Bioinformatics and integrated analyses of prognosis-associated key genes in lung adenocarcinoma
Introduction
Lung cancer (LC) is the most frequently diagnosed cancer worldwide and has the highest death rate (1,2). Of the different types of LC, approximately 80–85% are non-small cell LC (NSCLC) with adenocarcinoma accounting for very considerable proportion (2-4). Previous studies have discussed the factors affecting the development and tumorigenesis of lung adenocarcinoma (LUAD), including genomic mutation of EGFR and KRAS, and the gene fusion detection of ALK, which have been widely used in clinical practice (5-7). Although activating EGFR mutations for NSCLCs are considered predictive biomarkers for tyrosine kinase inhibitor (TKI) treatment (8), the total EGFR mutation frequency is only around 30% (9). Despite initially positive responses to EGFR TKI therapy, which is the standard main treatment for LUAD, most patients develop progression and TKI resistance during 1–2 years after TKI treatment commencement (10). Positive ALK rearrangement occurs in 3–5% of NSCLCs. Although ALK-positive NSCLC patients benefit from crizotinib, the development of acquired resistance still causes treatment failure and poor prognosis (11). There are also some problems, such as difficulties in early diagnosis and chemoradiotherapy resistance, that remain to be addressed (12-14). The 5-year survival rate of patients with advanced LUAD is still low at 3–10% following diagnosis (15). Current prognostic and predictive biomarkers are not adequate, and the pathogenesis of LUAD is still far from clear. Therefore, further investigations on sensitive biomarkers that are related to overall survival (OS) are urgent.
Genome expression profiles have been widely used to identify prognostic signatures in patients with cancer. Deferentially expressed genes (DEGs) affecting prognosis have been analyzed and are widely used in clinical practice. Weighted gene co-expression network analysis (WGCNA), a method to elucidate the interactions of pathogenic genes in cellular processes, is a bioinformation tool used to determine the correlation between hub genes and modules with clinical traits (16,17). We applied WGGNA to the Gene Expression Omnibus (GEO) dataset to identify key genes that are related to pathway modules and to provide LUAD prognostic markers. This systematical biologic method of co-expression network for models has been used to study many cancers, such as pancreatic cancer (18), gastric adenocarcinoma (19), and renal clear cell carcinoma (20). However, relative research in LUAD is still insufficient.
In the present study, we set up a gene co-expression network of DEGs from the GSE32863 dataset using the WGCNA package (version 1.69) and identified specific modules correlated with the clinical and pathophysiological characteristics of LUAD. Furthermore, the data were analyzed by Gene Ontology (GO), Kyoto Encyclopedia of Genes and Genomes (KEGG), and Kaplan-Meier Plotter analysis for predictive key genes of LUAD patients (21). The function of key genes [dynein light chain roadblock-type 2 (DYNLRB2) and mouse homolog of ß1 spectrin (SPTBN1)] were confirmed by the gene set enrichment analysis (GSEA) database (22). Our article was the first report to suggest a link between the DYNLRB2 and SPTBN1 expression and LUAD prognosis. We also verified the mRNA expression of DYNLRB2 by quantitative reverse transcription polymerase chain reaction (qRT-PCR), and examined the protein expression by Western blotting. Functional experiments were performed in vitro. Our aim was to identify specific biomarkers closely correlated with the prognosis of LUAD, and provide insight into the mechanism of LUAD.
We present the following article in accordance with the MDAR reporting checklist (available at http://dx.doi.org/10.21037/jtd-21-49).
Methods
Data collection
The GSE32863 dataset contained gene expression profiling of 58 LUAD samples, and normal adjacent lung tissues were downloaded from the GEO repository (http://www.ncbi.nlm.nih.gov). Datasets were downloaded in CEL format and analyzed on R (version 3.1.2). After setting the threshold as adj. P<0.05 and |logFC|>1, DEGs from candidate genes were screened using the limma R package. The study was conducted in accordance with the Declaration of Helsinki (as revised in 2013).
Pathway enrichment analysis
To explore the functional pathways of DEGs, GO enrichment and KEGG pathway analysis of both upregulated and downregulated DEGs using the cluster profiler package, the threshold value was set as P<0.05.
