Research output of artificial intelligence in arrhythmia from 2004 to 2021: a bibliometric analysis
Introduction
Artificial intelligence (AI) refers to computer algorithms with human-like intelligence developed to solve specific problems (1). The methodologies of AI are changing with the improvement of technology, and machine learning (ML) may sometimes be referred to as AI in cardiological guidelines, as ML is the major technique of AI currently applied in this field (2,3). As a branch of ML, artificial neural network (ANN) is becoming increasingly promising in medicine, despite twice experiencing the “AI winter” (4). ANN, including deep learning and convolutional neural network, are comprised of an input layer, hidden layers, and an output layer of neurons which respectively process information that is fed in or put out of the model (5). In fact, this is not a new concept; it has been utilized in clinical decision-making in medicine decades ago in the 1990s, which could be regarded as the onset of its application (6).
By promoting data classification and comprehensive decision-making, AI is gradually being used in many applications in clinical practice (7). In particular, cardiovascular medicine, which processes diversified format of nonlinear and visual clinical data, such as electrocardiograms (ECG) and echocardiograms, requires a different and superior approach to traditional linear analysis. Moreover, hemodynamic and electrophysiological data are being increasingly captured by the popularity of wearable devices. The development of numerous AI software programs has prompted the use of deep learning in cardiac arrhythmia classification and advanced image processing by deconvoluting the segmentation of cardiac indices (8). Thus, a lot of recent study focus on the big data processing of these separate information in cardiovascular data and imaging due to the digitization of daily clinical activities which can hardly be possible in the past (9). As the most common technique for detecting cardiac arrhythmia, ECG provides a massive amount of data collected in home and hospital settings. Various approaches have been proposed and well researched in recent years, including methods of achieving a higher level of automatic arrhythmia event selection as well as noise reduction, to support more accurate computerized interpretation (10). However, there has been limited systematic analysis of deep learning in arrhythmia.
Having been performed for nearly one hundred years, quantitative studies, especially bibliometric methods, are increasingly being developed and used to estimate the variations of productivity of countries, authors, institutions and journals (11,12). By undertaking a statistical and quantitative analysis of publications and identifying and extracting ANN studies in arrhythmia, we analyzed clusters of citations and keywords in these studies. Furthermore, we used multiple analytical tools to map the trends of arrhythmia research in deep learning from 2004 to 2021. In this study, AI mainly referred to ANN which is presently the mainstream ML method designed to mimic human neuron working pattern by setting a simple mathematical equation with parameters in each neural network adjusted during network training (3,13). However, support vector machine (SVM) learning is closely related to ANN learning (SVM can be regarded as two layers of neural networks, and in this study we considered both SVM and ANN as similar methods of ML) (13); hence, we also included articles relevant to SVM and ANN. This analysis will provide an overview of the current understandings in the literature regarding ANN in arrhythmia and directions for future study.
Methods
Search strategy
Data were acquired on July 12, 2021, from the Science Citation Index-Expanded (SCI-E) edition of the Web of Science Core Collection (WoSCC) v. 5.32 (Clarivate Analytics, Philadelphia, USA; https://clarivate.com/). There were no ethical issues, as the data were downloaded from the public-facing WoSCC. Literature published from 2004 to 2021 were included in the search, and the following keywords and terms were used: ‘Artificial intelligence’ or ‘Neural Network Model’ or ‘Deep learning’ and ‘Arrhythmia’. To avoid changes in citation rates, all searches were performed on the same day (July 12, 2021). Given that database entries for the year would not be complete at the time of the search, the year 2022 was excluded. After all data were collected, the results were arranged according to the number of times cited.
Study selection
By reading the title and abstracts, two independent reviewers collected all the data acquired from the SCI-E edition of the WoSCC database. A full text was downloaded from PubMed or other databases, accordingly, only if the main topic was concerned with AI and arrhythmia and the language of publication was English. The exclusion criteria were as follows: (I) the main topic of the article was not concerned with AI and arrhythmia; (II) the abstract of the article could not be acquired from the WoSCC; or (III) the article was a duplicate. A third reviewer settled any differences between the two reviewers through discussion.
Assessment of the articles and journals
The following data were identified and recorded for analysis by the two reviewers: (I) titles; (II) authors; (III) numbers of citations; (IV) keywords; (V) publication years; (VI) topics; (VII) funding source; (VIII) countries of origin; (IX) journal names; and (X) impact factors (IFs) using the Journal Citation Reports (JCR, 2021 edition).
