Rationale and design of the Early Chronic Obstructive Pulmonary Disease (ECOPD) study in Guangdong, China: a prospective observational cohort study
Introduction
Chronic obstructive pulmonary disease (COPD) is characterized by persistent respiratory symptoms and airflow obstruction, usually caused by airway and/or alveolar abnormalities as a result of exposure to toxic particles or gases (1). According to the latest Chinese epidemiological survey results, the prevalence of COPD among people over 40 years old has increased from 8.2% in 2007 to 13.7% (2,3). It is estimated that COPD will rise to become the third leading cause of death worldwide by 2030 (4). The prevalence of COPD is high and the burden of disease on society is heavy. Moreover, COPD is a heterogeneous disease and its clinically relevant subtypes are not well understood, especially the clinically relevant subtypes of early COPD (5,6).
Identifying individuals who will eventually develop airflow obstruction consistent with a diagnosis of COPD may facilitate therapeutic interventions with the potential to modify the course of disease (7). In the 2001 Global Initiative for Chronic Obstructive Lung Disease (GOLD) report, it was proposed that GOLD 0 represents a population with normal lung function but with chronic respiratory symptoms (cough and sputum production), that is, a high-risk population who could develop COPD in the future (8). However, recent studies have found that it remains uncertain whether individuals with normal lung function and chronic symptoms of cough and sputum production are more likely to develop COPD than those without these symptoms, although the latter population has a higher risk of respiratory disease-related mortality and all-cause mortality (9-12). Recently, a systematic review summarized that individuals who are at risk of subsequently developing COPD with a preserved forced expiratory volume in 1 second (FEV1)/forced vital capacity (FVC) in terms of the three aspects of symptoms, structure, and function defines them as having pre-COPD (7). However, the current understanding of which individuals are more likely to progress to airflow obstruction is still incomplete in many respects.
At present, the cohorts for studying high-risk groups for COPD and early COPD mainly include the COPDGene study (13), SPIROMICS study (14), CanCOLD study (15), SOON study (16), FOOTPRINTS study (17), and MARKO cohort (18). However, these cohort studies have only included current smokers and have not included non-smokers. Thus, the study findings from the above cohort studies have limited generalizability because 30–40% of patients with COPD do not smoke, especially in low- and middle-income countries (19-21).
In view of this evidence gap, we designed a prospective observational cohort study in Guangdong, China entitled the Early Chronic Obstructive Pulmonary Disease (ECOPD) study. The ECOPD study has four specific aims: (I) identification of characteristics, parameters, and biomarkers that may predict development of airflow obstruction and annual decline in lung function with normal spirometry; (II) identification of clinically relevant early COPD subtypes; (III) identification of characteristics, parameters, and biomarkers that may predict disease progression in these early COPD subtypes; and (IV) development and validation of machine learning models to predict development of airflow obstruction and disease progression.
We present the following article in accordance with the SPIRIT reporting checklist (available at https://dx.doi.org/10.21037/jtd-21-1379).
Methods
Study design and population
The diagnostic criteria for early COPD are still inconclusive, so this protocol does not provide a clear definition of early COPD. We will adopt whether FEV1/FVC is greater than or less than 0.7 for enrollment in the study design, and we will further analyze the definitions of early COPD one by one in the future analysis of cohort data. This is a prospective observational cohort study among individuals aged 40–80 years in Guangdong, China. We will recruit approximately 2,000 participants (1,000 without COPD, 800 with GOLD stage 1–2, and 200 with GOLD stage 3–4) from the community using a population-based survey for COPD. Participants will complete standard respiratory epidemiological questionnaires and pre-bronchodilator and post-bronchodilator spirometry. We will also collect information regarding health outcomes [modified British Medical Research Council (mMRC) dyspnea scale, COPD assessment test (CAT) and COPD clinical questionnaire (CCQ)], We will also obtain satellite remote sensing data to assess pollutant exposure. All Participants with post-bronchodilator FEV1/FVC <0.70 will be invited to participate in the ECOPD study. According to the chronological order of spirometry completion, we will randomly invite a quarter of participants who have post-bronchodilator FEV1/FVC ≥0.70 to participate in the ECOPD study. Participants who are invited and willing to participate in the study will further complete a chest computed tomography (CT) scan, impulse oscillometry (IOS), and biomarker measurement (blood and urine). Subgroup will additionally complete induced sputum, exercise capacity tests [6-minute walk test (6MWT) and cardiopulmonary exercise testing (CPET)] and home monitoring/personal sampling as pollutant exposure measures (Figure 1).
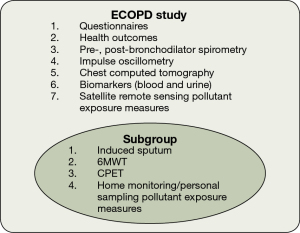
The inclusion criteria are as follows: (I) aged 40–80 years; (II) willing to participate in this study and providing a signed informed consent form; (III) completing the standard respiratory epidemiological questionnaire in this study; and (IV) completing pre-bronchodilator and post-bronchodilator spirometry. The exclusion criteria are as follows: (I) aged <40 or >80 years; (II) respiratory infection or exacerbation in the 4 weeks prior to screening; (III) heart attack (myocardial infarction, malignant arrhythmia) in the past 3 months; (IV) hospitalized for heart disease within the past 1 month; (V) chest, abdomen, or eye surgery in the past 3 months; (VI) previous lobectomy; (VII) malignant tumors newly discovered and being treated; (VIII) receiving anti-tuberculosis drug treatment or active pulmonary tuberculosis; (IX) history of mental disorders, auditory hallucinations, visual hallucinations, or taking antipsychotic drugs; (X) history of cognitive disorders, including dementia or cognitive disorders; (XI) history of high paraplegia; and (XII) pregnant or lactating women. Considering that some patients with asthma have a high risk of developing COPD, a previous diagnosis of asthma confirmed by a pulmonary physician and the presence or absence of airflow reversibility will not be an exclusion criterion (22,23).
