COX-2-related tumor immune microenvironment in non-small cell lung cancer: a novel signature to predict hot and cold tumor
Introduction
Lung cancer remains the leading cause of malignant tumor mortality worldwide (1). Non-small cell lung cancer (NSCLC) accounts for approximately 85% of all lung cancer cases, and is divided into three types: adenocarcinoma, squamous cell carcinoma, and large cell carcinoma (2), of which adenocarcinoma and squamous cell carcinoma are the most prevalent. Despite the continuous improvement in the tertiary prevention of lung cancer, the 5-year overall survival (OS) for NSCLC is still not ideal, especially for patients with late stage disease (less than 10%) (3). With the support of next-generation sequencing technology, some of molecular subtypes have been classified, facilitating the progress of clinical application of targeted therapy and immunotherapy.
Immunotherapy is a method of immune system activation to fight and efficiently remove tumor cells (4). Immune checkpoint inhibitors (ICIs) are most important immunotherapeutic modalities, and have offered a novel path for the treatment of NSCLC. ICIs are already being applied in first-line NSCLC therapy, and are generally combined with chemotherapy and radiotherapy (3). The most popular ICIs are a series of programmed cell death protein 1/programmed cell death 1 ligand 1 (PD-1/PD-L1) inhibitors, which block the PD-1/PD-L1 pathway between tumor cells and T cells, and activate infiltrating T cells to attack tumor cells (5). However, not all NSCLC patients benefit from ICIs treatment. Despite the fact that several ICI therapy-related clinical studies have found that patients with high PD-1 expression can benefit from ICI treatment, some patients with high PD-1 expression still do not benefit from PD-1 inhibitors. T cell exhaustion may be the important reason. When CD8+ T cell is under the condition of exhaustion, even with positive PD-1 expression, it does not have ability to eliminate the tumor cells (6). Likewise, it is found that PD-L1 is translated inside the nuclear having a new function to promote the tumorigenesis but not binding on the surface of cellular membrane (7). It explains that a part of patients have high PD-1/PD-L1 expression but have little response to the PD-1/PD-L1 inhibitor.
The tumor microenvironment (TME) is an internal environment where tumor cells reside; it is extremely complex and contains various tumor-related and immune cells, which constitute the entire microenvironment in tumor tissue, and involves interaction between different cell types (8). However, the mechanism underlying the low response to ICI treatment remains unclear. The TME in different NSCLC patients is not identical, which may explain patients undergoing ICI treatment have diverse therapeutic outcomes. Additionally, the tumor immune microenvironment (TIME) is correlated with tumor immunotherapy. Various immune cells in the TME are not identical, which suggests that the response to ICI treatment is also correlated to the TIME.
Cyclooxygenase-2 (COX-2) is associated with inflammation-related diseases, such as cancer. COX-2 is secreted by cancer-associated fibroblasts (CAFs), macrophage type 2 cells, and cancer cells in the TME, and have the ability to promote an inflammatory response in the local environment and induce tumor progression, metastasis, and drug resistance (9). COX-2 inhibitors have long been used for pain and to reduce inflammation, but evidence regarding the effectiveness of COX-2 inhibitors in anti-tumor therapy has emerged (10). However, in TME the mechanism of COX-2 influencing the PD-1/PD-L1 therapy is unclear. It is necessary to dig out the relationship between COX-2 and TME to help us deeply understand how COX-2 play a biological role in TME.
In this study, we relied on the RNA-seq data and used bioinformatics analysis to find out a novel diagnostic signature of hot and cold tumor and a potential gene target may have relations between each subtype. We integrated lung adenocarcinoma (LUAD) and lung squamous cell carcinoma (LUSC) RNA sequencing (RNA-seq) dataset from The Cancer Genome Atlas (TCGA) database as the NSCLC dataset. Tumor infiltrating immune cells were calculated through the CIBERSORT algorithm and the result was classified by the machine learning method to distinguish the hot and cold tumor subtypes which have different response to ICIs therapy. Additionally, we categorized the subtypes into hot and cold tumor groups, and performed an in-depth analysis the different genes between these two types to screen for novel diagnostic signatures of ICI therapy and mine hub genes for further study. We present the following article in accordance with the TRIPOD reporting checklist (available at https://jtd.amegroups.com/article/view/10.21037/jtd-22-257/rc).
