Development and validation of a prognostic risk signature for lung adenocarcinoma constructed by six ferroptosis, necroptosis, and pyroptosis-related lncRNAs
Introduction
Lung cancer is a common and serious cancer globally, with an incidence rate of 11.4% and mortality rate of 18.0% (1). About 85% of all lung cancer cases are non-small cell lung cancer (NSCLC) and 15% are small cell lung cancer (SCLC) (2), and lung adenocarcinoma (LUAD) is a major histological type of NSCLC, accounting for more than 50% of NSCLC cases (3). The opportunity to provide appropriate treatment is often delayed as many cases are diagnosed late, and due to the complex tumor microenvironment (TME) and heterogeneity, the sensitivity and specificity of chemotherapy and targeted therapy remain low. In recent years, with the increasing understanding of programmed cell death (PCD) mechanisms in tumor immunity, immunotherapy represented by immune checkpoint inhibitors (ICIs) has brought promising new approaches to LUAD treatment (4,5). However, the number of patients benefiting from immunotherapy remains in the minority, and there remain few biomarkers that can effectively identify immunotherapy beneficiaries (6). Therefore, it is urgent to explore prognostic markers that can help evaluate the individualized treatment of LUAD.
Ferroptosis, necroptosis, and pyroptosis are three PCD mechanisms different from apoptosis and autophagy. Ferroptosis is a process in which reactive oxygen species (ROS) induced by ferric divalent oxygenase or ester oxygenase catalyze the accumulation of highly expressed unsaturated fatty acids on cell membranes and peroxidation and induce cell death, which is exacerbated by the reduced expression of antioxidant systems (glutathione GSH and GPX4) (7,8). Necroptosis is a form of PCD unrelated to caspase (9). Due to the inhibition of caspase-8 activity, RIP1 and RIP3 could be ineffectively cleaved and inactivated or moved to the apoptotic pathway (10). Trans-phosphorylation of RIP1 and RIP3 also promotes their aggregation into filamentous-like necrosome (11). MLKL is further activated by RIP3 and is recruited to the cell membrane and changes its permeability, leading to cell death (12). Pyroptosis is another form of PCD, which induces shear and aggregation of gasdermin family members through caspase 1, 4, 5, 11 and lipopolysaccharide (LPS)-mediated inflammasome, regulating cell proliferation and cell death (13-15).
Exploring the interaction between PCD and the TME is a new strategy to explore cancer treatment, and many studies have confirmed the effect of ferroptosis, necrosis, and pyroptosis on tumor immunity (16-18). In addition, these PCDs may trigger strong anti-tumor immunity in tumors, and the combination of mutagens and ICIs can play a synergistic role in enhancing anti-tumor activity (19-21). Interestingly, in the TME, not only are there interactions between PCD and tumor immunity, but there are also potential interactions among ferroptosis, necroptosis, and pyroptosis (22). However, only a few studies have explored the co-actors and processes in these PCDs (23-25).
Long non-coding RNA (lncRNA) are more than 200 nucleotides in length and are the most abundant type of non-protein-coding RNA (26). They are widely distributed and regulate various physiological and pathological processes, including PCD. Tran et al. reported the regulatory effects of some lncRNAs on ferroptosis, necroptosis, and pyroptosis in cancer (27-29). In addition, most of the existing prognostic models related to PCD only consider a single mechanism, such as ferroptosis, necroptosis, and pyroptosis, while the factors of the combined effects of multiple mechanisms are not fully reflected. Ferroptosis, necroptosis, and pyroptosis-related lncRNA (FNPRlncRNA) affects PCD through multiple mechanisms and is closely related to tumor development and prognosis. The study of FNPRlncRNA may be a promising direction in understanding the treatment and prognosis of LUAD.
In this study, based on gene expression data of LUAD in The Cancer Genome Atlas (TCGA), we explored lncRNAs associated with ferroptosis, necroptosis, and pyroptosis for the first time and constructed a prognostic risk signature to identify different LUAD clusters. The TME, immune cell infiltration, therapeutic benefits, and prognosis of different clusters of LUAD were then systematically analyzed. The results suggest the risk signature is a reliable prognostic biomarker for LUAD and may contribute to its individualized treatment. We present the following article in accordance with the TRIPOD reporting checklist (available at https://jtd.amegroups.com/article/view/10.21037/jtd-22-1151/rc).
Methods
Data collection and study design
The clinical information and fragments per kilobase million (FPKM) format of RNA-sequencing (RNA-seq) profiles of LUAD patients were retrieved from TCGA on February 27, 2022 (https://portal.gdc.cancer.gov). To improve research accuracy, patients with missing overall survival (OS) or poor OS (less than 30 days) were excluded to reduce statistical bias in this analysis.