Baseline and follow-up assessments
Table 1 shows a summary of the assessments in this study.
Table 1
Assessment | Visit 1 (baseline) | Visit 2 (12 months) | Visit 3 (24 months) | Visit 4 (36 months) |
---|---|---|---|---|
Anthropometric measurements | ||||
Height | √ | √ | √ | √ |
Weight | √ | √ | √ | √ |
Seated blood pressure | √ | √ | √ | √ |
Resting oxygen saturation | √ | √ | √ | √ |
Questionnaires | ||||
COPD epidemiological questionnaire | √ | √ | ||
Medical history | √ | √ | √ | √ |
Medications history | √ | √ | √ | √ |
mMRC dyspnea scale | √ | √ | √ | √ |
CAT | √ | √ | √ | √ |
CCQ | √ | √ | √ | √ |
Acute respiratory event/exacerbation assessment | √ | √ | √ | √ |
Pulmonary function | ||||
Pre-bronchodilator spirometry | √ | √ | √ | √ |
Post-bronchodilator spirometry | √ | √ | √ | √ |
Impulse oscillometry | √ | √ | √ | √ |
Chest CT | ||||
Inspiration | √ | |||
Expiration | √ | |||
Biomarkers | ||||
Blood samples | √ | √ | ||
Urine samples | √ | √ | ||
Induced sputuma | √ | √ | ||
Pollutant exposure measures | ||||
Satellite remote sensing/district and county based sampling | Daily monitoring | |||
Home monitoring/personal samplinga | √ | √ | √ | √ |
Exercise capacity | ||||
6MWTa | √ | √ | ||
CPETa | √ | √ |
a, subgroup A: completed by some participants. ECOPD study, Early Chronic Obstructive Pulmonary Disease study; COPD, chronic obstructive pulmonary disease; mMRC, modified British Medical Research Council; CAT, chronic obstructive pulmonary disease assessment test; CCQ, chronic obstructive pulmonary disease clinical questionnaire; CT, computed tomography; 6MWT, 6-minute walk test; CPET, cardiopulmonary exercise testing.
Questionnaires
A questionnaire interview will be performed by well-trained staff. The standard respiratory epidemiological questionnaire in this study has been modified from a COPD epidemiological study in China, and includes smoking status, smoking index, passive smoking, biomass exposure, history of occupational exposure to dust/gases/fumes, and family history of respiratory diseases (2,24). We classified participants’ smoking status as never smoked, former smoking, and current smoking (24). Never smoked was defined as having smoked a total of fewer than 100 cigarettes in the past. Current smoking was defined as smoking at baseline. Former smoking was defined as having previously smoked more than 100 cigarettes but not having smoked for at least 6 months prior to baseline. The smoking index was defined as the number of packs of cigarettes that were smoked daily, multiplied by the years of smoking. We defined passive smoking as inhalation of smoke by non-smokers who lived with smokers for more than 1 year. Biomass exposure was defined as cooking or heating using biomass (mainly wood, crop residues, charcoal, grass, and dung) for more than 1 year (21). History of occupational exposure to dust/gases/fumes was defined as having occupational exposure to dust/gases/fumes for more than 1 year over a participants’ lifetime. We defined family history of respiratory diseases as having parents, siblings, and children with respiratory diseases (chronic bronchitis, emphysema, asthma, COPD, cor pulmonale, bronchiectasis, lung cancer, interstitial lung disease, obstructive sleep apnea hypopnea syndrome). Medications and medical history will also be assessed at all visits.
Quality of life will be assessed at all visits using the mMRC dyspnea scale, CAT score, and CCQ score (25,26). Acute respiratory events or exacerbations are defined as the onset or worsening of at least two of the following symptoms: cough, sputum production, purulent sputum, wheezing, and dyspnea lasting for at least 48 h after excluding left and right heart dysfunction, pulmonary embolism, pneumothorax, pleural effusion, arrhythmia, and other diseases (27,28). Participants are to immediately contact investigators in the case of worsening respiratory symptoms. The duration, interval, severity, and management of acute respiratory events or exacerbations will be recorded and adjudicated by the investigators.
Spirometry
Spirometry will be performed by well-trained staff in accordance with the operational methods and quality control standards recommended by American Thoracic Society and Europe Respiratory Society (29,30). We will use a portable spirometer (CareFusion, Yorba Linda, CA, USA). At least three acceptable measurements and two reproducible tests (i.e., maximum and sub-maximum of FVC and FEV1 within 150 mL or 5%) will be conducted for each participant. We will record the maximum value of FVC and FEV1 and the maximal mid-expiratory flow, forced expiratory flow 50%, and forced expiratory flow 75% on the curve of FEV1 + FVC maximum value (30). We will administer a bronchodilator [salbutamol (Ventolin, GlaxoSmithKline) 400 µg] by inhalation through a 500 mL spacer and will repeat spirometry 20 min later, using the same criteria. The diagnosis of COPD and grading standard of lung function are recommended in the GOLD guidelines (1). Participants with post-bronchodilator FEV1/FVC <0.70 will be diagnosed with COPD. The pulmonary function classification of participants is divided according to the percentage of the predicted value of post-bronchodilator FEV1. We will use reference values of the European Coal and Steel Community 1993 for predicted values of FEV1, adjusted using conversion factors for the Chinese population (men, 0.95 and women, 0.93) (31,32).