WGCNA for modules
We constructed a weight co-expression network of DEGs using WGCNA package version 1.69. We filtered the genes with top 25% variance using an algorithm for further analysis. We excluded outlier samples before incorporating DEGs into the co-expression network. Network modules were divided by a dynamic tree-cutting algorithm. To assess the stability of modules identified in the microarray dataset, we used a module preservation analysis (nPermutations =200) to detect the stability of the modules. A soft threshold (β=7) was used to transform the correlation matrix into a signed weighted adjacency matrix. The preservation statistics, Zsummary and median rank, were used to quantify the preservation of gene modules. Modules with Zsummary >10 indicated strong preservation, and a lower preservation statistics median rank indicated reliable preservation; these were selected as the key modules. The different module eigengenes (MEs) and clinical traits, including age, sex, ethnicity, smoking history and the number of cigarettes smoked each year, and stage of disease development were evaluated by Pearson’s correlation test. The module with module significance <0.05 and correlation >0.25 was selectedfor further analysis. Correlations of modules were shown using a heat map.
Screening for hub genes
In our study, hub genes were screened as the overlapping genes between DEGs and significant modules using Cytoscape 3.7.0 (https://cytoscape.org) for in-depth analysis and validation. The key genes in in the intersection region between DEGs and modules are showed in the Venn diagram.
Hub gene analysis
The Kaplan-Meier Plotter database (http://kmplot.com) is an online survival analysis website (23). The database contains research on 54,675 genes and 18,674 cancer samples in cancers, such as breast cancer and LC. Based on the results of the survival analysis from Kaplan-Meier Plotter website, we verified the influence of different key genes expressions on the prognostic effect of LUAD patients.
Validation of the mRNA expression by The Cancer Genome Atlas Program (TCGA)
We used Gene Expression Profiling Interactive Analysis (GEPIA; http://gepia.cancer-pku.cn/), a website for RNA expression analysis of various tumors based on the TCGA database (24), to verify whether the expression of the selected key gene was significantly associated with LUAD by boxplots.
GSEA
We used GSEA version 2.21 software for the pathway of key genes. We divided LUAD samples into 2 groups according to their expression levels. We used the C2 cp.kegg.v5.1. symbols.gmt dataset from the Molecular Signature Database version 6.2 as the reference gene set. The method of default weighted enrichment statistics was used for the enrichment analysis. We set the random combination times to 1,000.
Cell culture and transfection
A549, a lung cancer cell line was purchased from the Shanghai Institute of Cell Biology (Shanghai, China). Cells were cultured in Dulbecco’s modified Eagle’s medium buffer which was purchase from Invitrogen (Carlsbad, CA, USA) with 10% fetal bovine serum (Thermo Fisher Scientific, Waltham, MA, USA) and 1% penicillin-streptomycin solution (Invitrogen, Carlsbad, CA, USA). The cells were cultured in an incubator with 5% CO2 at 37 °C. When cell density reached 80%, cells were generated. We prepared transfected A549 cells with DYNLRB2 overexpression, according to the manufacturer’s protocols (GenSript, Piscataway, New Jersey, USA). A549 cells at 90–95% confluence were transfected using Lipofectamine 2000 (Invitrogen, Carlsbad, CA, USA) with the plasmids pIRES2-ZsGreen1-homo-DYNLRB2 and plasmids pIRES2-ZsGreen1. All plasmid DNA was extracted using an Invitrogen Purelink HiPure Plasmid Kit (Invitrogen, Carlsbad, CA, USA). A549 cells were transfected with the plasmid using X-tremeGENE HP (Roche Diagnostics, Shanghai, China) and continuously cultured for 48 h.
Cell counting kit-8 (CCK-8) assays and apoptosis analysis
After transfection for 48 h, we tested cell viability using CCK-8 assay (n=3/group). CCK-8 buffer (MCE, Shanghai, China) (10 µL) was added into the wells and further cultured in an incubator at 37 °C for 4 h. The microplate reader was used to measure the absorbance value at 450 nm.
The apoptosis analyze was implemented after 48 h transfection (n=3/group). After digestion with 0.25% ethylenediaminetetraacetic acid-free trypsin (Invitrogen, Carlsbad, CA, USA) and washing, the cells were centrifuged at 1,500 rpm to remove the supernatant. Apoptosis was evaluated using an Annexin V-APC/7-AAD apoptosis detection kit (BioLegend, San Diego, CA, USA).