Based on gene expression data of LUAD in TCGA, ferroptosis, necroptosis, and pyroptosis-related lncRNAs (FNPRlncRNAs) were obtained by taking the intersection of ferroptosis-related lncRNAs (FRlncRNAs), necroptosis-related lncRNAs (NRlncRNAs), and pyroptosis-related lncRNAs (PRlncRNAs) differentially expressed in LUAD and normal tissues. Patients with complete survival information and expression data from TCGA database were randomly assigned to training and testing sets (1:1). Univariate, LASSO, and multivariate Cox regression analyses were used to establish a risk signature based on FNPRlncRNAs in the training set and then evaluated. A nomogram for prognostic prediction of individual LUAD patients was constructed based on risk score and clinical variables, such as age, gender, tumor stage. Gene set enrichment analysis (GSEA), single sample gene set enrichment analysis (ssGSEA), and ESTIMATE were used to analyze characteristics of the TME between subgroups. T-cell dysfunction and Exclusion (TIDE) scores were used to assess the response to immunotherapy in each risk subgroup. LUAD clusters were identified according to the risk signature, and the Molecular and immune characteristics between each cluster were evaluated. Finally, the half-maximal inhibitory concentration (IC50) was used to assess the sensitivity of each cluster to the chemotherapy and targeted drugs for the treatment. The study was conducted in accordance with the Declaration of Helsinki (as revised in 2013).
Identifying ferroptosis, necroptosis, and pyroptosis-related lncRNAs
A list of 388 ferroptosis-related genes (FRGs) was downloaded from FerrDb (version 7.4, http://www.zhounan.org/ferrdb/current/) (30), and a list of 74 necroptosis-related genes (NRGs) was obtained from previous literature (31). A list of 52 pyroptosis-related genes (PRGs) was obtained from prior literature (32), and these are displayed in Table S1. According to the expression data of LUAD in TCGA, 157 differentially expressed FRGs (DEFRGs), 25 differentially expressed NRGs (DENRGs), and 21 differentially expressed PRGs (DEPRGs) were obtained through the analysis of gene expression differences between LUAD and normal tissues using the Limma package of R [|log fold change (FC)| >0.585, false discovery rate (FDR) <0.05] (33). Then, with the correlation coefficient (|Pearson R|) >0.4 and P<0.001, 2,609 FRlncRNAs, 1,610 NRlncRNAs, and 906 PRlncRNAs were obtained by co-expression analysis of mRNAs and lncRNAs. We also analyzed the differential expression of these three sets of lncRNAs with |logFC| >1, FDR <0.05. Moreover, we intersected the sets of FRlncRNAs [2,609], NRlncRNAs [1,610], and PRlncRNAs [906] to obtain a set containing 567 ferroptosis, necroptosis, and pyroptosis-related lncRNAs (FNPRlncRNAs). Finally, through the differential expression analysis of FNPRlncRNAs in LUAD and normal tissues, 243 differentially expressed FNPRlncRNAs (DEFNPRlncRNAs) were obtained (|logFC| >1, FDR <0.05).
Establishing and validating the risk signature according to FNPRlncRNAs of LUAD
Patients with complete survival information and expression data from TCGA database were randomly assigned to training and testing sets (1:1), and the training set was used to construct a prognostic risk signature. First, DEFNPRlncRNAs significantly correlated with OS were screened by univariate Cox (uni-Cox) regression analysis combined with the clinical information of the training set (P<0.05). Then, to prevent overfitting during modeling, we performed minimum absolute contraction and selection operator (LASSO) analysis using the glmnet R package (using the penalty parameter estimated by 10-fold cross-validation) to screen out hub DEFNPRlncRNAs associated with LUAD prognosis (34). Finally, a prognostic risk signature constructed by optimal DEFNPRlncRNAs was established by multivariate Cox (multi-Cox) regression analysis. The risk score for each patient was obtained by multiplying the expression levels of the DEFNPRlncRNAs in the risk signature by the corresponding coefficients then adding them, and was calculated by the following formula:
According to the median risk value of patients in the training set, those in training, testing, and entire sets were divided into high- and low-risk subgroups. The survival package of R was employed to reveal differences in survival between high- and low-risk subgroups of each set respectively, and survival curves were plotted for visualization. The relationship between risk signature and clinical factors was demonstrated by boxplots. Uni-Cox and multi-Cox regression analyses investigated whether the risk signature was a potential independent prognostic indicator of LUAD. Receiver operating characteristic (ROC) curves were established, and the area under ROC curves (AUC) was calculated to evaluate the predictive value of the risk signature.
Nomogram and calibration
A nomogram was constructed using the RMS package of R to predict 1-, 3-, and 5-year survival of LUAD patients based on the risk signature and clinical variables such as age, sex, and tumor stage, while calibration curves verified the accuracy of the nomogram.
GSEA
GSEA was used to explore potential differences in biological function between risk subgroups (35). The genomes “c5.go.v7.4.symbols” and “c2.cp.kegg.v7.4.symbols” were obtained from MSigDB database (version 7.4) (http://www.gsea-msigdb.org/gsea/login.jsp). The adjusted P<0.05 was considered statistically significant, and the ClusterProfiler package of R was used for enrichment analysis (36).
TME of the risk signature
First, XCELL, EPIC, TIMER, QUANTISEQ, MCPCOUNTER, CIBERSORT-ABS, and CIBERSORT were used to calculate the correlation between immune infiltrating and the prognosis risk signature (37-42). According to the GSVA package of R, a ssGSEA was then used to score immune infiltrating cells and immune function in different subgroups (43). Finally, stromalscore, immunescore, and estimatescore (stromalscore + immunescore) were calculated for each patient using ESTIMATE to compare the TME in high- and low-risk subgroups (44).
Estimating the effect of immunotherapy
The ggpubr package of R was used to compare the expression of immune checkpoints between high- and low-risk subgroups (Wilcoxon signed-rank test, and P<0.05 was considered significant). Then, T cell dysfunction and exclusion (TIDE) was used to compare the response to immunotherapy between high- and low-risk subgroups (45).