Data synthesis and analysis
After converting to txt text file format, the data were imported into the online analytic platforms, Literature Metrology (http://bibliometric.com/) and VOSviewer v. 1.6.15 (Leiden University’s Centre for Science and Technology Studies, Leiden, Netherlands; https://www.cwts.nl) including collaborative networks, keywords, institutions, cited references, authorship and so on. Keywords used more than twice were selected and assembled for analysis focusing on the types of ECG signal as well as neural networks. GraphPad Prism version 9.1.1 (GraphPad Software Inc, California, USA) was used in the data synthesis and illustration of ECG signal. The Literature Metrology platform was used to analyze the number of included articles and the number of articles published each year. The top 17 keywords for each year were identified. VOSviewer software was used to present the keyword occurrence network, with two-dimensional knowledge maps of the relative positions and density of nodes (i.e., keywords) in the network.
Results
Total numbers of published articles
Taking the number of published items into consideration, from 2004 to 2021, a total of 713 publications were identified in the WoSCC using our search strategy. Subsequently, after screening titles and abstracts, 636 papers were selected for analysis, while 77 publications were excluded because of their irrelevance to the inclusion criteria (unrelated titles, 73 papers; inaccessibility of study, 2 papers; duplications of other studies, 2 papers). In total, 636 articles on the topic of deep learning of arrhythmia from 2004 to 2021 were included in the analysis (Figure 1). The number of publications began to increase dramatically from 2017 onwards, and the annual number of publications was over 140 in 2020 (Figure 2).
Distribution by countries
The 636 included articles were mostly published in five countries/regions (n=503, 79.09%) (Figure 3). The greatest number of publications came from China (n=181, 28.46%), followed by the United States (US; n=139, 21.86%), India (n=87, 13.68%), and England (n=53, 8.33%). Of all the countries, China exhibited the most dramatic growth in the number of publications over the last 5 years. The frequency of international collaboration is shown in Figure 4; the US and China collaborated most frequently, followed by China and Australia.
Distribution by citations
Of the 636 selected articles, the top 100 articles were ranked according to the number of times they had been cited by other sources (Table 1). During the period from 2004 to 2021, the top-cited article (number of citations, n=703) was “Automatic classification of heartbeats using ECG morphology and heartbeat interval features”, which was published in 2004 and achieved a Web of Science citation index (WoSCI) of 43.05. This was followed by the article, “Real-time patient-specific ECG classification by 1-D convolutional neural networks” (n=565), which was published in 2016 and achieved a WoSCC of 96.57. The third-ranked article, “Cardiologist-level arrhythmia detection and classification in ambulatory electrocardiograms using a deep neural network” (n=402) was published in 2019 and achieved a WoSCC of 134. The fourth-ranked article, “A deep convolutional neural network model to classify heartbeats” (n=291) was published in 2017 and achieved a WoSCC of 58.2. The fifth-ranked the article, “Classification of electrocardiogram signals with support vector machines and particle swarm optimization” (n=289) was published in 2008 and achieved a WoSCC of 20.64.
Table 1
Rank | Journal | The total number of articles |
The total number of references |
Average number of citations |
---|---|---|---|---|
1 | Information Sciences | 5 | 214 | 42.8 |
2 | Nature Medicine | 2 | 85 | 42.5 |
3 | IEEE Transactions on Systems Man Cybernetics-Systems | 1 | 27 | 27 |
4 | Information Fusion | 1 | 21 | 21 |
5 | Entropy | 2 | 37 | 18.5 |
Distribution by journals
The 636 included articles were published by 270 journals. The Institute of Electrical and Electronics Engineers (IEEE) Access [2021; impact factor (IF) =3.367] contributed the largest number of articles (36 articles, 5.66%), followed by Computers in Biology and Medicine (2021; IF =4.589; 21 articles, 3.30%), Physiological Measurement (2021; IF =2.833; 13 articles, 2.04%), Biological Signal Processing and Control (2021; IF =3.88; 10 articles, 1.57%), and Expert Systems with Applications (2021; IF =6.954; 9 articles, 1.41%), according to the Journal Citation Reports (JCR) 2021 standards (Table 2). The top five journals of the top 100 articles ranked by the number of citations contributed to publications were Information Sciences (2021; 42.8 average citations), followed by Nature Medicine (2021; 42.5 average citations), IEEE Transactions on Systems Man Cybernetics-Systems (2021; 27 average citations), Information Fusion (2021; 21 average citations), and Entropy (2021; 18.5 average citations), according to the Journal Citation Reports (JCR) 2021 standards (Table 3).