Western blot analysis
Protein expression levels of DYNLRB2 in transfected cells were confirmed by Western blot analysis. A549 transfected cells were harvested 48 h after transfection and extracted by lysis buffer with 0.01% protease inhibitor and phenylmethylsulphonyl fluoride. The cells were centrifuged at 12,000 rpm at 4 °C for 5 min to remove the supernatant. The protein concentration was measured using the BCA Protein Quantitation Kit (Thermo Fisher, MA, USA). Each 20 μL sample was added to 10% sodium dodecylsulfate-polyacrylamide gel electrophoresis. The polyvinylidene difluoride membranes were blocked for 2 h with 5% non-fat milk in Tris-buffered saline containing 0.05% Tween-20 (TBST) at room temperature. The membranes were further incubated with primary antibodies targeting rabbit anti-human DYNLRB2 (Sigma-Aldrich, St. Louis, MO, USA) (1:1,000) and rabbit anti-human GAPDH (Sigma-Aldrich, St. Louis, MO, USA) (1:1,000) at 4 °C overnight, then washed 5 times with TBST. The membranes were further incubated with horseradish peroxidase-conjugated secondary antibody (Bio-Rad, Hercules, CA, USA) (1:50,000) diluted in TBST. After washing, the membranes were reacted via enhanced chemiluminescence (ECL) solution (Bio-Rad, Hercules, CA, USA). Finally, the gel band intensity was analyzed by BandScan 5.0 software for protein concentration.
qRT-PCR
RNA was extracted from A549 transfected cells and A549 cells using 1 mL TRIzol reagent (Invitrogen, Carlsbad, CA, USA). PCR was performed using the 7500 Fast Real-Time PCR System (Applied Biosystems, Foster City, CA, USA) with the following primers: Homo DYNLRB2 sense 5'-ATGGCAGAGGTGGAGGAAAC-3' and reverse 5'-TGGCTTTCATTGTCAGGTGA-3'. Homo GAPDH 5'-TCAAGAAGGTGGTGAAGCAGG-3' and reverse 5'-TCAAAGGTGGAGGAGTGGGT-3'. Homo DYNLRB2 was normalized by GAPDH using 2-ΔΔCq method.
Statistical analysis
Quantitative data which were generated from three independent experiments and presented as means ± standard deviation (SD). The data were compared using independent sample t-test. SPSS 24.0 (IBM, Armonk, NY, USA) was used for statistical analysis. P values <0.05 were considered statistically significant.
Results
DEG identification and enrichment analysis
GSE32863 containing 23,762 RNAs was analyzed using the limma package following preprocessing. In total, 1,587 DEGs were identified, including 649 upregulated genes and 938 downregulated genes in the LUAD samples, as shown in a volcano map (Figure 1A). The top 100 DEGs, according to |logFC| value, are shown in a heatmap (Figure 1B).
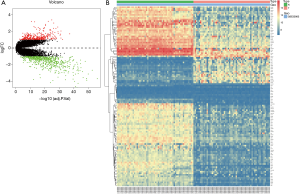
DEGs were analyzed using the clusterProfiler package for enrichment analysis. P<0.05 was defined as a meaningful cut-off value. In the GO analysis, upregulated genes that were significantly enriched included cell adhesion molecule binding, extracellular matrix structural constituent conferring tensile strength and so on (Figure 2A). A total of 71 biologic processes were obtained by downregulating genes. We selected the top 12 biologic processes, as shown in Figure 2B. Downregulated genes were enriched in glycosaminoglycan binding, cytokine binding, growth factor binding and so on. In the KEGG analysis, upregulated genes were mainly enriched in biosynthesis of amino acids, carbon metabolism and so on (Figure 2C). Downregulated genes were mainly enriched in complement and coagulation cascades, Staphylococcus aureus infection, osteoclast differentiation, pertussis and so on (Figure 2D). Significantly enriched pathways results demonstrated that DEGs play important roles in LUAD development.