IOS (Masterscreen IOS, Hochberg, Germany) will be performed according to European Respiratory Society guidelines (33). IOS is an effort-independent test performed during tidal breathing to measure the frequency dependence of sound waves emanating from the bronchial tree. The operator gently presses the participants’ cheeks with the hands to prevent cheek vibrations from affecting the accuracy of measurement. Participants breathe quietly through a loudspeaker-activated mouthpiece for 30–45 s without contraction of the glottis or interposition of the tongue. Respiratory resistance at 5 Hz (R5) and respiratory resistance 20 Hz (R20) are used as indicators of total airway resistance and central airway resistance, respectively. Resistance that drops from 5 to 20 Hz (R5–R20) is considered an indicator of resistance in the peripheral small airways (34). We will also record the following variables: reactance at 5 Hz, which reflects the elastic recoil of the small airways; resonance frequency, which is defined as the frequency at which the airway inertia is equal to the capacitance around the lung; and reactance area, which reflects the elasticity around the lung (35).
Imaging
High-resolution CT will be performed with each participant lying supine at suspended full inspiration and the deep end of expiration from the apex to the base of the lung using a multidetector-row CT scanner (Siemens Definition AS Plus 128-slicers and United-imaging uCT 760 128-slicers). The researchers will train each participant to hold their breath at the end of a deep inhalation and deep expiration to achieve the best condition before the scan. Two radiologists who are blinded to each participant’s pulmonary function will independently score each scan. The CT Assessment Scoresheet is shown in Supplementary (Appendix 1). The detailed CT scan protocol is shown in Table 2.
Table 2
Scanner make | Siemens | United-imaging |
---|---|---|
Scan model | Definition (AS Plus), 128-slices | uCT 760, 128-slices |
Scan type | Spiral | Spiral |
Scan FOV | Lungs | Lungs |
Rotation time, s | 0.5 | 0.5 |
Pitch | 1.1 | 1.1 |
kVp | 120 | 120 |
Inspiratory mAs | Effective mAs 200 | Effective mAs 200 |
Expiratory mAs | Effective mAs 50 | Effective mAs 50 |
Dose modulation | Care dose off | Care dose off |
Algorithm 1 | B35f | B_SOFT_B |
Algorithm 2 | B46f | B_SHARP_C |
Matrix | 512×512 | 512×512 |
Additional image filters | No selection | No selection |
Thickness, mm | 0.75 | 0.75 |
Interval, mm | 0.5 | 0.5 |
FOV, field of view.
Quantitative assessment of CT images will be performed using Chest Imaging Platform (https://www.chestimagingplatform.org) on the semi-automated 3D Slicer 4.11 software (https://www.slicer.org) (36). Emphysema is quantified by measuring the emphysema index of each patient, which is defined as the percentage of low-attenuation areas below −950 Hounsfield units (HU) on full-inspiration CT, and inspiratory 15th percentile, which is defined as the HU at which 15% of the voxels fall below at full inspiration (37). Air trapping is defined as the percentage of low-attenuation areas below −856 HU on full-expiration CT (37). We will also record the following variables: pectoralis muscle area, erector spinae muscle area, diameter of the pulmonary artery, and diameter of the aorta (38-40).
Exercise capacity
We will conduct a 6MWT in accordance with the American Thoracic Society guidelines (41). A CPET will be administered using a calibrated bicycle ergometer (Quark PFT Ergo Bp900, COSMED, Rome, Italy) to maximal tolerance, with the following procedure: 2 min of rest, 2 min of unloaded cycling at 55–65 rpm, stepwise increases in work load of 5–30 W/min at 55–65 rpm until symptom-limited fatigue (a total of approximately 8–12 min), then 10 min of recovery and 3 min of rest. Continuous breath-by-breath gas exchange and 12-lead electrocardiogram will be recorded. Measurements of exercise tolerance such as peak power output (), peak oxygen uptake (), and peak respiratory exchange ratio () will be obtained. Breathing reserve is calculated as estimated maximum voluntary ventilation (FEV1 ×40) minus peak minute ventilation (42-44). The lowest value of ventilatory equivalents for carbon dioxide (lowest VE/VCO2) calculated each minute during exercise can be used to determined ventilatory efficiency (45,46). Anaerobic threshold is determined using the V-slope method and the predicted values are determined according to the equations proposed by Wasserman and Hansen (47,48). Exercise restriction will be considered to be of the predicted value and maximal effort to be (49).
Biomarkers
Well-trained staff will collect and prepare biological samples from all participants, as follows. For peripheral blood collection, two inert separation gel procoagulant tubes are used to collect 3 mL of blood. Then, two ethylenediamine tetraacetic (EDTA) anticoagulant tubes are used to collect 3 mL of blood, after which the tubes are gently inverted three to five times. Finally, PAXgene Blood RNA tubes are used to collect 2.5 mL of blood, with gentle inversion (8–10 times) after collection.
The collected blood samples will be stored at room temperature for at least 30 min, and sample preparation will be completed within 8 h. One blood sample in an inert separation gel procoagulant tube and one sample in an EDTA anticoagulation tube will be sent to the clinical laboratory to detect relevant blood biochemical and blood routine indicators. The remaining inert separation gel procoagulant tube and EDTA anticoagulant tube are centrifuged at 3,000 rpm for 10 min. Serum is separated in the inert separation gel procoagulant tube and transferred to a labeled cryotube. The plasma and cell pellet are collected separately from the EDTA anticoagulation tube and transferred to labeled cryotubes. All samples will be stored at a temperature below −20 °C until transport. Blood collected in PAXgene Blood RNA tubes does not require sample preparation and will be stored at a temperature below −20 °C until transport.