Identifying LUAD clusters
ConsensusClusterPlus package was used to identify LUAD clusters (C1, C2, and C3) based on the expression levels of six DEFNPRlncRNAs (46), and Principal component analysis (PCA) and T-distributed Stochastic Neighbour Embedding (T-SNE) were used to visualize the distribution of LUAD clusters. The survival package of R was used to analyze the prognosis of patients in the three LUAD clusters (Kaplan-Meier method and log-rank test), and in the same way, we evaluated the immune microenvironment and clinical treatment of LUAD clusters C1, C2, and C3. First, we used XCELL, TIMER, QUANTISEQ, MCPCOUNTER, EPIC, CIBERSORT-ABS, and CIBERSORT to assess the correlation between C1, C2, and C3 clusters and immune cell infiltration. Following this, stromalscore, immunescore, and estimatescore were calculated for LUAD patients to compare the infiltration of immune cells and stromal cells among the three clusters, before the ggpubr package of R was used to compare the activation of immune checkpoint-related genes among the clusters. TIDE was also used to evaluate the response of the three LUAD clusters to immunotherapy. Finally, IC50 of anti-tumor agents in the three clusters was calculated using the pRRophetic package of R (47).
Evaluating chemotherapy and targeted therapy in three clusters
PRRophetic, an R package consisting of almost 700 cell lines and 138 drugs, predicts the response of clinical drugs to cancer based on tumor gene expression levels and has been revealed to be robust in predicting treatment responses in various clinical trials (48). We calculated IC50 of some common targeted and chemotherapeutic agents in three LUAD clusters (C1, C2, and C3) using pRRophetic package.
Statistical analysis
R4.1.3 software was used for all statistical data analyses, Wilcoxon test was utilized to analyze the differences between the two groups, and Kruskal-Wallis test was employed for differential analysis among the three groups. In addition, the relevant tests were performed using Spearman analysis, and survival curves were plotted using log-rank and Kaplan-Meier tests. The AUC was used to calculate the predictable score for binary data, the acceptable AUC threshold values is 0.5. P<0.05 was considered statistically significant.
Results
Identifying ferroptosis, necroptosis, and pyroptosis-related lncRNAs
The flow chart of this study is depicted in Figure 1. The differential expression of 157 DEFRGs, 25 DENRGs, and 21 DEPRGs in LUAD tissues and normal lung tissues are illustrated in Figure 2A, and the differential expressions of FRlncRNAs, NRlncRNAs, and PRlncRNAs in LUAD tissues and normal lung tissues are displayed in Figure 2B. The intersection of FRlncRNAs, NRlncRNAs, and PRlncRNAs yielded 567 FNPRlncRNAs, as illustrated in Figure 2C. The differential expressions of 243 DEFNPRlncRNAs in LUAD and normal lung tissues are depicted in Figure 2D.
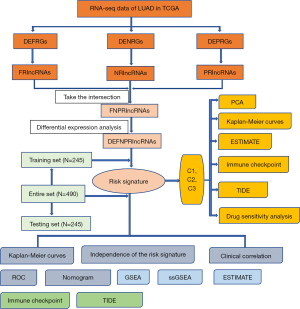
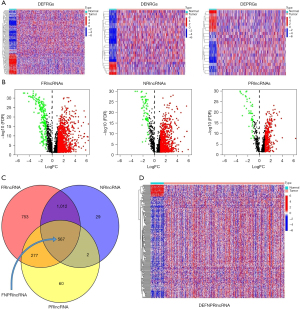
Construction of the risk signature
In the training set, 39 DEFNPRlncRNAs were confirmed to be associated with LUAD prognosis by uni-Cox analysis (Figure 3A, Table S2, P<0.05). The differential expression of these 39 lncRNAs between LUAD and normal tissues in TCGA samples is displayed in Figure S1, and the co-expression relationships between the 39 FNPRlncRNAs and FRGs, NRGs, and PRGs are shown in Figure 3B. After LASSO-penalised Cox regression analysis, a risk signature constructed by six FNPRlncRNAs was obtained (Figure 4A,4B), and their co-expression network with FRGs, NRGs, and PRGs is presented in Figure 4C. The risk score for each patient was calculated according to the following risk formula:
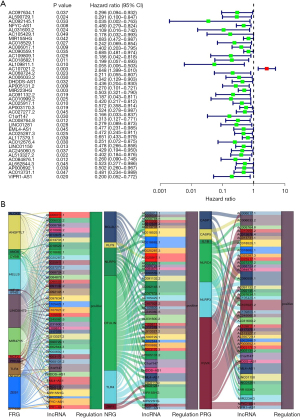
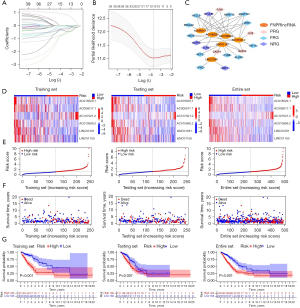
According to the median risk value of patients in the training set, those in training, testing, and entire sets were divided into high- and low-risk subgroups, respectively, and the expressions of the six FNPRlncRNAs in high- and low-risk subgroups are depicted in Figure 4D.