Table 2
Rank | Journal | The total number of articles |
The total number of references |
Average number of citations |
---|---|---|---|---|
1 | IEEE Access | 36 | 75 | 2.08 |
2 | Computers In Biology and Medicine | 21 | 375 | 17.86 |
3 | Physiological Measurement | 13 | 72 | 5.54 |
4 | Biomedical Signal Processing and Control | 10 | 120 | 12 |
5 | Expert Systems with Applications | 9 | 77 | 8.56 |
Table 3
Rank | Journal | The total number of articles |
The total number of references |
Average number of citations |
---|---|---|---|---|
1 | Information Sciences | 5 | 214 | 42.8 |
2 | Nature Medicine | 2 | 85 | 42.5 |
3 | IEEE Transactions on Systems Man Cybernetics-Systems | 1 | 27 | 27 |
4 | Information Fusion | 1 | 21 | 21 |
5 | Entropy | 2 | 37 | 18.5 |
Analysis of keywords
An estimation of trends in the field was revealed by analyzing the top 17 keywords (Figure 5). Nearly all of the keywords showed an ascending tendency. The keywords, ‘Classification’ and ‘Model’, were the first and second most frequently used keywords, respectively, in the last 5 years of the study period.
Distribution by authors
There were more than 2,530 authors who contributed to the publications. The network maps of the authors and co-cited authors produced by the VOSviewer software are shown in Figure 6A,6B. The author, Acharya, UR, provided the greatest number of articles (n=21), followed by Oh, SL (n=6) and Tan, RS (n=6). The top five cited authors were Acharya, UR (n=676), Tan, RS (n=316), Oh, SL (n=214), and Tan, JH (n=207) (Tables 4,5).
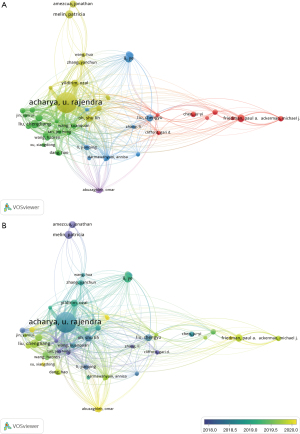
Table 4
Rank | Authors | The total number of articles | The total number of references | Average number of citations |
---|---|---|---|---|
1 | Acharya, UR | 21 | 676 | 32.19 |
2 | Oh, SL | 6 | 214 | 35.67 |
3 | Tan, RS | 6 | 316 | 52.67 |
4 | Liu, CY | 6 | 13 | 2.17 |
5 | Zhang, L | 6 | 10 | 1.67 |
Table 5
Rank | Authors | The total number of articles | The total number of references | Average number of citations |
---|---|---|---|---|
1 | Acharya, UR | 15 | 676 | 32.19 |
2 | Tan, RS | 6 | 316 | 52.67 |
3 | Oh, SL | 6 | 214 | 35.67 |
4 | Tan, JH | 3 | 207 | 69 |
5 | Adam, M | 3 | 207 | 69 |
Distribution by institutions
The publications were from 1,042 institutions. An extensive collaborative network analysis was demonstrated in Figure 7A,7B, in which the distance between two keywords indicates the connection of the keywords in terms of co-citation links. The top five institutions published 96 articles (about 15.09% of the total). The Mayo Clinic (n=29, 4.56%) published the greatest number of articles, followed by Ngee Ann Polytechnic (n=22, 3.46%), the Chinese Academy of Sciences (n=18, 2.83%), Shanghai Jiao Tong University (n=16, 2.51%), and the National Cheng Kung University (n=11, 1.72%) (Table 6). The top five institutions ranked by the number of citations were National Heart Center of Singapore (2021; 37.13 average citations), followed by Ngee Ann Polytechnic (2021; 33.77 average citations), University of Malaya (2021; 31.70 average citations), National University of Singapore (2021; 28 average citations), and the Stanford University (2021; 25.2 average citations) respectively (Table 7).