Urine samples are to be collected by the participants themselves. Participants will each be provided with two 15 mL urine tubes without preservatives and one disposable urine cup marked with the participants’ information. Participants will be instructed to collect a midstream urine sample in the disposable cup, pour some of the urine into each of the two tubes, and then return the tubes to the researchers. One urine sample per participant will be sent to the clinical laboratory to test urine indicators. The collected urine samples will be stored at 4 °C; all samples should be prepared within 2 h. The tubes containing urine samples are to be inverted three times, mixed well, and transferred to labeled cryotubes. All prepared samples are stored at −20 °C until transport.
All cryotubes tubes containing samples will be stored on dry ice during transport to the biological material repository of the State Key Laboratory of Respiratory Diseases. After scanning the bar code, cryotubes will be stored in an ultra-low temperature freezer at −80 °C.
Before the induced sputum examination, participants will be asked to gargle and to rinse their mouth. The participants will inhale nebulized saline (3%) in three sequential 7-min inhalation periods using an ultrasonic nebulizer with an output of 1.5 mL/min. Participants will then be asked to expectorate sputum into a petri dish, and the quality of sputum will be evaluated under light microscopy at ×10 magnification. A sample will be considered adequate when the percentage of squamous cells is less than 20%. Qualified sputum plugs are selected and weighed. Sputum will be processed within 3 h of sampling for optimum cell viability. A volume of phosphate-buffered saline (PBS) containing 0.2% dithiothreitol will be used to digest sputum. Portions of the sputum samples are agitated using a vortex, placed on ice for 15 min, placed on a bench rocker for 5 min, filtered through a 70-µm Falcon cell strainer, and centrifuged at 3,000 rpm and 4 °C for 10 min. The sputum supernatant is then discarded. The cell pellet is resuspended in 1,000 µL PBS, and 30 µL of the cell suspension is taken for hematoxylin-eosin staining of cell smears. On average, two slides will be prepared for each patient. The sample is placed in an incubator at 65 °C for 20 min and then sealed with resinene. At least 400 non-squamous cells (neutrophils, eosinophils, macrophages, lymphocytes, and epithelial cells) should be counted under light microscopy. Absolute values and percentages of each cell type will be recorded.
Pollutant exposure measures
The primary pollutants of interest in this study are fine and inhalable particulate matter (PM2.5, PM10, respectively), ozone, sulfur dioxide, nitrogen dioxide, and carbon monoxide. We aim to examine the impacts of long-term exposure to air pollution owing to ambient, cooking, and traffic pollutants on the development and progression of airflow obstruction. To estimate personal exposure, all participants will be required to complete a questionnaire at every visit. Information will be collected on their current home address, predictors of specific infiltration efficiencies (Finf), time-location patterns, cooking conditions, fuel use, and tobacco smoke exposure. To access historical exposure, we will use data from remote satellite platforms to calculate long-term estimates of PM and O3. The resolution of estimation is at a 3×3-km grid for each home address. We will use existing administrative monitoring (i.e., maintained by a government agency) and the concentrations of both PM and gases will be monitored continually during the study period. Several approaches are available that use such data: nearest monitor, inverse distance weighting, and land use regression. No fewer than 25 homes per district/county will be selected to carry out outdoor and indoor monitoring. Outdoor sampling is often conducted in the open space outside each home and indoor sampling is carried out in the main room of the same building. Paired outdoor-indoor sampling and corresponding predictors (e.g., number of windows and percent of open windows) are used to establish a Finf prediction model for each district/county. A subset of the study population will be selected for personal sampling. Portable monitors will be used to monitor personal exposure for 3 days during each season. The elemental and organic composition of PM will be analyzed using X-ray fluorescence, ion chromatography, and pre-fired quartz fiber filters.
Data collection and management
All raw data will be recorded and collected using an electronic data capture system. Data verification and consistency checks will be performed on locked data by the data management center to ensure data quality. After review, editing, and checking, the data are to be locked to prevent further manipulation.
Statistical analysis
We have ensured a sufficient sample size to ensure that our research goals about pre-COPD achieved (annual decline in FEV1 and development of airflow obstruction). To detect the difference of 15 [standard deviation (SD) 80] mL per year in FEV1 between high-risk group and non-high-risk group with 80% power, a two-sided type I error of 5%, a loss of follow-up rate of about 15%, we calculated that we needed about 1,000 participants with normal spirometry. This study is exploratory for early COPD because related research is still limited and controversial. Therefore, we will recruit approximately 1,000 COPD participants (800 with GOLD stage 1–2 and 200 with GOLD stage 3–4) to ensure that our research goals achieved (annual decline in FEV1). Sample size calculation were conducted with the use of PASS 15 software (NCSS, Kaysville, UT, USA).