Evaluating the predictive capability of the risk signature
We found fewer deaths in the low-risk subgroup than in the high-risk subgroup in training, testing, and entire sets (Figure 4E,4F). Kaplan-Meier curve analysis revealed that in training, testing, and entire sets, the low-risk subgroup had a better prognosis than the high-risk subgroup (P<0.05) (Figure 4G). While the relationship between the risk score and clinical traits revealed no difference in risk score distribution among age, gender, and smoking population (P>0.05) (Figure 5A-5C), it was related to tumor stage (Figure 5D). In addition, the risk signature showed good prognostic ability in LUAD patients at different stages (stages I–II and III–IV) (Figure 5E,5F).
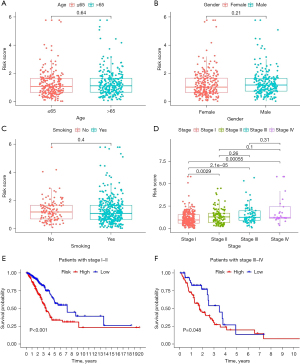
Uni-Cox regression and multi-Cox regression analyses confirmed the risk signature was an independent prognostic factor of LUAD (Figure 6A,6B). By comparing 1-year ROC curves established by gender, age, race, smoking, and tumor stage, the AUC value of the risk signature was the highest in the training set (0.742) (Figure 6C). The AUC values of 1-, 3-, and 5-year ROC generated by the risk signature in the training set were 0.742, 0.762, and 0.749, respectively (Figure 6D), The AUC values of 1-, 3-, and 5-year ROC generated by the risk signature in the testing set were 0.672, 0.642, and 0.563, respectively (not shown in Figure). and the AUC values of 1-, 3- and 5-year ROC in the entire set were 0.711, 0.699 and 0.665, respectively (Figure 6E). The results show our risk signature is helpful for prognostic prediction of LUAD patients.
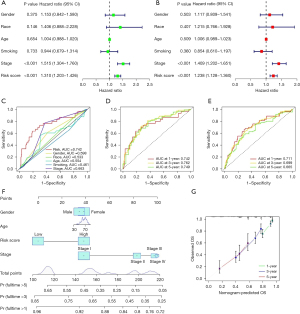
Nomogram and calibration
A nomogram was constructed based on the risk signature of patients and clinical variables such as age, gender, and tumor stage (Figure 6F), and calibration curves evaluated the accuracy of 1-, 3-, and 5-year nomogram survival predictions (Figure 6G).
GSEA
GSEA was used to identify the functions and pathways of gene sets enriched in the two risk subgroups, and pathway enrichment results are shown in Table S3. We found gene sets from high-risk score samples were enriched in pathways related to cell cycle, TCA cycle, DNA replication, ribosome, and spliceosome, and were enriched in functions related to cornification, keratinization, keratinocyte differentiation, epidermal cell differentiation, and mitotic sister chromatid separation. The gene sets of low-risk samples were enriched in pathways related to allograft rejection, asthma, the intestinal immune network for IGA production, primary immunodeficiency, and systemic lupus erythematosus, and were enriched in functions related to the spliceosomal TRI-snRNP complex assembly, DNA packaging complex, SM-like protein family complex, the small nuclear ribonucleoprotein complex, and T cell receptor complex (Figure 7A-7D).
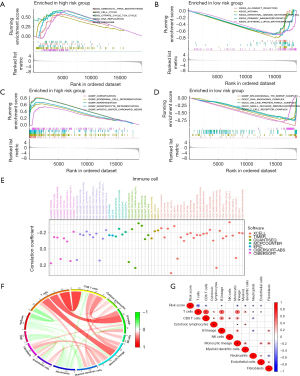
Estimating the tumor immune microenvironment of the risk signature
The correlation analysis results of seven calculation methods on immune infiltrating cells and the risk signature are shown in Figure 7E, and details of the association between immune-infiltrating cells and risk score obtained by CIBERSORT-ABS are shown in Figure S2. Further analysis of MCPCOUNTER revealed the risk score was negatively correlated with T cells, CD8+ T cells, B lineage, monocytic lineage, myeloid dendritic cells, neutrophils, and endothelial cells (Figure 7F-7G), and through ssGSEA analysis, we found B cells, CD8+ T cells, macrophages, dendritic cells (DCs), mast cells, neutrophils, T helper cells, Tfh, Th1 cells, Th2 cells, tumor-infiltrating lymphocytes (TIL), and Treg were more predominant in the low-risk subgroup. No significant difference was observed in natural killer cells between the two subgroups (Figure 8A), while the activity of 13 immune pathways in the low-risk subgroup was significantly higher than in the high-risk subgroup (Figure 8B). By estimating the TME scores, we also found stromalscore, immunescore, and estimatescore were higher in the low-risk subgroup than in the high-risk subgroup (Figure 8C). These results suggest the risk signature can be used to evaluate the tumor immune microenvironment in LUAD patients.
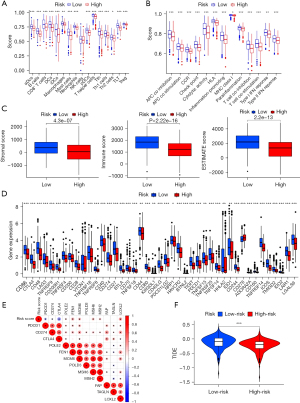
Estimating the effect of immunotherapy
By analyzing the expression of the immune checkpoint, we found the expression of most immune checkpoints in the low-risk subgroup including CD274, PDCD1, CTLA4, and LAG3 was significantly higher than in the high-risk subgroup (Figure 8D). Further analysis indicated the risk score was negatively correlated with the expressions of CD274, PDCD1, and CTLA4 but positively correlated with POLE2, FEN1, MCM6, MSH2, and LOXL2 (Figure 8E). In the analysis of the potential clinical efficacy of immunotherapy evaluated by TIDE, we found the TIDE score of the low-risk subgroup was higher than that of the high-risk subgroup (Figure 8F).