Methods
In this study, we collected the LUAD and LUSC RNA-seq and clinical data from TCGA database. CIBERSORT algorithm used to estimate the immune cell infiltration, then we used infiltration data to classified the hot and cold tumor subtypes. Weighted correlation network analysis (WGCNA) was used to recognize the genes related to the hot and cold tumor. LASSO, SVM and GMM statistic methods were used to calculate the hot and cold tumor diagnostic signature. Kaplan-Meier analysis, GO and KEGG enrichment were used to compute the hot and cold tumor OS and potential pathway. The study was conducted in accordance with the Declaration of Helsinki (as revised in 2013).
Data collection and treatment
The RNA-seq dataset and clinical data of 485 LUAD and 473 LUSC samples from TCGA database were downloaded from University of California-Santa Cruz (UCSC) Xena website (URL: https://xena.ucsc.edu/). The LUAD and LUSC datasets were combined into NSCLC data, samples without clinical data matched were excluded. Gene expression in the RNA-seq matrix was transformed into Transcripts per million (TPM) from count. The approximate number of tumor infiltrating immune cells was calculated by CIBERSORT algorithm (R script v1.03, Aaron M. Newman, Stanford University). The script and signature matrix file, LM22, was downloaded from the CIBERSORT website (URL: http://CIBERSORT.stanford.edu/). The RNA-seq matrix input was changed to log2(TPM+1). Following calculation by CIBERSORT, each sample was found to have infiltration level of 22 types of immune cells. A method of unsupervised machine learning, R package ConsensusClusterPlus, was utilized to classify the subtypes from all samples in the NSCLC data. The ConsensusClusterPlus was employed based on the following conditions: maxK =20, reps =20, pItem =0.8, and pFeature =1.
Features extraction for predicting the tumor immune subtype
WGCNA was performed using the WGCNA package to analyze the correlation between hub gene modules and clinical features. The Glmnet package (Jerome Friedman, Trevor Hastie, Robert Tibshirani) was used for least absolute shrinkage and selection operator (LASSO) regression analysis of the variable selection and regularization, in order to screen for predictors in the gene expression matrix. Supervised machine learning was used to identify the genes diagnosis the hot or cold tumor, which was computed by Support Vector Machine (SVM) algorithm. Package of e1071 (David Meyer, Evgenia Dimitriadou, Kurt Hornik, Andreas Weingessel and Friedrich Leisch, TU Wien) and msvmRFE.r program (John Colby) were applied to realize the SVM algorithm. To find the predicted signatures of the tumor immune subtypes, the Gaussian Mixture Model (GMM) was used to calculate the best performing group of genes, after screening by the SVM.
Drug response
To identify the potential anti-tumor drugs in hot and cold tumors, the signature genes were applied to the Genomics of Drug Sensitivity in Cancer (GDSC) database, to compare the areas under the curve (AUCs) of drug response clusters in hot and cold tumors.
Pathway enrichment
The Database for Annotation, Visualization, and Integrated Discovery (DAVID) v6.8 (URL: https://david.ncifcrf.gov/) was applied to enrich the Kyoto Encyclopedia of Genes and Genomes (KEGG) and Gene Ontology (GO) hub gene-related pathways. Program R (R Foundation for Statistical Computing, Vienna, Austria) was used to organize the enrichment result from DAVID and draw the diagrams. Gene Set Variation Analysis (GSVA) was used to estimate the gene set enrichment variation and calculate the relationship between pathways.
Statistical analyses and diagram plots
The Kruskal-Wallis test was used to compare the gene expression between each group of samples. The AUC was used to calculate the predictable score for binary data, the acceptable AUC threshold values is 0.5. The Spearman test was applied to estimate the correlation coefficients between genes or pathways. OS analysis was based on the Kaplan-Meier (KM) statistical method. P<0.05 was considered to indicate a statistically significant difference. All the plots were draw by ggplot package (H. Wickham, Springer-Verlag, New York, USA). All of statistical analyses and plots were based on Program R (V4.03 and V4.10).