We will use frequency and percentage for comparisons between groups. Data with a normal distribution are expressed as mean ± SD. Data with a non-normal distribution are expressed as median (interquartile range). We will use a two-sample t-test, Wilcoxon rank-sum test, chi-square test, and Fisher’s exact test to evaluate differences between two groups, as appropriate. We will apply a mixed-effects model, which admits missing values, to assess longitudinal changes in FEV1, FVC, and FEV1/FVC between groups, after adjustment for confounders. The frequency and number of acute respiratory events or exacerbations in COPD will be compared using a Poisson regression model, with correction for confounders. Moreover, we will use machine learning algorithms (including random forest, neural network, gradient boosting, and support vector machines methods) to establish individualized prediction models for predicting development of airflow obstruction and disease progression. Statistical analyses will be conducted with IBM SPSS 24.0 software (IBM Corp., Armonk, NY, USA) and SAS 9.1 software (SAS Institute, Cary, NC, USA). P<0.05 will be set to indicate statistical significance.
Ethics and dissemination
The study was approved by the Ethics Committee of The First Affiliated Hospital of Guangzhou Medical University (No. 2018-53), and written informed consent will be obtained from all patients. The study findings will be presented at conferences and will be reported in peer-reviewed journals.
Discussion
To date, little evidence is available regarding which characteristics are more likely to be present in individuals who develop airflow obstruction or regarding clinically relevant early COPD subtypes. To our knowledge, this is the first prospective observational cohort study that aims to address the above evidence gap in China, focusing on individuals who are asymptomatic and never smokers, to identify early COPD.
The currently definition for a diagnosis of COPD, that is, post-bronchodilator FEV1/FVC <0.7, is controversial. A National Heart, Lung, and Blood Institute pooled cohorts study found that FEV1/FVC <0.7 showed no significant difference in discrimination of COPD-related hospitalization and mortality compared with FEV1/FVC < lower limits of normal (LLN) (50). FEV1/FVC <0.7 is used to diagnose airflow obstruction in clinical practice because of convenience. However, only using FEV1/FVC <0.7 to diagnose COPD will miss some individuals who have respiratory symptoms or imaging abnormalities (emphysema, small airway disease, and airway remodeling) but who do not meet the diagnostic criteria for airflow obstruction. These individuals have a faster rate of decline in lung function and higher all-cause mortality (51). Lowe and colleagues proposed combining respiratory symptoms, abnormal CT, and abnormal lung function to diagnose COPD, but this has not yet been adopted in the GOLD guidelines (51). Considering that no other comprehensive diagnostic methods for COPD are available, in this study, we will initially use post-bronchodilator FEV1/FVC <0.7 to diagnose airflow obstruction. The follow-up data in this study will be used to further explore the ability of combining respiratory symptoms, CT abnormalities, and lung function abnormalities to diagnose COPD and predict long-term prognosis.
From GOLD 0 to pre-COPD, a concern of the COPD research community has been which clinical characteristics are present that increase the likelihood of developing airflow obstruction, consistent with a diagnosis of COPD (7,8). Previous studies have found that people with nonobstructive chronic bronchitis (10,52), emphysema (53,54), small airway disease (55), airway remodeling (53,56), preserved ratio impaired spirometry (57,58), early history of asthma (22), low diffusion capacity for carbon monoxide (59), and accelerated FEV1 decline (60) are more likely to progress to airflow obstruction. However, some research findings are inconsistent, possibly because of different research designs, different smoking exposure levels, and inconsistent definitions. Nonetheless, currently, no relevant research data are available on high-risk groups for COPD in China. Future studies identifying the characteristics, parameters, and biomarkers that may predict development of airflow obstruction and annual decline in lung function with normal spirometry are required. Longitudinal investigation of normal spirometry and early COPD in the ECOPD study will provide new insights into the natural history and epidemiology of COPD.
Controversy remains surrounding the definition of early COPD. Previously, our research team used the definition of early-stage COPD, which is GOLD stage 1–2 (mild and moderate) (28). Recently, Martinez and colleagues proposed the following method for early COPD diagnosis: individuals younger than age 50 years with a smoking history of 10 or more pack-years and any of the following abnormalities: (I) post-bronchodilator FEV1/FVC < LLN; (II) compatible CT abnormalities; and (III) accelerated FEV1 decline (>60 mL/year) (6). This new diagnostic method would not include 20–40% of non-smokers with COPD, and it also excludes half of adult patients with COPD who develop the disease without evidence of accelerated lung function decline; therefore, this method has limited clinical utility (21,61). Early-stage COPD is a mild disease, and Martinez and colleagues have proposed a diagnostic method for early disease. There are obvious differences between the two. More research is needed to further explore methods for early COPD diagnosis, prevalence, clinical characteristics, and longitudinal prognosis. It is worth noting that the 3-year follow-up time in this study may not be enough. Therefore, if necessary, we may extend the follow-up time according to the actual situation and the wishes of the subjects.
Increases in computing power and increased data availability have enabled artificial intelligence and machine learning techniques to be used successfully in respiratory medicine (62). For instance, convolutional neural networks based on CT images can be used to detect and stage COPD and predict acute respiratory events and mortality (63). A random survival forest model could outperform body mass index, airflow obstruction, dyspnea, exercise capacity index, age, dyspnea, and the airflow obstruction index in predicting all-cause mortality (64). In the ECOPD study, we will also use deep learning and artificial intelligence methods to establish COPD diagnosis and disease progression prediction models for better individualized and precise prevention, management, and follow-up.
Conclusions
The ECOPD study will provide an opportunity to explore which characteristics are more likely to be present in those individuals who develop airflow obstruction, as well as clinically related early COPD subtypes, for closer patient follow-up and management. The data gathered may not only shed new light on early COPD subtypes and prediction of long-term disease progression but may also provide a basis for early detection of COPD. At present, recruitment and follow-up in this study is ongoing.