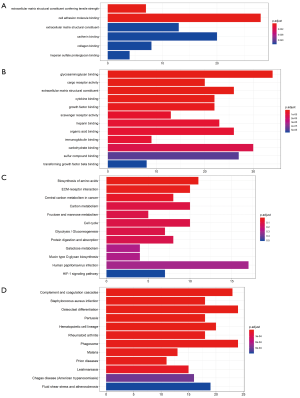
Construction of co-expression modules of LUAD by WGCNA
The top 25% variance DEGs were filtered by an algorithm for further analysis to set up the weighted co-expression network. We excluded 2 cluster height surpass samples before incorporating DEGs into the co-expression network (Figure 3A). The other 114 samples were retained for further analysis to determine the modules of highly correlated genes by WGCNA. A power value of β=7 was selected as the soft threshold parameter (Figure 3B), and 13 modules were generated (Figure 3C). Module stability was tested by the module preservation function in the WGCNA package (nPermutations =200). Zsummary statistics and median rank statistics for each module were then tested (Figure 3D). The interaction relationship of the modules was analyzed by plotting a network heatmap (Figure 3E). Based on the clinical traits in GSE32863, the interaction analysis of co-expression modules was performed. Among the 13 modules, those with module significance <0.05 and correlation >0.25 were identified to be associated modules. We found that module green and module pink correlated with smoking status, module yellow correlated with age and recurrence were considered as the key modules of interest. Smoking status, age, and recurrence were closely related to the treatment of cancer. In total, we chose genes from these modules that were most relevant to LUAD for further analysis.
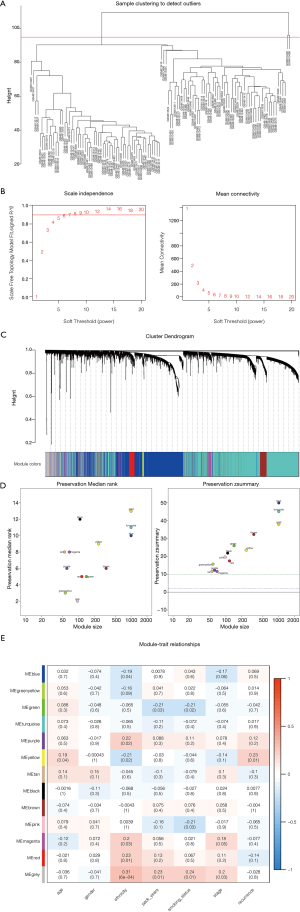
Screening for hub genes and survival analysis
To better extract key genes for prognosis from these data, a total of 15 key genes were found to overlap between WGCNA modules and the DEGs in GSE32863. Of these, 8 key downregulation genes were identified from module green (Figure 4A), 5 key upregulation genes were identified from module pink (Figure 4B), and 1 upregulation gene and 1 downregulation gene were identified from module yellow (Figure 4C). Survival analysis for key genes further evaluated their effects on the prognosis of LUAD. The Kaplan-Meier Plotter database search revealed that the expressions of DYNLRB and SPRBN1 were closely associated with LUAD patient survival. Patients with lower DYNLRB2 and SPRBN1 expressions had significantly shorter OS time than those with higher expressions (P≤0.05) (Figure 5).
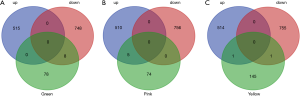
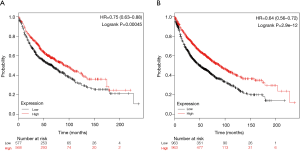
Validation of expressions of DYNLRB2 and SPTBN1 in LUAD by TCGA database
We then validated whether the screened hub genes were significantly associated with LUAD based on other data extracted from the TCGA database. We observed a decrease in DYNLRB2 and SPRBN1 expressions (Wilcoxon rank sum, P<0.05) in tumor tissue than normal tissue (Figure 6).
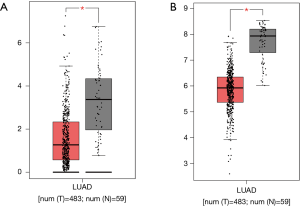
Enrichment analysis for key gene expression in LUAD
We investigated the biologic characteristics of different key genes in LUAD by GSEA assay. The GSEA results showed that upregulated DYNLRB2 and SPTBN1 were both enriched in drug metabolism cytochrome P450, cardiac muscle contraction, and retinol metabolism. Downregulated DYNLRB2 and SPTBN1 were associated with homologous recombination, base excision repair, and progesterone-mediated oocyte maturation (Figure 7).