Results
TIME in NSCLC
The RNA-seq data of NSCLC, including 485 LUAD and 473 LUSC from TCGA database. NSCLC RNA-seq matrix was used to calculate the composition of 22 types of immune cells in each sample through CIBERSORT algorithm (Figure 1A,1B). Based on the infiltration of 22 types of immune cells, the NSCLC samples were clustered via consensus clustering, which is a method of unsupervised machine learning. According to the cumulative distribution functions (CDF) and area under the CDF curve, when K=6, the area of both of two plots reached an approximate maximum (Figure 1C,1D). Then we check the cluster assignment of columns, cluster in K=6 was indicative of unstable membership (Figure 1E). It suggests that when parameter K=6, consensus and cluster are confidence. The consensus matrix, which was comprised of six clusters, was extracted for further analysis (Figure 1F).
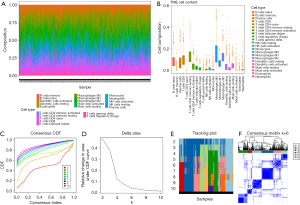
COX-2 associated with hot and cold tumor
According to the infiltration of cluster of differentiation 8 positive (CD8+) T cells and the expression of PD-1/PD-L1 in these six clusters, three ICI-related subtypes of NSCLC were identified (Figure 2A-2C). Also, three immunophenotypes were observed as immune-inflamed, immune-excluded, and immune deserted, respectively, based on the spatial quantification of CD8+ T cells in the tumor tissue (11). Cluster 2 had high-level infiltration of CD8+ T cells and high PD-1/PD-L1 expression, and was classified as an immune-inflamed type. Clusters 1 and 6 with medium infiltration of CD8+ T cells, but low PD-1/PD-L1 expression, were classified as immune-excluded types. The remaining clusters, which exhibited low-level infiltration of CD8+ T cells and low PD-1/PD-L1 expression, were classified as the immune desert type. Pre-clinical evidence has suggested that COX-2 inhibitors combining with ICIs can promote anti-tumor T-cell activation (12). Prostaglandin-endoperoxide synthase 2 (PTGS2, aliases of COX-2) expression is lower in the immune-inflamed type than in the other types, whereas PTGS2 expression is non-differentiated in the other two types (Figure 2D). It has been reported that overexpression of the COX-2 gene inhibits dendritic cell maturation, resulting lower anti-tumor CD8+ T cell infiltration. The results of our study are highly consistent with previous research. The Kaplan-Meier OS between three the immune subtypes (immune-desert type median OS =44.8 months, immune-exclude type median OS =57.5 months, immune-inflamed type median OS =66.1 months) in NSCLC were not significantly different (log rank P=0.1, two tailed test) (Figure 2E), which could be due to the fact that the TCGA clinical data does not include the ICI intervention history.
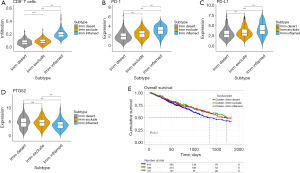
Hub genes identified through WGCNA
Based on the aforementioned result, we decided to combine the immune-excluded type and the immune desert type as cold tumors, and defined the immune-inflamed type as a hot tumor. Compared to hot tumors, the response rate of patients with cold tumors is relatively low (13). To determine the differential gene profiles between cold and hot tumors, WGCNA was employed to assess the gene expression profiles of both types. Firstly, we cleaned the data by excluding outlier samples (Figure 3A). In the network topology analysis, we selected the power 3 for soft-thresholding, which produced an output of 20 modules of different genes after network analysis (Figure 3B,3C). In 20 modules, consider the correlation coefficient of cold tumor (R=0.2, P<0.001), hot tumor (R=−0.2, P<0.001) and COX-2 expression (R=0.26, P<0.001), the greenyellow module is most relevant to the clinical traits (Figure 3D). In the greenyellow module, 924 genes were chosen for subsequent analysis.