Acknowledgments
We thank the medical staff of The First Affiliated Hospital of Guangzhou Medical University (Qingsi Zeng, Yu Deng, Huai Chen and Xiaoyan Huang), Lianping County People’s Hospital (Xiangwen Luo and Shuqing Yu), and Wengyuan County People’s Hospital (Changli Yang and Shengtang Chen) for their assistance in conducting this study. We thank Bijia Lin, Shaodan Wei, Xiaopeng Ling, Wenjun Lai, Qiaoyi He, and Yunsong Chen (Nanshan Medical Development Foundation of Guangdong Province, National Center for Respiratory Medicine, State Key Laboratory of Respiratory Disease, National Clinical Research Center for Respiratory Disease, Guangzhou Institute of Respiratory Health, The First Affiliated Hospital of Guangzhou Medical University) for their efforts in collecting the information and verification.
Funding: This study was supported by the National Key Research and Development Program (2016YFC1304101), the Local Innovative and Research Teams Project of Guangdong Pearl River Talents Program (2017BT01S155), the National Natural Science Foundation of China [81970045], and Zhong Nanshan Medical Foundation of Guangdong Province (ZNSA-202003, ZNSA-202012, ZNSA-2020013).
Footnote
Reporting Checklist: The authors have completed the SPIRIT reporting checklist. Available at https://dx.doi.org/10.21037/jtd-21-1379
Conflicts of Interest: All authors have completed the ICMJE uniform disclosure form (available at https://dx.doi.org/10.21037/jtd-21-1379). The authors have no conflicts of interest to declare.
Ethical Statement: The authors are accountable for all aspects of the work in ensuring that questions related to the accuracy or integrity of any part of the work are appropriately investigated and resolved. The study was approved by the Ethics Committee of The First Affiliated Hospital of Guangzhou Medical University (No. 2018-53), and written informed consent will be obtained from all patients. The study findings will be presented at conferences and will be reported in peer-reviewed journals.
Open Access Statement: This is an Open Access article distributed in accordance with the Creative Commons Attribution-NonCommercial-NoDerivs 4.0 International License (CC BY-NC-ND 4.0), which permits the non-commercial replication and distribution of the article with the strict proviso that no changes or edits are made and the original work is properly cited (including links to both the formal publication through the relevant DOI and the license). See: https://creativecommons.org/licenses/by-nc-nd/4.0/.
References
- Global Strategy for the Diagnosis, Management, and Prevention of Chronic Obstructive Lung Disease: 2021 report, 2021. Available online: https://goldcopd.org/2021-gold-reports/
- Zhong N, Wang C, Yao W, et al. Prevalence of chronic obstructive pulmonary disease in China: a large, population-based survey. Am J Respir Crit Care Med 2007;176:753-60. [Crossref] [PubMed]
- Wang C, Xu J, Yang L, et al. Prevalence and risk factors of chronic obstructive pulmonary disease in China (the China Pulmonary Health [CPH] study): a national cross-sectional study. Lancet 2018;391:1706-17. [Crossref] [PubMed]
- Mathers CD, Loncar D. Projections of global mortality and burden of disease from 2002 to 2030. PLoS Med 2006;3:e442. [Crossref] [PubMed]
- Celli BR, Wedzicha JA. Update on Clinical Aspects of Chronic Obstructive Pulmonary Disease. N Engl J Med 2019;381:1257-66. [Crossref] [PubMed]
- Martinez FJ, Han MK, Allinson JP, et al. At the Root: Defining and Halting Progression of Early Chronic Obstructive Pulmonary Disease. Am J Respir Crit Care Med 2018;197:1540-51. [Crossref] [PubMed]
- Han MK, Agusti A, Celli BR, et al. From GOLD 0 to Pre-COPD. Am J Respir Crit Care Med 2021;203:414-23. [Crossref] [PubMed]
- Pauwels RA, Buist AS, Calverley PM, et al. Global strategy for the diagnosis, management, and prevention of chronic obstructive pulmonary disease. NHLBI/WHO Global Initiative for Chronic Obstructive Lung Disease (GOLD) Workshop summary. Am J Respir Crit Care Med 2001;163:1256-76. [Crossref] [PubMed]
- Balte PP, Chaves PHM, Couper DJ, et al. Association of Nonobstructive Chronic Bronchitis With Respiratory Health Outcomes in Adults. JAMA Intern Med 2020;180:676-86. [Crossref] [PubMed]
- Allinson JP, Hardy R, Donaldson GC, et al. The Presence of Chronic Mucus Hypersecretion across Adult Life in Relation to Chronic Obstructive Pulmonary Disease Development. Am J Respir Crit Care Med 2016;193:662-72. [Crossref] [PubMed]
- Vestbo J, Lange P. Can GOLD Stage 0 provide information of prognostic value in chronic obstructive pulmonary disease? Am J Respir Crit Care Med 2002;166:329-32. [Crossref] [PubMed]
- Çolak Y, Nordestgaard BG, Vestbo J, et al. Prognostic significance of chronic respiratory symptoms in individuals with normal spirometry. Eur Respir J 2019;54:1900734. [Crossref] [PubMed]