Identifying LUAD clusters
LUAD clusters (C1, C2, and C3) were identified according to the expression levels of the six FNPRlncRNAs constructing the risk signature (Figure 9A,9B), and principal component analysis (PCA) and T-distributed stochastic neighbour embedding (T-SNE) demonstrated the distribution relationship between LUAD cluster distribution and risk score (Figure 9C,9D). In addition, Kaplan-Meier analysis showed the OS of the three LUAD clusters was significantly different (Figure 9E). XCELL, TIMER, QUANTISEQ, MCPCOUNTER, EPIC, CIBERSORT-ABS, and CIBERSORT were used to analyze the infiltration of immune cells of C1, C2, and C3 clusters, and showed the C1 subgroup had the highest degree of immune cell infiltration (Figure S3). Additional analysis demonstrated the stromalscore, immunescore, and estimatescore of patients in C1 cluster were significantly higher than those in C2 and C3, while there was no significant difference between C2 and C3 (Figure 9F).
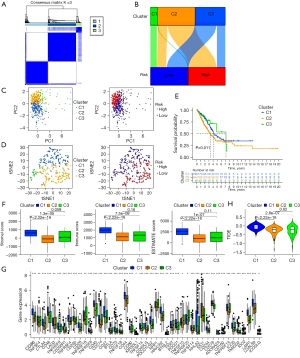
Predicting immunotherapy effect in the three clusters
Comparing the activation of immune checkpoint-related genes among the three LUAD clusters showed the expression of immune checkpoint-related genes in the C1 cluster was higher than in C2 and C3 clusters (Figure 9G). In addition, TIDE was used to assess the likelihood of these three clusters responding to immunotherapy, and the results revealed the TIDE score of the C1 subgroup was significantly higher than that of C2 and C3 subgroups, while there was no significant difference between the C2 and C3 subgroups (Figure 9H). These results suggest the risk score may be linked to immunotherapy and the C1 cluster may benefit most. We considered the absence of significant differences in immune cell infiltration, immune checkpoint activation, and TIDE scores between C2 and C3 might be due to the limited number of patients included in C3.
Evaluating chemotherapy and targeted therapy in the three subgroups
We used IC50 to assess the sensitivity of several common drugs in the three LUAD clusters. As presented in Figure 10, multiple chemotherapeutic agents and targeted therapy agents such as cisplatin, paclitaxel, nilotinib, sorafenib, sunitinib, and BIBW2992 (afatinib) showed different sensitivities in the three subgroups. This indicated the risk signature we constructed could provide help for more precise personalized medication for LUAD patients.
Discussion
At present, receiving effective treatment and obtaining a good prognosis remain the main challenges facing cancer patients. The TME is the direct site of tumor progression, immune escape, and immunotherapy response, and it is known that ferroptosis, necroptosis, and pyroptosis can modulate tumor immune processes by influencing the TME, respectively (22,49). Interestingly, extensive research has revealed interactions among these PCDs. For example, lytic cell death is the common endpoint of necroptosis and pyroptosis, and an essential protein called caspase-8 serves as a switch for both (23). ZBP1 functions as a fungal infection sensor to initiate pyroptosis and necroptosis (24), and the inactivation of RIP3 kinase, which plays a key role in necroptosis, can induce ferroptosis mediated by 15LOX/PEBP1 (25). Given the versatility of lncRNAs in the human body (50), they play an important role in the ferroptosis, necroptosis, and pyroptosis of tumor cells (27-29), and most literature has reported the prognostic value of prediction models based on ferroptosis-lncRNA, necroptosis-lncRNA, and pyroptosis-lncRNA in various cancers and their role in immunotherapy (51-55). FNPRlncRNA, which plays multiple identities in PCD, may be closely related to cancer development and prognosis, and its study may be a promising direction for understanding treatment and prognosis. Here, we first explored lncRNAs associated with ferroptosis, necroptosis, and pyroptosis in LAUD and constructed a risk signature.
In our study, uni-Cox regression and multi-Cox regression analyses confirmed the risk signature was an independent prognostic factor of LUAD, with patients with low-risk scores having a significantly better prognosis than those with high-risk scores. Training, testing, and entire sets confirmed the risk signature as a valuable biomarker that can be used to predict prognosis and guide treatment decisions. In addition, nomogram construction based on patient risk characteristics, age, gender, tumor stage, and other clinical variables is helpful for individualized assessment.