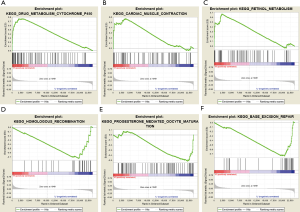
In vitro DYNLRB2 experiments
We first found that the expression of DYNLRB2 was downregulated in LUAD tissues by bioinformation analysis. The findings of our study indicated that DYNLRB2 was dysregulated and might be a suppressor gene for LUAD. We further verified this conclusion in vitro. We then upregulated DYNLRB2 expression using a plasmid vector (pIRES2-ZsGreen1-homo-DYNLRB2) in A549 cells and compared this with a negative control (DYNLRB2-NC). The mRNA level and protein level of DYNLRB2 were tested. The PCR analysis results showed that DYNLRB2 in the A549 was upregulated 17.6 times versus Normal Control (NC) (Figure 8). The protein level of DYNLRB2 increased after transfection with the pIRES2-ZsGreen1-homo-DYNLRB2 plasmid vector (P<0.01) (Figure 9). CCK-8 assays were then used to identify the influence of DYNLRB2 on A549 cell proliferation. Compared with the NC group, DYNLRB2 overexpressed cells were significantly inhibited (P<0.01) (Figure 10). Apoptosis analyses were performed to determine if DYNLRB2 changed the apoptotic rate in transfected cells. There was an increasing apoptotic rate in A549 cells transfected with pIRES2-ZsGreen1-homo-DYNLRB2 compared with A549 cells transfected with the NC vector (P<0.001). No significant difference was observed in A549 cells transfected with the NC vector and in normal A549 cells (Figure 11).
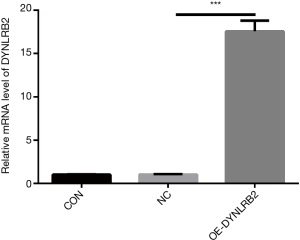
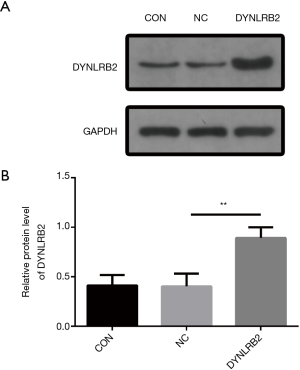
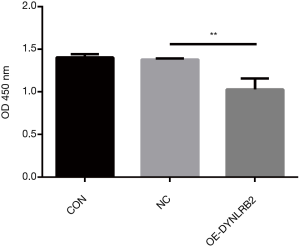
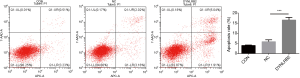
Discussion
Efficient diagnostic markers and accurate therapeutic targets are lacking for LUAD patients. Therefore, the aims of the present study were to identify potential biomarkers closely correlated with LUAD prognosis and to provide insight into the mechanism of LUAD. We collected GSE32863 from GEO datasets and constructed a gene co-expression network with WGCNA to study the data. GO and KEGG enrichment analyses showed that DEGs in GSE32863 might play a role in LUAD. Significantly changed modules that correlated with LUAD were identified. The results showed that 3 modules screened out by WGCNA were closely related to clinical traits of LUAD; these were age, recurrence of LUAD, and smoking status. Those genes were verified using TCGA and survival analysis. The results showed DYNLRB2 and SPRBN1 in modules could serve as biomarkers for prognosis.
DYNLRB2 has been considered a suppressor gene for many cancers. DYNLRB2 was found to be dysregulated in lung squamous cell carcinoma and nasopharyngeal carcinoma (25,26). DYNLRB2 was also found to be related to mixed primary ductal carcinoma in situ of the breast (27). Previously published studies have suggested that DYNLRB2 downregulation could be involved in tumor progression and pathogenesis mechanisms. It also could serve as a molecular switch that determines invasive progress and could be involved in cellular migration (27,28). Our study demonstrated that DYNLRB2 was dysregulated in LUAD and might be a suppressor gene for LUAD. Our data also confirmed that the overexpression of DYNLRB2 significantly suppressed A549 cell growth and caused apoptosis in vitro. Further analysis of the functional consequences of reduced DYNLRB2 expression should be carried out in LUAD.