- Regan EA, Hokanson JE, Murphy JR, et al. Genetic epidemiology of COPD (COPDGene) study design. COPD 2010;7:32-43. [Crossref] [PubMed]
- Couper D, LaVange LM, Han M, et al. Design of the Subpopulations and Intermediate Outcomes in COPD Study (SPIROMICS). Thorax 2014;69:491-4. [Crossref] [PubMed]
- Bourbeau J, Tan WC, Benedetti A, et al. Canadian Cohort Obstructive Lung Disease (CanCOLD): Fulfilling the need for longitudinal observational studies in COPD. COPD 2014;11:125-32. [Crossref] [PubMed]
- Labarca G, Bustamante A, Valdivia G, et al. The boundaries of mild chronic obstructive pulmonary disease (COPD): design of the searching clinical COPD onset (SOON) study. BMJ Open 2017;7:e015731. [Crossref] [PubMed]
- Crapo J, Gupta A, Lynch DA, et al. FOOTPRINTS study protocol: rationale and methodology of a 3-year longitudinal observational study to phenotype patients with COPD. BMJ Open 2021;11:e042526. [Crossref] [PubMed]
- Vrbica Ž, Labor M, Gudelj I, et al. Early detection of COPD patients in GOLD 0 population: an observational non-interventional cohort study - MARKO study. BMC Pulm Med 2017;17:36. [Crossref] [PubMed]
- Salvi SS, Barnes PJ. Chronic obstructive pulmonary disease in non-smokers. Lancet 2009;374:733-43. [Crossref] [PubMed]
- Siddharthan T, Gupte A, Barnes PJ. Chronic Obstructive Pulmonary Disease Endotypes in Low- and Middle-Income Country Settings: Precision Medicine for All. Am J Respir Crit Care Med 2020;202:171-2. [Crossref] [PubMed]
- Zhou Y, Wang C, Yao W, et al. COPD in Chinese nonsmokers. Eur Respir J 2009;33:509-18. [Crossref] [PubMed]
- Asamoah-Boaheng M, Acheampong L, Tenkorang EY, et al. Association between early history of asthma and COPD diagnosis in later life: a systematic review and meta-analysis. Int J Epidemiol 2018;47:1865-76. [Crossref] [PubMed]
- Morgan BW, Grigsby MR, Siddharthan T, et al. Epidemiology and risk factors of asthma-chronic obstructive pulmonary disease overlap in low- and middle-income countries. J Allergy Clin Immunol 2019;143:1598-606. [Crossref] [PubMed]
- Zhou Y, Hu G, Wang D, et al. Community based integrated intervention for prevention and management of chronic obstructive pulmonary disease (COPD) in Guangdong, China: cluster randomised controlled trial. BMJ 2010;341:c6387. [Crossref] [PubMed]
- Jones PW, Harding G, Berry P, et al. Development and first validation of the COPD Assessment Test. Eur Respir J 2009;34:648-54. [Crossref] [PubMed]
- van der Molen T, Willemse BW, Schokker S, et al. Development, validity and responsiveness of the Clinical COPD Questionnaire. Health Qual Life Outcomes 2003;1:13. [Crossref] [PubMed]
- Anthonisen NR, Manfreda J, Warren CP, et al. Antibiotic therapy in exacerbations of chronic obstructive pulmonary disease. Ann Intern Med 1987;106:196-204. [Crossref] [PubMed]
- Zhou Y, Zhong NS, Li X, et al. Tiotropium in Early-Stage Chronic Obstructive Pulmonary Disease. N Engl J Med 2017;377:923-35. [Crossref] [PubMed]
- Miller MR, Crapo R, Hankinson J, et al. General considerations for lung function testing. Eur Respir J 2005;26:153-61. [Crossref] [PubMed]
- Miller MR, Hankinson J, Brusasco V, et al. Standardisation of spirometry. Eur Respir J 2005;26:319-38. [Crossref] [PubMed]
- Quanjer PH, Tammeling GJ, Cotes JE, et al. Lung volumes and forced ventilatory flows. Report Working Party Standardization of Lung Function Tests, European Community for Steel and Coal. Official Statement of the European Respiratory Society. Eur Respir J Suppl 1993;16:5-40. [Crossref] [PubMed]
- Zheng J, Zhong N. Normative values of pulmonary function testing in Chinese adults. Chin Med J (Engl) 2002;115:50-4. [PubMed]
- Oostveen E, MacLeod D, Lorino H, et al. The forced oscillation technique in clinical practice: methodology, recommendations and future developments. Eur Respir J 2003;22:1026-41. [Crossref] [PubMed]
- Lipworth B, Manoharan A, Anderson W. Unlocking the quiet zone: the small airway asthma phenotype. Lancet Respir Med 2014;2:497-506. [Crossref] [PubMed]
- King GG, Bates J, Berger KI, et al. Technical standards for respiratory oscillometry. Eur Respir J 2020;55:1900753. [Crossref] [PubMed]
- Fedorov A, Beichel R, Kalpathy-Cramer J, et al. 3D Slicer as an image computing platform for the Quantitative Imaging Network. Magn Reson Imaging 2012;30:1323-41. [Crossref] [PubMed]
- Labaki WW, Martinez CH, Martinez FJ, et al. The Role of Chest Computed Tomography in the Evaluation and Management of the Patient with Chronic Obstructive Pulmonary Disease. Am J Respir Crit Care Med 2017;196:1372-9. [Crossref] [PubMed]
- Tanimura K, Sato S, Fuseya Y, et al. Quantitative Assessment of Erector Spinae Muscles in Patients with Chronic Obstructive Pulmonary Disease. Novel Chest Computed Tomography-derived Index for Prognosis. Ann Am Thorac Soc 2016;13:334-41. [Crossref] [PubMed]