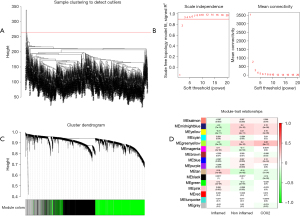
Screen hub genes and explore novel signatures
LASSO regression was used to screen the module genes from the WGCNA analysis to remove the multi-collinearity genes. We set the parameter as lambda.min =0.011, and 116 genes were then filtered from the 924 genes (Figure 4A,4B). Supervised machine learning SVM was applied to classify the relationship between hot and cold tumor classification in these 116 genes, and 10 genes were found to exhibit the minimum cross validation error. (Figure 4C). Each gene was scored by the SVM algorithm, and we selected the top 10 related genes (XIRP1, CLMP, TNFSF4, STMN3, KIRREL1, SH2D3C, MFAP2, C1S19, PLVAP, and VCL) to combine with the three core genes (PDCD1, CD274, and PTGS2) for further analysis. GMM was applied to count the AUC values of 8,191 groups consisting of 13 potential genes. The purple group, which included seven genes (STMN3, KIRREL1, SH2D3C, VCL, PDCD1, CD274, PTGS2), had the highest diagnostic AUC value (Figure 4D). These seven genes consisted of a combined diagnostic signature with an AUC of 0.838, which was considerably higher than that of any single gene alone (Figure 4E), suggesting that this new diagnostic signature could be a predictor of the therapeutic effect of ICIs.
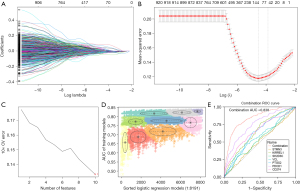
SH2D3C is a potential target and related to the OS of hot tumor
Kaplan-Meier survival analysis shows that the OS of PDCD1 in NSCLC was not significant (log rank P=0.76, two tailed test); the OS of SH2D3C in NSCLC was not significant (log rank P=0.45, two tailed test). In hot tumor, high PDCD1 expression group had worse OS than low PDCD1 expression group (log rank P=0.047, two tailed test); high SH2D3C expression group had worse OS than low SH2D3C expression group either (log rank P=0.003, two tailed test). In cold tumor, the OS of PDCD1 (log rank P=0.49, two tailed test) and SH2D3C (log rank P=0.36, two tailed test) was not significant. It suggested that the OS of PDCD1 and SH2D3C was not associated with that of the NSCLC patients (Figure 5A,5B) or the cold tumor subtype (Figure 5C,5D). Meanwhile, OS was significantly related to the hot tumor subtype (Figure 5E,5F). These findings revealed that PDCD1 and SH2D3C could be survival-relevant biomarkers; however, the remaining genes were not. SH2D3C expression was moderate relative to PDCD1 expression (R=0.49, P<0.001), which suggests that SH2D3C is an important gene may interact with PD-1 to influence TME and ICI treatment in NSCLC (Figure 5G). To understand which drug is suitable for both hot and cold tumors, we searched more than 300 compounds in the GDSC database. By comparing the tumor gene expression profiles and NSCLC cell expression matrix of hot tumor and cold tumors, we found that the AUC of methotrexate (Figure 5H) was lower in hot tumors (P=0.0023) than cold tumor, and that the AUC of trichostatin-A (Figure 5I) was lower in cold tumors (P=0.023). This signifies that methotrexate is effective hot tumors and trichostatin-A could be administered to cold tumor patients.
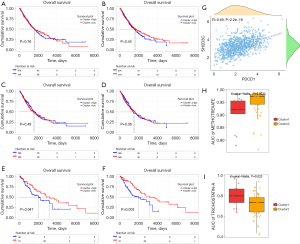
Pathway enrichment related to hot and cold tumor
After WGCNA analysis, we acquired 924 genes to have next step analysis to explore the GO and KEGG pathway enrichment related to the phenotype between hot and cold tumor. The enriched pathways from the DAVID database (Figure 6A,6B) were analyzed using GSVA to further calculate the relationship between these pathways (Figure 6C). We found that the mechanism of focal adhesion and actin cytoskeleton pathways maybe play a crucial role between hot and cold tumor. Survival analysis showed that high enrichment group of the focal adhesion pathway had significantly worse OS than low enrichment group (log rank P=0.005, two tailed test), and high enrichment group of the actin cytoskeleton pathway had worse OS than low enrichment group too (log rank P=0.022, two tailed test) (Figure 6D,6E).