GSEA was used to assess the enrichment of functions and pathways in the two risk subgroups identified by the risk signature. The results indicated multiple pathways associated with immune-related diseases, functions associated with gene regulatory processes, and functions associated with T cell receptor complexes were all enriched in the low-risk subgroup. T cell antigen receptors (TCR) can help T cells effectively recognize autoantigens and non-autoantigens, including recognizing the inappropriate expression of endogenous proteins in cancerous tissues and affecting T cell differentiation and function (44). Such enrichment characteristics may be attributed to the effect of PCD on immune cell infiltration and immune activity. Previous studies have revealed enhanced immune infiltration induced by ferroptosis, necroptosis, or pyroptosis can enhance therapeutic effects (56-58). Necrotizing tumor cells can induce the maturation of bone marrow-derived DC after phagocytosis (17), and cells that undergo necrotic apoptosis are involved in immune system activation, particularly antigen presentation and cross-activation of CD8+ T cells (57,59). In addition, there is evidence CD8+ T cells inhibit tumor cells by inducing ferroptosis, necroptosis, and pyroptosis (22,60,61), and this study supports these conclusions. We analyzed the differences in tumor immune cell infiltration between the risk subgroups from multiple perspectives and found the infiltration of various immune cells, including CD8+ T cells, myeloid dendritic cells, and neutrophils in the low-risk subgroup was significantly higher than in the high-risk subgroup. Infiltrating immune cells constitute the main component of an immune microenvironment and are the core force of tumor immunity. Our results suggest the low-risk subgroup has a more favorable tumor immune microenvironment, which also predicts a better prognosis for patients in that subgroup.
ICIs, represented by PD-1 and CTLA-4 inhibitors, have benefited NSCLC patients in clinical trials (4,5), and the expression level of the immune checkpoint can affect the effectiveness of tumor immunotherapy (62). We analyzed the correlation between the model and the expression of common immune checkpoints, and the results revealed the expression of most was significantly higher in the low-risk subgroup than in the high-risk subgroup. In other words, the low-risk subgroup may have more resistant means to maintain a tumor-friendly immune environment in the face of tumor immunity to diminish the efficacy of immunotherapy. In addition, TIDE can analyze the mechanism of tumor immune escape, which involves inducing tumor T cell dysfunction in tumors with high cytotoxic T lymphocyte (CTL) infiltration and preventing T cell infiltration in tumors with a low CTL level, and predict its clinical efficacy based on the tumor expression profile before immunotherapy (45). Our analysis showed the low-risk group had a higher TIDE score, suggesting immune resistance and immune escape were more likely to occur in the low-risk subgroup than in the high-risk subgroup during immunotherapy. This suggests patients in the low-risk subgroup may benefit less from immunotherapy than those in the high-risk subgroup.Finally, three robust molecular clusters (C1, C2, and C3) were identified based on the expression of the six lncRNAs in the risk signature, and the C1 cluster presented the best advantage in immune infiltration compared to C2 and C3 clusters. In addition, the expression of immune checkpoints and TIDE scores suggest C1 clusters may benefit less from immunotherapy than C2 and C3 clusters. In addition, the pRRophetic package of R (47), which can calculate drug sensitivity based on gene expression in cancer cell lines, has been a significant aid to the assessment of drug sensitivity in numerous studies (63,64), and we evaluated the sensitivity of chemotherapy and targeted therapy agents in three LUAD subgroups. Our study provides a reference for the identification of different populations susceptible to chemotherapy, targeted therapy, and immunotherapy, which may help the development of individualized treatment for cancer.
We first explored lncRNAs associated with ferroptosis, necroptosis, and pyroptosis in LAUD and constructed a risk signature composed of FNPRlncRNAs, providing a novel approach to exploring biomarkers related to tumor prognosis and immunotherapy. In future clinical applications, our risk signature may provide an effective prediction of the immune landscape, therapeutic benefits, and prognosis of LUAD patients. However, this study has some limitations. First, the six lncRNAs involved in constructing the risk signature must be verified by specific biological studies. Second, to increase the reliability of the signature, subsequent effective external validation based on realistic studies is required and will be the direction of our subsequent research.
Conclusions
In conclusion, we have developed a biomarker according to lncRNAs associated with ferroptosis, necroptosis, and pyroptosis, which can effectively predict the prognosis of LUAD and may contribute to the individualized treatment of LUAD patients.
Acknowledgments
We sincerely acknowledged the contributions from the TCGA project.
Funding: None.
Footnote
Reporting Checklist: The authors have completed the TRIPOD reporting checklist. Available at https://jtd.amegroups.com/article/view/10.21037/jtd-22-1151/rc
Conflicts of Interest: All authors have completed the ICMJE uniform disclosure form (available at https://jtd.amegroups.com/article/view/10.21037/jtd-22-1151/coif). The authors have no conflicts of interest to declare.
Ethical Statement: The authors are accountable for all aspects of the work in ensuring that questions related to the accuracy or integrity of any part of the work are appropriately investigated and resolved. The study was conducted in accordance with the Declaration of Helsinki (as revised in 2013).
Open Access Statement: This is an Open Access article distributed in accordance with the Creative Commons Attribution-NonCommercial-NoDerivs 4.0 International License (CC BY-NC-ND 4.0), which permits the non-commercial replication and distribution of the article with the strict proviso that no changes or edits are made and the original work is properly cited (including links to both the formal publication through the relevant DOI and the license). See: https://creativecommons.org/licenses/by-nc-nd/4.0/.