SPTBN1 is an adapter protein for transforming growth factor-ß signaling through the Smad3/Smad4 complex formation. SPTBN1 is related to tumor cell motility, adhesion, migration, and invasion (29,30). SPTBN1 is recently considered a negative regulator of tumorigenesis. It was reported that the downregulation of SPTBN1 is found in human hepatocellular carcinoma (HCC), ovarian cancer and colon cancer (29-31). In pancreatic cancer, decreased SPTBN1 expression suggested worsening prognosis (32). SPTBN1-ALK gene fusion in LUAD has been found to cause resistance to multiple therapies (33). SPTBN1 could work as a tumor suppressor in tumorigenesis; however, further studies are required to determine its effect on LUAD development.
Downregulated DYNLRB2 and SPTBN1 affect the homologous recombination, progesterone-mediated oocyte maturation, and base excision repair pathways, which are closely associated with LUAD development. Dysfunction of homologous recombination is associated with many cancers and considered as the most important one (34,35). Homologous recombination is a major repair pathway for double-strand break repair (36). The overexpression of the homologous recombination is associated with metastasis development and a high Ki67 score (37). It also regulates sensitivity to chemotherapeutic drugs. Disorder of the homologous recombination increases sensitivity to anticancer drug treatment (38,39). The overexpression of homologous recombination in lung tumor tissues leads to low chemosensitivity of patients with NSCLC (37). It also influences radiosensitivity in NSCLC (40). Therefore, DYNLRB2 and SPTBN1 via the homologous recombination regulate the development of LUAD.
Although a comprehensive bioinformatics analysis using GEO datasets and in vitro experiments were performed, the present study had some limitations, we did not deeply prove potential mechanism how they effect LUAD prognosis. Further in vivo experiments are required to verify our results. Nevertheless, the present study provides novel insight into LUAD pathogenesis.
Conclusions
In the present study, bioinformatics analysis tools were used for determining biomarkers for LUAD prognosis. Our results indicated that DYNLRB2 and SPRBN1 were key genes in LUAD. In addition, the expressions of DYNLRB2 and SPRBN1 were found to be good prognostic makers. Further studies should focus on exploring the biologic functions and molecular mechanism of DYNLRB2 and SPRBN1 for LUAD.
Acknowledgments
Funding: This study was partially supported by Guangxi key research and development program No. (GK) AB18221080.
Footnote
Reporting Checklist: The authors have completed the MDAR reporting checklist. Available at http://dx.doi.org/10.21037/jtd-21-49
Conflicts of Interest: All authors have completed the ICMJE uniform disclosure form (available at http://dx.doi.org/10.21037/jtd-21-49). The authors have no conflicts of interest to declare.
Ethical Statement: The authors are accountable for all aspects of the work in ensuring that questions related to the accuracy or integrity of any part of the work are appropriately investigated and resolved. The study was conducted in accordance with the Declaration of Helsinki (as revised in 2013).
Open Access Statement: This is an Open Access article distributed in accordance with the Creative Commons Attribution-NonCommercial-NoDerivs 4.0 International License (CC BY-NC-ND 4.0), which permits the non-commercial replication and distribution of the article with the strict proviso that no changes or edits are made and the original work is properly cited (including links to both the formal publication through the relevant DOI and the license). See: https://creativecommons.org/licenses/by-nc-nd/4.0/.
