumoral immunotherapy. J Immunother Cancer 2020;8:e000443. [Crossref] [PubMed]
- Khan F, Anker CJ, Garrison G, et al. Endobronchial ultrasound-guided transbronchial needle injection for local control of recurrent non-small cell lung cancer. Ann Am Thorac Soc 2015;12:101-4. [Crossref] [PubMed]
- Kinsey CM, Garrison G. Endobronchial ultrasound transbronchial needle injection: ex vivo measurement of dead space volume of the needle assembly. Ann Am Thorac Soc 2015;12:1253-4. [PubMed]
- Mehta HJ, Begnaud A, Penley AM, et al. Treatment of isolated mediastinal and hilar recurrence of lung cancer with bronchoscopic endobronchial ultrasound guided intratumoral injection of chemotherapy with cisplatin. Lung Cancer 2015;90:542-7. [Crossref] [PubMed]
- Kinsey CM. Endobronchial ultrasound-guided-transbronchial needle injection for direct therapy of lung cancer. Ame Medical J 2018;3:74. [Crossref]
- Hohenforst-Schmidt W, Zarogoulidis P, Darwiche K, et al. Intratumoral chemotherapy for lung cancer: re-challenge current targeted therapies. Drug Des Devel Ther 2013;7:571-83. [PubMed]
- Aerts HJ, Velazquez ER, Leijenaar RT, et al. Decoding tumour phenotype by noninvasive imaging using a quantitative radiomics approach. Nat Commun 2014;5:4006. [Crossref] [PubMed]
- Lambin P, Velazquez ER, Leijenaar R, et al. Radiomics: extracting more information from medical images using advanced feature analysis. Eur J Cancer 2012;48:441-6. [Crossref] [PubMed]
- van Griethuysen JJM, Fedorov A, Parmar C, et al. Computational radiomics system to decode the radiographic phenotype. Cancer Res 2017;77:e104-7. [Crossref] [PubMed]
- Eisenhauer EA, Therasse P, Bogaerts J, et al. New response evaluation criteria in solid tumours: Revised RECIST guideline (version 1.1). Eur J Cancer 2009;45:228-47. [Crossref] [PubMed]
- Kinsey CM, Estépar RSJ, Wei Y, et al. Regional emphysema of a non-small cell tumor is associated with larger tumors and decreased survival rates. Ann Am Thorac Soc 2015;12:1197-205. [PubMed]
- Washko GR, Hunninghake GM, Fernandez IE, et al. Lung volumes and emphysema in smokers with interstitial lung abnormalities. N Engl J Med 2011;364:897-906. [Crossref] [PubMed]
- Castaldi PJ, San José Estépar R, Mendoza CS, et al. Distinct quantitative computed tomography emphysema patterns are associated with physiology and function in smokers. Am J Respir Crit Care Med 2013;188:1083-90. [Crossref] [PubMed]
- Huang Y, Liu Z, He L, et al. Radiomics Signature: a potential biomarker for the prediction of disease-free survival in early-stage (I or II) non-small cell lung cancer. Radiology 2016;281:947-57. [Crossref] [PubMed]
- Peikert T, Duan F, Rajagopalan S, et al. Novel high-resolution computed tomography-based radiomic classifier for screen-identified pulmonary nodules in the National Lung Screening Trial. PLoS One 2018;13:e0196910. [Crossref] [PubMed]
- Celikoglu F, Celikoglu SI, York AM, et al. Intratumoral administration of cisplatin through a bronchoscope followed by irradiation for treatment of inoperable non-small cell obstructive lung cancer. Lung Cancer 2006;51:225-36. [Crossref] [PubMed]
- Mori V, Roy GS, Bates JHT, et al. Cisplatin pharmacodynamics following endobronchial ultrasound-guided transbronchial needle injection into lung tumors. Sci Rep 2019;9:6819. [Crossref] [PubMed]