References
- Sung H, Ferlay J, Siegel RL, et al. Global Cancer Statistics 2020: GLOBOCAN Estimates of Incidence and Mortality Worldwide for 36 Cancers in 185 Countries. CA Cancer J Clin 2021;71:209-49. [Crossref] [PubMed]
- Siegel RL, Miller KD, Jemal A. Cancer statistics, 2020. CA Cancer J Clin 2020;70:7-30. [Crossref] [PubMed]
- Chen Z, Fillmore CM, Hammerman PS, et al. Non-small-cell lung cancers: a heterogeneous set of diseases. Nat Rev Cancer 2014;14:535-46. [Crossref] [PubMed]
- Socinski MA, Jotte RM, Cappuzzo F, et al. Atezolizumab for First-Line Treatment of Metastatic Nonsquamous NSCLC. N Engl J Med 2018;378:2288-301. [Crossref] [PubMed]
- Antonia S, Goldberg SB, Balmanoukian A, et al. Safety and antitumour activity of durvalumab plus tremelimumab in non-small cell lung cancer: a multicentre, phase 1b study. Lancet Oncol 2016;17:299-308. [Crossref] [PubMed]
- Passaro A, Brahmer J, Antonia S, et al. Managing resistance to immune checkpoint inhibitors in lung cancer: treatment and novel strategies. J Clin Oncol 2022;40:598-610. [Crossref] [PubMed]
- Hadian K, Stockwell BR. SnapShot Ferroptosis. Cell 2020;181:1188-1188.e1. [Crossref] [PubMed]
- Yang WS. Regulation of ferroptotic cancer cell death by GPX4. Cell 2014;156:317-31. [Crossref] [PubMed]
- Galluzzi L, Kroemer G. Necroptosis: a specialized pathway of programmed necrosis. Cell 2008;135:1161-3. [Crossref] [PubMed]
- Dillon CP, Weinlich R, Rodriguez DA, et al. RIPK1 blocks early postnatal lethality mediated by caspase-8 and RIPK3. Cell 2014;157:1189-202. [Crossref] [PubMed]
- Chan FKM. Programmed necrosis/necroptosis: an inflammatory form of cell death. In: Wu H. editor. Cell Death. New York, NY: Springer; 2014:211-28.
- Cai Z, Jitkaew S, Zhao J, et al. Plasma membrane translocation of trimerized MLKL protein is required for TNF-induced necroptosis. Nat Cell Biol 2014;16:55-65. [Crossref] [PubMed]
- Humphries F, Shmuel-Galia L, Ketelut-Carneiro N, et al. Succination inactivates gasdermin D and blocks pyroptosis. Science 2020;369:1633-7. [Crossref] [PubMed]
- Wang K, Sun Q, Zhong X, et al. Structural Mechanism for GSDMD Targeting by Autoprocessed Caspases in Pyroptosis. Cell 2020;180:941-955.e20. [Crossref] [PubMed]
- Muendlein HI, Jetton D, Connolly WM, et al. cFLIPL protects macrophages from LPS-induced pyroptosis via inhibition of complex II formation. Science 2020;367:1379-84. [Crossref] [PubMed]
- Wang W, Green M, Choi JE, et al. CD8+ T cells regulate tumour ferroptosis during cancer immunotherapy. Nature 2019;569:270-4. [Crossref] [PubMed]
- Aaes TL, Kaczmarek A, Delvaeye T, et al. Vaccination with Necroptotic Cancer Cells Induces Efficient Anti-tumor Immunity. Cell Rep 2016;15:274-87. [Crossref] [PubMed]
- Wang YY, Liu XL, Zhao R. Induction of Pyroptosis and Its Implications in Cancer Management. Front Oncol 2019;9:971. [Crossref] [PubMed]
- Wang Q, Wang Y, Ding J, et al. A bioorthogonal system reveals antitumour immune function of pyroptosis. Nature 2020;579:421-6. [Crossref] [PubMed]
- Workenhe ST, Nguyen A, Bakhshinyan D, et al. De novo necroptosis creates an inflammatory environment mediating tumor susceptibility to immune checkpoint inhibitors. Commun Biol 2020;3:645. [Crossref] [PubMed]
- Wang G, Xie L, Li B, et al. A nanounit strategy reverses immune suppression of exosomal PD-L1 and is associated with enhanced ferroptosis. Nat Commun 2021;12:5733. [Crossref] [PubMed]
- Tang R, Xu J, Zhang B, et al. Ferroptosis, necroptosis, and pyroptosis in anticancer immunity. J Hematol Oncol 2020;13:110. [Crossref] [PubMed]
- Fritsch M, Günther SD, Schwarzer R, et al. Caspase-8 is the molecular switch for apoptosis, necroptosis and pyroptosis. Nature 2019;575:683-7. [Crossref] [PubMed]
- Banoth B, Tuladhar S, Karki R, et al. ZBP1 promotes fungi-induced inflammasome activation and pyroptosis, apoptosis, and necroptosis (PANoptosis). J Biol Chem 2020;295:18276-83. [Crossref] [PubMed]
- Lamade AM, Wu L, Dar HH, et al. Inactivation of RIP3 kinase sensitizes to 15LOX/PEBP1-mediated ferroptotic death. Redox Biol 2022;50:102232. [Crossref] [PubMed]
- Kapranov P, Cheng J, Dike S, et al. RNA maps reveal new RNA classes and a possible function for pervasive transcription. Science 2007;316:1484-8. [Crossref] [PubMed]
- Gai C, Liu C, Wu X, et al. MT1DP loaded by folate-modified liposomes sensitizes erastin-induced ferroptosis via regulating miR-365a-3p/NRF2 axis in non-small cell lung cancer cells. Cell Death Dis 2020;11:751. [Crossref] [PubMed]