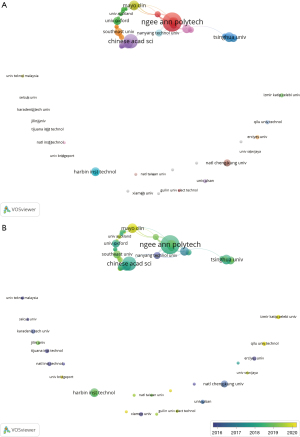
Table 6
Rank | The total number of articles |
The total number of references |
Average number of citations |
|
---|---|---|---|---|
1 | Mayo Clinic, Florida, US | 29 | 19 | 0.66 |
2 | Ngee Ann Polytechnic, Singapore | 22 | 743 | 33.77 |
3 | Chinese academy of sciences, Beijing, China | 18 | 83 | 4.61 |
4 | Shanghai Jiao Tong University, Shanghai, China | 16 | 16 | 1 |
5 | National Cheng Kung University, Taiwan, China | 11 | 104 | 9.45 |
Table 7
Rank | The total number of articles |
The total number of references |
Average number of citations |
|
---|---|---|---|---|
1 | National Heart Center of Singapore, Singapore | 8 | 297 | 37.13 |
2 | Ngee Ann Polytechnic, Singapore | 22 | 743 | 33.77 |
3 | University of Malaya, Kuala Lumpur, Malaysia | 10 | 317 | 31.7 |
4 | National University of Singapore, Singapore | 7 | 196 | 28 |
5 | Stanford University, California, US | 10 | 252 | 25.2 |
Knowledge map of deep learning research in arrhythmia
The network visualization of keywords is shown in Figure 8A,8B. The connection between two keywords was stronger when they were located closer to each other. Furthermore, lines represented the strongest co-citation links between keywords. As demonstrated in Figure 8C, a two-dimensional knowledge map with major keywords as nodes showed the frequency of keywords as well as their relative co-occurrence using colors of different intensities. An intense (yellow) color indicated the frequency of keywords, which are labeled in the center of each intense color using different sizes. The knowledge map indicated those keywords in the publications that occurred more frequently and co-occurred with a higher number of other keywords.
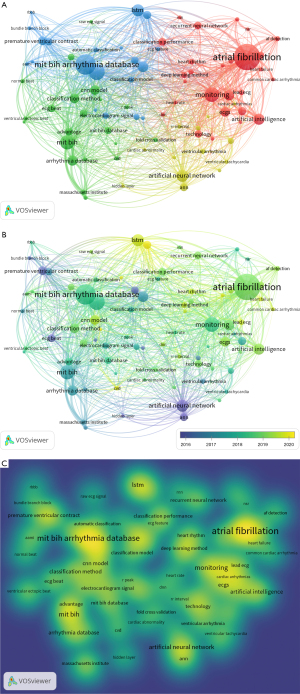
Type of ECG signal
Figure 9 demonstrates the type of ECG signal, which was the focus of the published studies, according to the frequency of keywords. Of the seven types of arrhythmias identified, atrial fibrillation (AF) (36%) and ventricular arrhythmia (32%) were the most frequently studied. These were followed by detection of sinus rhythm (11%), bundle branch block (8%), myocardial infarction (5%), supraventricular arrhythmia (4%), and sudden cardiac death (4%).
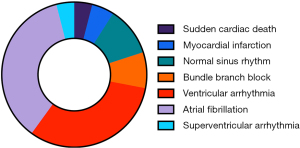
Main ANN algorithms in electrocardiogram interpretation
The main types of algorithms utilized in electrocardiogram are shown in Table 8, all of which are algorithms based on the supervised learning model. The algorithms most frequently applied to the interpretation of arrhythmia were the convolutional neural network (51 times) and SVM (63 times) algorithms. Neural model algorithms, such as the recurrent neural model (19 times), the probabilistic neural model (13 times), and the neuro-fuzzy system (17 times), were also widely used in the interpretation of arrhythmia. Generally, feed forward neural networks (eight times), convolutional recurrent neural network (RNN) (three times), and radical basis function neural networks (four times) were applied fewer times.