References
- Chen W, Zheng R, Baade PD, et al. Cancer statistics in China, 2015. CA Cancer J Clin 2016;66:115-32. [Crossref] [PubMed]
- Besse B, Adjei A, Baas P, et al. 2nd ESMO Consensus Conference on Lung Cancer:non-small-cell lung cancer first-line/second and further lines of treatment in advanced disease. Ann Oncol 2014;25:1475-84. [Crossref] [PubMed]
- Dempke WC, Suto T, Reck M. Targeted therapies for non-small cell lung cancer. Lung cancer 2010;67:257-74. [Crossref] [PubMed]
- Ferlay J, Shin HR, Bray F, et al. Estimates of worldwide burden of cancer in 2008:GLOBOCAN 2008. Int J Cancer 2010;127:2893-917. [Crossref] [PubMed]
- Maemondo M, Inoue A, Kobayashi K, et al. Gefitinib or chemotherapy for non–small-cell lung cancer with mutated EGFR. N Engl J Med 2010;362:2380-8. [Crossref] [PubMed]
- Riely GJ, Marks J, Pao W. KRAS mutations in non–small cell lung cancer. Proc Am Thorac Soc 2009;6:201-5. [Crossref] [PubMed]
- Camidge DR, Bang YJ, Kwak EL, et al. Activity and safety of crizotinib in patients with ALK-positive non-small-cell lung cancer:updated results from a phase 1 study. Lancet Oncol 2012;13:1011-9. [Crossref] [PubMed]
- Tracy S, Mukohara T, Hansen M, et al. Gefitinib induces apoptosis in the EGFRL858R non–small-cell lung cancer cell line H3255. Cancer Res 2004;64:7241-4. [Crossref] [PubMed]
- Tanaka T, Matsuoka M, Sutani A, et al. Frequency of and variables associated with the EGFR mutation and its subtypes. Int J Cancer 2010;126:651-5. [Crossref] [PubMed]
- Jänne PA, Yang JC, Kim DW, et al. AZD9291 in EGFR inhibitor–resistant non–small-cell lung cancer. N Engl J Med 2015;372:1689-99. [Crossref] [PubMed]
- Katayama R, Shaw AT, Khan TM, et al. Mechanisms of acquired crizotinib resistance in ALK-rearranged lung cancers. Sci Transl Med 2012;4:120ra17. [Crossref] [PubMed]
- Minna JD, Roth JA, Gazdar AF. Focus on lung cancer. Cancer Cell 2002;1:49-52. [Crossref] [PubMed]
- Koning CC, Wouterse SJ, Daams JG, et al. Toxicity of concurrent radiochemotherapy for locally advanced non–small-cell lung cancer: a systematic review of the literature. Clin Lung Cancer 2013;14:481-7. [Crossref] [PubMed]
- Willers H, Azzoli CG, Santivasi WL, et al. Basic mechanisms of therapeutic resistance to radiation and chemotherapy in lung cancer. Cancer J 2013;19:200-7. [Crossref]
- Zhou X, Kemp BL, Khuri FR, et al. Prognostic implication of microsatellite alteration profiles in early-stage non-small cell lung cancer. Clin Cancer Res 2000;6:559-65. [PubMed]
- Langfelder P, Horvath S. WGCNA: an R package for weighted correlation network analysis. BMC Bioinformatics 2008;9:559. [Crossref] [PubMed]
- Saris CG, Horvath S, van Vught PW, et al. Weighted gene co-expression network analysis of the peripheral blood from Amyotrophic Lateral Sclerosis patients. BMC Genomics 2009;10:405. [Crossref] [PubMed]
- Giulietti M, Righetti A, Principato G, et al. LncRNA co-expression network analysis reveals novel biomarkers for pancreatic cancer. Carcinogenesis 2018;39:1016-25. [Crossref] [PubMed]
- Yepes S, López R, Andrade RE, et al. Co-expressed miRNAs in gastric adenocarcinoma. Genomics 2016;108:93-101. [Crossref] [PubMed]
- Wu P, Liu JL, Pei SM, et al. Integrated genomic analysis identifies clinically relevant subtypes of renal clear cell carcinoma. BMC Cancer 2018;18:287. [Crossref] [PubMed]
- Györffy B, Lanczky A, Eklund AC, et al. An online survival analysis tool to rapidly assess the effect of 22,277 genes on breast cancer prognosis using microarray data of 1,809 patients. Breast Cancer Res Treat 2010;123:725-31. [Crossref] [PubMed]
- Cavalieri D, Castagnini C, Toti S, et al. Eu. Gene Analyzer a tool for integrating gene expression data with pathway databases. Bioinformatics 2007;23:2631-2. [Crossref] [PubMed]