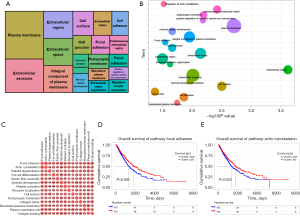
Discussion
ICI therapy has changed the treatment landscape of multiple advanced cancers, including NSCLC (13). In fact, ICI treatment is the first choice of many late stage patients. It is generally acknowledged that NSCLC patients have high PD-1/PD-L1 expression, and thus could have a significant response to PD-1/PD-L1 inhibitor treatment. Nevertheless, a proportion of PD-1/PD-L1-positive patients has little or no response to ICI therapy. Recently, clinicians have tended to use PD-1/PD-L1 DNA expression, tumor mutation burden (TMB), and microsatellite instability/mismatch repair-deficient (MSI/dMMR) to predict the therapeutic effect of ICIs (14). As with the TMB, MSI/dMMR-positive patients still have a certain probability of no response to ICI treatment, with even some patients even experiencing hyper-progressive disease after treatment.
The TME refers to the area in which tumor cells reside, and contains support cells such as blood vessels, CAFs, extracellular matrix, immune-associated cells, and various of signal molecules secreted by different kinds of cell in the TME (15). The TME does not only include substrates; some life activities, such as hypoxia, oxidative stress, metabolic alterations, also occur in the TME (16). All of these processes interact with each other; otherwise, the content change will influence the immune profile of the TME. Considering of TME heterogeneity, even both with high expression of PD-1, the response of ICIs treatment still has huge gap between the patients. There must be some other factors regulating the TME that respond to ICI drugs.
Classifying tumors as either hot or cold is the basic way of distinguishing tumors that could respond to ICI therapy from those that will not. Infiltration of CD8+ T cells into the tumor is most important factor to identify hot and cold tumors. In our study, we found that a cluster identified by unsupervised machine learning, which showed high-level CD8+ T cell infiltration, was correlated to PD-1/PD-L1 expression and was classified as a hot tumor subtype (11). In other clusters, CD8+ T cells and PD-1/PD-L1 were relatively lower than those of the hot tumor cluster, and were thus defined as cold tumors. To understand the difference between the hot and cold tumors, investigated the different genes of these two subtypes, hoping to identify some new biomarkers to predict the response to ICI therapy prior to treatment.
COX-2 is a famous molecule that correlated to inflammation (17). COX-2 inhibitors are common drugs applied in many chronic inflammatory diseases. Chronic inflammation over a prolonged period is a crucial factor in tumorigenesis. COX-2 is also strongly correlated to the TME, and some important cells the TME (such as CAFs, macrophage type 2 cells, and tumor cells) can secrete the COX-2. Thus, dysregulated control of the TME can induce COX-2 overexpression (9,18,19). In our analysis, we found that COX-2 expression was lower in hot tumors than in cold tumors. However, in cold tumors, COX-2 expression in immune-excluded and immune desert subtypes were not different, and the infiltration of CD8+ T cells in these two types was significantly different. This result is consistent with previous research. In the TME, cells can act to both promote and inhibit the inflammatory effect, and the balance of these opposing actions in infiltrating cells is likely the key reason why some patients, who have the same high expression of PD-1/PD-L1, have totally different ICI treatment responses (20). Meanwhile, previous research has indicated that COX-2 inhibitors have the potential value to be anti-tumor assistant drugs and improve the therapeutic outcomes of patients (15). However, these drugs are not strongly recommended for use as a first-line clinical treatment by officials. Considering the availability of these medicines, we believe that COX-2 inhibitor still presents a potential complementary treatment for tumor immunotherapy.