Table 8
Algorithms | Type of learning | Frequency |
---|---|---|
Convolutional neural network | Supervised | 51 |
Convolutional recurrent neural network | Supervised | 3 |
Feed forward neural network | Supervised | 8 |
Neuro-fuzzy System | Supervised | 17 |
Recurrent neural network | Supervised | 19 |
Radical basis function neural network | Supervised | 4 |
Probabilistic neural network | Supervised | 13 |
Support vector machine | Supervised | 63 |
ANN, artificial neural network.
Discussion
AI has been developing at an accelerating rate in recent years. Roughly divided into supervised learning and non-supervised learning by whether data is labeled, ML is the frontier of AI nowadays (14). The increasing application of ML in medicine has generated considerable interest among researchers. Most researches focused on application of AI in tracking of health behavior and medical diagnosis as well as prognostication (15). As the first bibliometric analysis of AI in arrhythmia, we found the number of publications drastically increased annually from several articles in 2004, to a booming over the last 5 years as the rapid development of computer science, popularity of the concept of artificial intelligence (which attracts investment and more academic as well as social interaction) and partially due to the growing number of output index in the number of publications in the WoSCC database. In the evolution of networks, convolutional neural network (CNN) architecture, such as ResNet, became distinguished in 2015 for better estimating the target neural function with increased nonlinearity by increasing the depth of neurons (16). Since then, the application of ANN soared up with a larger and larger number of relevant publications and we can find papers associated with ANN in arrhythmia began to increase in 2017. As demonstrated in Figure 8B, the methodology of AI over the 5 years mainly refers to neural networks instead of SVM. We may expect the development of ANN especially deep learning in arrhythmia promising with numerous future potentials.
China has contributed the most studies to the field over the years, being among the top 10 countries/regions and accounting for 25.14% of the total number of publications. However, the citation index remains low, suggesting that while there is increasing focus on the development of deep learning in arrhythmia in China, there needs to be more improvement in the range and depth of research on the topic. Despite only contributing 4.21% of the publications, Singapore dominated the top five list of institutions publishing the most articles. Ngee Ann Polytechnic, the National Heart Centre of Singapore, and the National University of Singapore all ranked among the top five, with an average number of citations over 30. The United States, which produced 19.52% of the publications, demonstrated a significant influence in neuro networks in arrhythmia research. With respect to international collaboration, both China and the US have played key roles in promoting international cooperation, with the strongest international collaborations existing between the United States and China, followed by Turkey and Singapore (Figure 4). As for institutional collaboration (as ranked by the number of published articles), the Mayo Clinic, Ngee Ann Polytechnic, and the Chinese Academy of Sciences were among the top five institutions (in that order), with one of these institutions affiliated with the United States, while the other two are separately affiliated with Singapore and China.
Among the top five journals (as ranked by the average citations index), four were in the engineering realm, which was consistent with the list of top journals (as ranked by the number of published articles). The average citation per article was 12.6, while the total number of references in the publications was 9,009. The IFs of the published journals varied which indicated that this topic has only recently become a focus of interest. Moreover, the journals were comprised of a wide range of categories, from engineering to computer science and medicine, suggesting collaboration between different subjects and disciplines.
Ranked by the number of articles published, the top author identified was affiliated with Ngee Ann Polytechnic and had published 21 articles; the four other authors in the list had each published six articles. Interestingly, Acharya, UR, the author with the most publications, also included using the greatest number of references, suggesting potentially better quality of his publications. Consequently, we identified many co-citations related to his work, including Oh, SL, and Tan, RS, whose average number of citations ranked in the top two, (as shown in Figure 8). Furthermore, since 2018, there have been an increasing number of authors focusing on this research area.
All 49 items were divided into four clusters with four different colors. Clusters 1, 2, 3, and 4 were comprised of 10,17, 13, and 9 items, respectively, in the network visualization, ranging from blue to yellow. Each had a differently-sized label, as determined by the weight of the item as well as the cluster to which they belonged (Figure 8A,8B). Lines between items and the distance between two keywords represented the links and relatedness of the keywords in terms of co-citation links in the visualization, and was similarly in the item density visualization. The findings indicated that AI was initially applied to relatively simple situations, such as the detection of heart beats (17), and was later utilized to further analyze common cardiac arrhythmias with the application of special neural networks, including the CNN and RNNs concomitantly. AF constituted a large part of the map (as shown in Figure 9), indicating that AF has become a controversial focus in this topic. Moreover, the keywords “MIT BIH arrhythmia database” make up a significant part of the visualization as well, suggesting that many scholars applied AI using this dataset and this dataset could be the largest online public arrhythmia dataset.