- Hou GX, Liu P, Yang J, et al. Mining expression and prognosis of topoisomerase isoforms in non-small-cell lung cancer by using Oncomine and Kaplan–Meier plotter. PLoS One 2017;12:e0174515. [Crossref] [PubMed]
- Tang Z, Li C, Kang B, et al. GEPIA: a web server for cancer and normal gene expression profiling and interactive analyses. Nucleic Acids Res 2017;45:W98-102. [Crossref] [PubMed]
- Tian F, Zhao J, Fan X, et al. Weighted gene co-expression network analysis in identification of metastasis-related genes of lung squamous cell carcinoma based on the Cancer Genome Atlas database. J Thorac Dis 2017;9:42-53. [Crossref] [PubMed]
- Ye Z, Wang F, Yan F, et al. Bioinformatic identification of candidate biomarkers and related transcription factors in nasopharyngeal carcinoma. World J Surg Oncol 2019;17:60. [Crossref] [PubMed]
- Liao S, Desouki MM, Gaile DP, et al. Differential copy number aberrations in novel candidate genes associated with progression from in situ to invasive ductal carcinoma of the breast. Genes Chromosomes Cancer 2012;51:1067-78. [Crossref] [PubMed]
- Jiang J, Yu L, Huang X, et al. Identification of two novel human dynein light chain genes, DNLC2A and DNLC2B, and their expression changes in hepatocellular carcinoma tissues from 68 Chinese patients. Gene 2001;281:103-13. [Crossref] [PubMed]
- Zhi X, Lin L, Yang S, Bhuvaneshwar K, et al. βII‐Spectrin (SPTBN1) suppresses progression of hepatocellular carcinoma and Wnt signaling by regulation of Wnt inhibitor kallistatin. Hepatology 2015;61:598-612. [Crossref] [PubMed]
- Chen M, Zeng J, Chen S, et al. SPTBN1 suppresses the progression of epithelial ovarian cancer via SOCS3-mediated blockade of the JAK/STAT3 signaling pathway. Aging 2020;12:10896-911. [Crossref] [PubMed]
- Tang Y, Katuri V, Srinivasan R, et al. Transforming growth factor-beta suppresses nonmetastatic colon cancer through Smad4 and adaptor protein ELF at an early stage of tumorigenesis. Cancer Res 2005;65:4228-37. [Crossref] [PubMed]
- Jiang X, Gillen S, Esposito I, et al. Reduced expression of the membrane skeleton protein beta1-spectrin (SPTBN1) is associated with worsened prognosis in pancreatic cancer. Histol Histopathol 2010;25:1497-506. [PubMed]
- Gu FF, Zhang Y, Liu YY, et al. Lung adenocarcinoma harboring concomitant SPTBN1-ALK fusion, c-Met overexpression, and HER-2 amplification with inherent resistance to crizotinib, chemotherapy, and radiotherapy. J Hematol Oncol 2016;9:66. [Crossref] [PubMed]
- Li D, Liu H, Jiao L, et al. Significant effect of homologous recombination DNA repair gene polymorphisms on pancreatic cancer survival. Cancer Res 2006;66:3323-30. [Crossref] [PubMed]
- Lips EH, Mulder L, Hannemann J, et al. Indicators of homologous recombination deficiency in breast cancer and association with response to neoadjuvant chemotherapy. Ann Oncol 2011;22:870-6. [Crossref] [PubMed]
- Helleday T. Pathways for mitotic homologous recombination in mammalian cells. Mutat Res 2003;532:103-15. [Crossref] [PubMed]
- Saviozzi S, Ceppi P, Novello S, et al. Non–small cell lung cancer exhibits transcript overexpression of genes associated with homologous recombination and DNA replication pathways. Cancer Res 2009;69:3390-6. [Crossref] [PubMed]
- Helleday T. Homologous recombination in cancer development, treatment and development of drug resistance. Carcinogenesis 2010;31:955-60. [Crossref] [PubMed]
- Helleday T, Petermann E, Lundin C, et al. DNA repair pathways as targets for cancer therapy. Nat Rev Cancer 2008;8:193-204. [Crossref] [PubMed]
- Yin M, Liao Z, Huang YJ, et al. Polymorphisms of homologous recombination genes and clinical outcomes of non-small cell lung cancer patients treated with definitive radiotherapy. PLoS One 2011;6:e20055. [Crossref] [PubMed]
(English Language Editor: R. Scott)