However, the mechanism through which COX-2 inhibitors change the TME and induce anti-tumor effects is still unclear. In our study, we aimed to employ bioinformatics analysis to investigate big data related to NSCLC to screen the crucial genes of immunotherapy response prediction. We defined the hot and cold tumor subtypes of NSCLC data by infiltration of CD8+ T cells, PD-1/PD-L1 expression, and COX-2 gene expression. Using a series of analyses, we established a predictable signature that included seven hub genes to predict whether a tumor was hot or cold before treatment. Our signature had an AUC value of 0.838, which is a delightful discovery. However, due to limitations of the available sources, we did not validate this result. In future research, we plan to collect the tumor tissue or blood samples of patients before administration of ICI therapy explore the predictive value of the signature in the real world.
In this study, we also found an important gene, known as SH2D3C, which had a good correlation with PD-1 expression. Furthermore, NSCLC patient with high expression of SH2D3C and PD-1 may benefit to the OS in hot tumor type. We speculated that SH2D3C may play an important role the PD-1-related tumor immunotherapy. One previous study revealed that SH2D3C plays an oncological role in lung cancer and promotes tumor immune evasion by inhibiting and even excluding T cells in lung tumor environment (21). In further study, it is necessary to use the Clustered Regularly Interspaced Short Palindromic Repeats (CRISPR)-based method to screen the relative genes and downstream pathways. Regulating SH2D3C maybe help to discover novel immune-related pathways and understand the relationship between immunotherapy and the TME. SH2D3C may be a target that could change cold tumors into hot ones, and thus deserves further in-depth research.
Conclusions
In summary, the era of immunotherapy is still in the early days, and a large number of advanced NSCLC patients have already benefited from it. This paper aimed to utilize big data from the TCGA database to analyze the COX-2-related ICI treatment response signatures to predict cold and hot tumors, and improve the clinical treatment strategy. Additionally, SH2D3C is a potential treatment target for NSCLC. Immunotherapy combined with other treatments is being increasingly used as a first-line clinical treatment. Through our study, we hope to identify some novel assistant medicines in immunotherapy, as well as a target that can reverse cold tumors into hot ones.
Acknowledgments
The authors would like to thank TCGA for sharing the LUAD and LUSC datasets.
Funding: This work was supported by the Ningbo Municipal Natural Science Foundation (No. 201401A6110062).
Footnote
Reporting Checklist: The authors have completed the TRIPOD reporting checklist. Available at https://jtd.amegroups.com/article/view/10.21037/jtd-22-257/rc
Conflicts of Interest: All authors have completed the ICMJE uniform disclosure form (available at https://jtd.amegroups.com/article/view/10.21037/jtd-22-257/coif). The authors have no conflicts of interest to declare.
Ethical Statement: The authors are accountable for all aspects of the work in ensuring that questions related to the accuracy or integrity of any part of the work are appropriately investigated and resolved. The study was conducted in accordance with the Declaration of Helsinki (as revised in 2013).
Open Access Statement: This is an Open Access article distributed in accordance with the Creative Commons Attribution-NonCommercial-NoDerivs 4.0 International License (CC BY-NC-ND 4.0), which permits the non-commercial replication and distribution of the article with the strict proviso that no changes or edits are made and the original work is properly cited (including links to both the formal publication through the relevant DOI and the license). See: https://creativecommons.org/licenses/by-nc-nd/4.0/.