AI in medicine has been booming since 2016, with most of the growth attributed to three major players: the US, Europe, and China (18). Compared with the US and Europe, China was a late participant to this competition. Yet, China has processed a large number of clinical datasets for the training of AI models, which are indispensable for developing a precise model; other countries cannot claim merit for a similar achievement. In cardiology, electrocardiogram can be difficult for beginners to master. Advancement in data space augmentation as well as synthesis using deep learning models are making the application of AI in detection and treatment of arrhythmia feasible (19). AI was originally created as a pattern with different layers of precision, including input, pattern, summation, normalization, and output, which were regarded as the neural network (20). In fact, linear regression is a one-layer neuron network expressed as Y=a+b1X1+b2X2,while a SVM could be regarded as two layers networks. Although SVM demonstrated good capability in non-linear classification and was the major player in the realm of AI in arrhythmia, the term SVM rarely showed up after 2016 according to Figure 8B (21). Being more accurate algorithmically, ANN could have more capacity in model fitting, in terms of non-linear prediction, and has thus become the mainstream AI methodology in arrhythmia (21,22). Basically, with an appropriate number of hidden nodes in a layer, the neural network model will have a low estimation error, as determined by the amount of data and the avoidance of over fitting (23). CNN has become the culprit neural network model in the analysis of medical images (24). It is widely used in the recognition, classification, and processing of images and appeared frequently as a result, as illustrated in Table 8 (25). However, most of the present approach of utilizing AI involves labeling data initially and then following this up with an analysis of the labeled data. It requires intensive labor in labeling clinical message. The encouragement of semi-supervised learning as well as self-supervised learning could prompt the utilization of AI in arrhythmia more widely, as a self-supervised learning method only requires the operator to input a small number of typical data in order for computers to be able to automatically extract unlabeled data without supervision (26,27). Its application eliminates the need for manual recognition and, in the foreseeable future, could probably become mature enough to be utilized in in-hospital monitoring, radiofrequency ablation operations, and portable devices (28). For patients with angina, this monitoring could not only monitor the onset of myocardial infraction (MI), but could also help in predicting it. For example, the de Winter syndrome is regarded as a sign of possible progression of MI. The diagnosis of arrhythmia could be faster and more accurate, especially for an arrhythmia such as AF, which is asymptomatic initially but requires early detection and monitoring to control heart rate. Significantly, AF accounts for 36% of the present studies in this field (Figure 9). Nevertheless, most studies today focus on ECG differentiation (29-31). However, recently OPTIMA approach (termed as optimal target identification via modelling of arrhythmogenesis) was proposed by Boyle et al. (32), which involves the identification of optimal atria target in persistent atrial fibrillation patients via modelling of arrhythmogenesis. The model was reconstructed by means of late gadolinium enhancement magnetic resonance imaging (LGE-MRI) scans, which detect fibrotic details of atria based on each individual (32,33). This is the hallmark of the utilization of AI from “two dimension” electrocardiogram to “three dimension” model of atria arrhythmia detection and suggests the trend in the future.
Limitations
Articles included in our study were restricted to peer-reviewed and English-language publications; journals and articles published without peer-review or in a language other than English were excluded in the citation analysis. As the number of citations of an article increases over time, those articles published in the earlier part of the period selected for the literature search would have achieved relatively more citations than later studies, potentially affects the citation analysis. We did not take self-citation into consideration.
Conclusions
By identifying and analyzing articles focusing on the application of AI in arrhythmia, we demonstrated the rapid of AI in arrhythmia research. AI is becoming prevalent in the diagnosis, prognostication, and treatment of arrhythmia, especially in atrial fibrillation and ventricular arrhythmia. Notably, between 2004 and 2021, Singapore played an indispensable role in this field, with a significant number of collaborations and citations. China, Europe, India, and the US accounted for most of the publications on the application of AI in arrhythmia during this period.
Acknowledgments
We would like to thank K. Gilbert and A. Kassem for their help in polishing our paper.
Funding: This work was supported by the National Natural Science Foundation of China (Nos. 81900301 and 81870254), National Key Research and Development Program of China (No. 2018YFC1313502), Science and Technology Planning Project of Guangzhou (No. 201904010451), Guangdong Medical Science and Technology Research Funding (No. A2017516), Sichuan Science and Technology Program (No. 2021YFS0159) and Special Project for Research and Development in Key Areas of Guangzhou Province (No. 2019B020230004).