- McDonald ML, Diaz AA, Ross JC, et al. Quantitative computed tomography measures of pectoralis muscle area and disease severity in chronic obstructive pulmonary disease. A cross-sectional study. Ann Am Thorac Soc 2014;11:326-34. [Crossref] [PubMed]
- Wells JM, Washko GR, Han MK, et al. Pulmonary arterial enlargement and acute exacerbations of COPD. N Engl J Med 2012;367:913-21. [Crossref] [PubMed]
- ATS Committee on Proficiency Standards for Clinical Pulmonary Function Laboratories. ATS statement: guidelines for the six-minute walk test. Am J Respir Crit Care Med 2002;166:111-7. [Crossref] [PubMed]
- Hansen JE, Sue DY, Wasserman K. Predicted values for clinical exercise testing. Am Rev Respir Dis 1984;129:S49-55. [Crossref] [PubMed]
- Sue DY, Hansen JE. Normal values in adults during exercise testing. Clin Chest Med 1984;5:89-98. [Crossref] [PubMed]
- Campbell SC. A comparison of the maximum voluntary ventilation with the forced expiratory volume in one second: an assessment of subject cooperation. J Occup Med 1982;24:531-3. [PubMed]
- Sun XG, Hansen JE, Garatachea N, et al. Ventilatory efficiency during exercise in healthy subjects. Am J Respir Crit Care Med 2002;166:1443-8. [Crossref] [PubMed]
- Myers J, Arena R, Oliveira RB, et al. The lowest VE/VCO2 ratio during exercise as a predictor of outcomes in patients with heart failure. J Card Fail 2009;15:756-62. [Crossref] [PubMed]
- Sue DY, Wasserman K, Moricca RB, et al. Metabolic acidosis during exercise in patients with chronic obstructive pulmonary disease. Use of the V-slope method for anaerobic threshold determination. Chest 1988;94:931-8. [Crossref] [PubMed]
- Wasserman K, Hansen JE, Sue DY, Stringer WW, Whipp BJ. Principles of Exercise Testing and Interpretation. 4th edition. Philadelphia, PA, USA: Lippincott Williams and Wilkins, 2004.
- Guazzi M, Adams V, Conraads V, et al. EACPR/AHA Scientific Statement. Clinical recommendations for cardiopulmonary exercise testing data assessment in specific patient populations. Circulation 2012;126:2261-74. [Crossref] [PubMed]
- Bhatt SP, Balte PP, Schwartz JE, et al. Discriminative Accuracy of FEV1:FVC Thresholds for COPD-Related Hospitalization and Mortality. JAMA 2019;321:2438-47. [Crossref] [PubMed]
- Lowe KE, Regan EA, Anzueto A, et al. COPDGene® 2019: Redefining the Diagnosis of Chronic Obstructive Pulmonary Disease. Chronic Obstr Pulm Dis 2019;6:384-99. [Crossref] [PubMed]
- Guerra S, Sherrill DL, Venker C, et al. Chronic bronchitis before age 50 years predicts incident airflow limitation and mortality risk. Thorax 2009;64:894-900. [Crossref] [PubMed]
- Mohamed Hoesein FA, de Jong PA, Lammers JW, et al. Airway wall thickness associated with forced expiratory volume in 1 second decline and development of airflow limitation. Eur Respir J 2015;45:644-51. [Crossref] [PubMed]
- Oh AS, Strand M, Pratte K, et al. Visual Emphysema at Chest CT in GOLD Stage 0 Cigarette Smokers Predicts Disease Progression: Results from the COPDGene Study. Radiology 2020;296:641-9. [Crossref] [PubMed]
- Stockley JA, Ismail AM, Hughes SM, et al. Maximal mid-expiratory flow detects early lung disease in α1-antitrypsin deficiency. Eur Respir J 2017;49:1602055. [Crossref] [PubMed]
- Smith BM, Kirby M, Hoffman EA, et al. Association of Dysanapsis With Chronic Obstructive Pulmonary Disease Among Older Adults. JAMA 2020;323:2268-80. [Crossref] [PubMed]
- Wan ES, Fortis S, Regan EA, et al. Longitudinal Phenotypes and Mortality in Preserved Ratio Impaired Spirometry in the COPDGene Study. Am J Respir Crit Care Med 2018;198:1397-405. [Crossref] [PubMed]
- Wijnant SRA, De Roos E, Kavousi M, et al. Trajectory and mortality of preserved ratio impaired spirometry: the Rotterdam Study. Eur Respir J 2020;55:1901217. [Crossref] [PubMed]
- Harvey BG, Strulovici-Barel Y, Kaner RJ, et al. Risk of COPD with obstruction in active smokers with normal spirometry and reduced diffusion capacity. Eur Respir J 2015;46:1589-97. [Crossref] [PubMed]
- Petersen H, Sood A, Polverino F, et al. The Course of Lung Function in Middle-aged Heavy Smokers: Incidence and Time to Early Onset of Chronic Obstructive Pulmonary Disease. Am J Respir Crit Care Med 2018;198:1449-51. [Crossref] [PubMed]
- Agusti A, Faner R. How to Define Early Chronic Obstructive Pulmonary Disease. Am J Respir Crit Care Med 2018;198:973. [Crossref] [PubMed]
- Gonem S, Janssens W, Das N, et al. Applications of artificial intelligence and machine learning in respiratory medicine. Thorax 2020;75:695-701. [Crossref] [PubMed]
- González G, Ash SY, Vegas-Sánchez-Ferrero G, et al. Disease Staging and Prognosis in Smokers Using Deep Learning in Chest Computed Tomography. Am J Respir Crit Care Med 2018;197:193-203. [Crossref] [PubMed]
- Moll M, Qiao D, Regan EA, et al. Machine Learning and Prediction of All-Cause Mortality in COPD. Chest 2020;158:952-64. [Crossref] [PubMed]