References
- Sung H, Ferlay J, Siegel RL, et al. Global Cancer Statistics 2020: GLOBOCAN Estimates of Incidence and Mortality Worldwide for 36 Cancers in 185 Countries. CA Cancer J Clin 2021;71:209-49. [Crossref] [PubMed]
- Travis WD, Brambilla E, Nicholson AG, et al. The 2015 World Health Organization Classification of Lung Tumors: Impact of Genetic, Clinical and Radiologic Advances Since the 2004 Classification. J Thorac Oncol 2015;10:1243-60. [Crossref] [PubMed]
- Duma N, Santana-Davila R, Molina JR. Non-Small Cell Lung Cancer: Epidemiology, Screening, Diagnosis, and Treatment. Mayo Clin Proc 2019;94:1623-40. [Crossref] [PubMed]
- McCune JS. Rapid Advances in Immunotherapy to Treat Cancer. Clin Pharmacol Ther 2018;103:540-4. [Crossref] [PubMed]
- Efremova M, Rieder D, Klepsch V, et al. Targeting immune checkpoints potentiates immunoediting and changes the dynamics of tumor evolution. Nat Commun 2018;9:32. [Crossref] [PubMed]
- Beltra JC, Manne S, Abdel-Hakeem MS, et al. Developmental Relationships of Four Exhausted CD8+ T Cell Subsets Reveals Underlying Transcriptional and Epigenetic Landscape Control Mechanisms. Immunity 2020;52:825-841.e8. [Crossref] [PubMed]
- Du W, Zhu J, Zeng Y, et al. KPNB1-mediated nuclear translocation of PD-L1 promotes non-small cell lung cancer cell proliferation via the Gas6/MerTK signaling pathway. Cell Death Differ 2021;28:1284-300. [Crossref] [PubMed]
- Junttila MR, de Sauvage FJ. Influence of tumour micro-environment heterogeneity on therapeutic response. Nature 2013;501:346-54. [Crossref] [PubMed]
- Hashemi Goradel N, Najafi M, Salehi E, et al. Cyclooxygenase-2 in cancer: A review. J Cell Physiol 2019;234:5683-99. [Crossref] [PubMed]
- Qiu HY, Wang PF, Li Z, et al. Synthesis of dihydropyrazole sulphonamide derivatives that act as anti-cancer agents through COX-2 inhibition. Pharmacol Res 2016;104:86-96. [Crossref] [PubMed]
- Hegde PS, Chen DS. Top 10 Challenges in Cancer Immunotherapy. Immunity 2020;52:17-35. [Crossref] [PubMed]
- Wang SJ, Khullar K, Kim S, et al. Effect of cyclo-oxygenase inhibitor use during checkpoint blockade immunotherapy in patients with metastatic melanoma and non-small cell lung cancer. J Immunother Cancer 2020;8:e000889. [Crossref] [PubMed]
- Bonaventura P, Shekarian T, Alcazer V, et al. Cold Tumors: A Therapeutic Challenge for Immunotherapy. Front Immunol 2019;10:168. [Crossref] [PubMed]
- Lizardo DY, Kuang C, Hao S, et al. Immunotherapy efficacy on mismatch repair-deficient colorectal cancer: From bench to bedside. Biochim Biophys Acta Rev Cancer 2020;1874:188447. [Crossref] [PubMed]
- Hui L, Chen Y. Tumor microenvironment: Sanctuary of the devil. Cancer Lett 2015;368:7-13. [Crossref] [PubMed]
- Augustin RC, Delgoffe GM, Najjar YG. Characteristics of the Tumor Microenvironment That Influence Immune Cell Functions: Hypoxia, Oxidative Stress, Metabolic Alterations. Cancers (Basel) 2020;12:3802. [Crossref] [PubMed]
- Yao C, Narumiya S. Prostaglandin-cytokine crosstalk in chronic inflammation. Br J Pharmacol 2019;176:337-54. [Crossref] [PubMed]
- Ohtsuka J, Oshima H, Ezawa I, et al. Functional loss of p53 cooperates with the in vivo microenvironment to promote malignant progression of gastric cancers. Sci Rep 2018;8:2291. [Crossref] [PubMed]
- Liu Y, Borchert GL, Surazynski A, et al. Proline oxidase, a p53-induced gene, targets COX-2/PGE2 signaling to induce apoptosis and inhibit tumor growth in colorectal cancers. Oncogene 2008;27:6729-37. [Crossref] [PubMed]
- Pelly VS, Moeini A, Roelofsen LM, et al. Anti-Inflammatory Drugs Remodel the Tumor Immune Environment to Enhance Immune Checkpoint Blockade Efficacy. Cancer Discov 2021;11:2602-19. [Crossref] [PubMed]
- Yeh YC, Lawal B, Hsiao M, et al. Identification of NSP3 (SH2D3C) as a Prognostic Biomarker of Tumor Progression and Immune Evasion for Lung Cancer and Evaluation of Organosulfur Compounds from Allium sativum L. as Therapeutic Candidates. Biomedicines 2021;9:1582. [Crossref] [PubMed]