Footnote
Peer Review File: Available at https://jtd.amegroups.com/article/view/10.21037/jtd-21-1767/prf
Conflicts of Interest: All authors have completed the ICMJE uniform disclosure form (available at https://jtd.amegroups.com/article/view/10.21037/jtd-21-1767/coif). SH reports receiving funding from Guangdong Medical Science and Technology Research Funding (No. A2017516). GK reports receiving funding from Sichuan Science and Technology Program (No. 2021YFS0159). WW reports receiving funding from National Natural Science Foundation of China (No. 81900301) and Science and Technology Planning Project of Guangzhou (No. 201904010451). YX reports receiving funding from National Natural Science Foundation of China (No. 81870254), Special Project for Research and Development in Key Areas of Guangzhou Province (No. 2019B020230004), and National Key Research and Development Program of China (No. 2018YFC1313502). SW reports receiving funding from Special Project for Research and Development in Key Areas of Guangzhou Province (No. 2019B020230004) and National Key Research and Development Program of China (No. 2018YFC1313502). The other authors have no conflicts of interest to declare.
Ethical Statement: The authors are accountable for all aspects of the work in ensuring that questions related to the accuracy or integrity of any part of the work are appropriately investigated and resolved.
Open Access Statement: This is an Open Access article distributed in accordance with the Creative Commons Attribution-NonCommercial-NoDerivs 4.0 International License (CC BY-NC-ND 4.0), which permits the non-commercial replication and distribution of the article with the strict proviso that no changes or edits are made and the original work is properly cited (including links to both the formal publication through the relevant DOI and the license). See: https://creativecommons.org/licenses/by-nc-nd/4.0/.
References
- Abdulkareem M, Petersen SE. The Promise of AI in Detection, Diagnosis, and Epidemiology for Combating COVID-19: Beyond the Hype. Front Artif Intell 2021;4:652669. [Crossref] [PubMed]
- Nagarajan VD, Lee SL, Robertus JL, et al. Artificial intelligence in the diagnosis and management of arrhythmias. Eur Heart J 2021;42:3904-16. [Crossref] [PubMed]
- Attia ZI, Harmon DM, Behr ER, et al. Application of artificial intelligence to the electrocardiogram. Eur Heart J 2021;42:4717-30. [Crossref] [PubMed]
- Muthukrishnan N, Maleki F, Ovens K, et al. Brief History of Artificial Intelligence. Neuroimaging Clin N Am 2020;30:393-9. [Crossref] [PubMed]
- Renganathan V. Overview of artificial neural network models in the biomedical domain. Bratisl Lek Listy 2019;120:536-40. [Crossref] [PubMed]
- Itchhaporia D, Snow PB, Almassy RJ, et al. Artificial neural networks: current status in cardiovascular medicine. J Am Coll Cardiol 1996;28:515-21. [Crossref] [PubMed]
- Krittanawong C, Johnson KW, Rosenson RS, et al. Deep learning for cardiovascular medicine: a practical primer. Eur Heart J 2019;40:2058-73. [Crossref] [PubMed]
- Miller DD. Machine Intelligence in Cardiovascular Medicine. Cardiol Rev 2020;28:53-64. [Crossref] [PubMed]
- Wang QC, Wang ZY. Big Data and Atrial Fibrillation: Current Understanding and New Opportunities. J Cardiovasc Transl Res 2020;13:944-52. [Crossref] [PubMed]
- Parvaneh S, Rubin J, Babaeizadeh S, et al. Cardiac arrhythmia detection using deep learning: A review. J Electrocardiol 2019;57S:S70-4. [Crossref] [PubMed]
- Rousseau R. Library science: Forgotten founder of bibliometrics. Nature 2014;510:218. [Crossref] [PubMed]
- Agarwal A, Durairajanayagam D, Tatagari S, et al. Bibliometrics: tracking research impact by selecting the appropriate metrics. Asian J Androl 2016;18:296-309. [Crossref] [PubMed]
- Krogh A. What are artificial neural networks? Nat Biotechnol 2008;26:195-7. [Crossref] [PubMed]
- Badillo S, Banfai B, Birzele F, et al. An Introduction to Machine Learning. Clin Pharmacol Ther 2020;107:871-85. [Crossref] [PubMed]
- Tran BX, Latkin CA, Vu GT, et al. The Current Research Landscape of the Application of Artificial Intelligence in Managing Cerebrovascular and Heart Diseases: A Bibliometric and Content Analysis. Int J Environ Res Public Health 2019;16:2699. [Crossref] [PubMed]
- Gu JX, Wang ZH, Kuen J, et al. Recent advances in convolutional neural networks. Pattern Recognit 2018;77:354-77. [Crossref]
- Acharya UR, Oh SL, Hagiwara Y, et al. A deep convolutional neural network model to classify heartbeats. Comput Biol Med 2017;89:389-96. [Crossref] [PubMed]
- Tran BX, Vu GT, Ha GH, et al. Global Evolution of Research in Artificial Intelligence in Health and Medicine: A Bibliometric Study. J Clin Med 2019;8:360. [Crossref] [PubMed]
- Wang S, Zhang L, Zuo W, et al. Class-specific Reconstruction Transfer Learning for Visual Recognition Across Domains. IEEE Trans Image Process 2019; [Epub ahead of print]. [PubMed]
- Ozyildirim BM, Avci M. Generalized classifier neural network. Neural Netw 2013;39:18-26. [Crossref] [PubMed]
- Johnson KW, Torres Soto J, Glicksberg BS, et al. Artificial Intelligence in Cardiology. J Am Coll Cardiol 2018;71:2668-79. [Crossref] [PubMed]
- Wei W, Yang X. Comparison of Diagnosis Accuracy between a Backpropagation Artificial Neural Network Model and Linear Regression in Digestive Disease Patients: an Empirical Research. Comput Math Methods Med 2021;2021:6662779. [Crossref] [PubMed]
- Xu LY, Bai Y, Hu M, et al. Artificial neural network in the prediction of nosocomial infection risk. Zhong Nan Da Xue Xue Bao Yi Xue Ban 2006;31:404-7. [PubMed]
- Anwar SM, Majid M, Qayyum A, et al. Medical Image Analysis using Convolutional Neural Networks: A Review. J Med Syst 2018;42:226. [Crossref] [PubMed]
- Litjens G, Ciompi F, Wolterink JM, et al. State-of-the-Art Deep Learning in Cardiovascular Image Analysis. JACC Cardiovasc Imaging 2019;12:1549-65. [Crossref] [PubMed]
- Dalca AV, Balakrishnan G, Guttag J, et al. Unsupervised learning of probabilistic diffeomorphic registration for images and surfaces. Med Image Anal 2019;57:226-36. [Crossref] [PubMed]
- Pinto A, Pereira S, Meier R, et al. Combining unsupervised and supervised learning for predicting the final stroke lesion. Med Image Anal 2021;69:101888. [Crossref] [PubMed]
- Ponomariov V, Chirila L, Apipie FM, et al. Artificial Intelligence versus Doctors' Intelligence: A Glance on Machine Learning Benefaction in Electrocardiography. Discoveries (Craiova) 2017;5:e76. [Crossref] [PubMed]
- Machiraju PK, Yedla P, Gubbala SP, et al. Identification, synthesis and evaluation of CSF1R inhibitors using fragment based drug design. Comput Biol Chem 2019;80:374-83. [Crossref] [PubMed]
- Attia ZI, Kapa S, Lopez-Jimenez F, et al. Screening for cardiac contractile dysfunction using an artificial intelligence-enabled electrocardiogram. Nat Med 2019;25:70-4. [Crossref] [PubMed]
- Attia ZI, Noseworthy PA, Lopez-Jimenez F, et al. An artificial intelligence-enabled ECG algorithm for the identification of patients with atrial fibrillation during sinus rhythm: a retrospective analysis of outcome prediction. Lancet 2019;394:861-7. [Crossref] [PubMed]
- Boyle PM, Zghaib T, Zahid S, et al. Computationally guided personalized targeted ablation of persistent atrial fibrillation. Nat Biomed Eng 2019;3:870-9. [Crossref] [PubMed]
- Zahid S, Cochet H, Boyle PM, et al. Patient-derived models link re-entrant driver localization in atrial fibrillation to fibrosis spatial pattern. Cardiovasc Res 2016;110:443-54. [Crossref] [PubMed]