- Tran DDH, Kessler C, Niehus SE, et al. Myc target gene, long intergenic noncoding RNA, Linc00176 in hepatocellular carcinoma regulates cell cycle and cell survival by titrating tumor suppressor microRNAs. Oncogene 2018;37:75-85. [Crossref] [PubMed]
- Liu J, Yao L, Zhang M, et al. Downregulation of LncRNA-XIST inhibited development of non-small cell lung cancer by activating miR-335/SOD2/ROS signal pathway mediated pyroptotic cell death. Aging (Albany NY) 2019;11:7830-46. [Crossref] [PubMed]
- Zhou N, Bao J. FerrDb: a manually curated resource for regulators and markers of ferroptosis and ferroptosis-disease associations. Database (Oxford) 2020;2020:baaa021. [Crossref] [PubMed]
- Xin S, Mao J, Duan C, et al. Identification and Quantification of Necroptosis Landscape on Therapy and Prognosis in Kidney Renal Clear Cell Carcinoma. Front Genet 2022;13:832046. [Crossref] [PubMed]
- Qi L, Xu R, Wan L, et al. Identification and Validation of a Novel Pyroptosis-Related Gene Signature for Prognosis Prediction in Soft Tissue Sarcoma. Front Genet 2021;12:773373. [Crossref] [PubMed]
- Ritchie ME, Phipson B, Wu D, et al. limma powers differential expression analyses for RNA-sequencing and microarray studies. Nucleic Acids Res 2015;43:e47. [Crossref] [PubMed]
- Simon N, Friedman J, Hastie T, et al. Regularization Paths for Cox's Proportional Hazards Model via Coordinate Descent. J Stat Softw 2011;39:1-13. [Crossref] [PubMed]
- Powers RK, Goodspeed A, Pielke-Lombardo H, et al. GSEA-InContext: identifying novel and common patterns in expression experiments. Bioinformatics 2018;34:i555-64. [Crossref] [PubMed]
- Yu G, Wang LG, Han Y, et al. clusterProfiler: an R package for comparing biological themes among gene clusters. OMICS 2012;16:284-7. [Crossref] [PubMed]
- Newman AM, Liu CL, Green MR, et al. Robust enumeration of cell subsets from tissue expression profiles. Nat Methods 2015;12:453-7. [Crossref] [PubMed]
- Marciniuk K, Trost B, Napper S. EpIC: a rational pipeline for epitope immunogenicity characterization. Bioinformatics 2015;31:2388-90. [Crossref] [PubMed]
- Aran D, Hu Z, Butte AJ. xCell: digitally portraying the tissue cellular heterogeneity landscape. Genome Biol 2017;18:220. [Crossref] [PubMed]
- Li T, Fan J, Wang B, et al. TIMER: A Web Server for Comprehensive Analysis of Tumor-Infiltrating Immune Cells. Cancer Res 2017;77:e108-10. [Crossref] [PubMed]
- Finotello F, Mayer C, Plattner C, et al. Molecular and pharmacological modulators of the tumor immune contexture revealed by deconvolution of RNA-seq data. Genome Med 2019;11:34. [Crossref] [PubMed]
- Becht E, Giraldo NA, Lacroix L, et al. Estimating the population abundance of tissue-infiltrating immune and stromal cell populations using gene expression. Genome Biol 2016;17:218. [Crossref] [PubMed]
- Hänzelmann S, Castelo R, Guinney J. GSVA: gene set variation analysis for microarray and RNA-seq data. BMC Bioinformatics 2013;14:7. [Crossref] [PubMed]
- Yoshihara K, Shahmoradgoli M, Martínez E, et al. Inferring tumour purity and stromal and immune cell admixture from expression data. Nat Commun 2013;4:2612. [Crossref] [PubMed]
- Jiang P, Gu S, Pan D, et al. Signatures of T cell dysfunction and exclusion predict cancer immunotherapy response. Nat Med 2018;24:1550-8. [Crossref] [PubMed]
- Wilkerson MD, Hayes DN. ConsensusClusterPlus: a class discovery tool with confidence assessments and item tracking. Bioinformatics 2010;26:1572-3. [Crossref] [PubMed]
- Geeleher P, Cox N, Huang RS. pRRophetic: an R package for prediction of clinical chemotherapeutic response from tumor gene expression levels. PLoS One 2014;9:e107468. [Crossref] [PubMed]
- Geeleher P, Cox NJ, Huang RS. Clinical drug response can be predicted using baseline gene expression levels and in vitro drug sensitivity in cell lines. Genome Biol 2014;15:R47. [Crossref] [PubMed]
- Niu X, Chen L, Li Y, et al. Ferroptosis, necroptosis, and pyroptosis in the tumor microenvironment: Perspectives for immunotherapy of SCLC. Semin Cancer Biol 2022;86:273-85. [Crossref] [PubMed]
- Liu SJ, Dang HX, Lim DA, et al. Long noncoding RNAs in cancer metastasis. Nat Rev Cancer 2021;21:446-60. [Crossref] [PubMed]
- Luo W, Wang J, Xu W, et al. LncRNA RP11-89 facilitates tumorigenesis and ferroptosis resistance through PROM2-activated iron export by sponging miR-129-5p in bladder cancer. Cell Death Dis 2021;12:1043. [Crossref] [PubMed]
- Tang Y, Li C, Zhang YJ, et al. Ferroptosis-Related Long Non-Coding RNA signature predicts the prognosis of Head and neck squamous cell carcinoma. Int J Biol Sci 2021;17:702-11. [Crossref] [PubMed]
- Huang J, Xu Z, Teh BM, et al. Construction of a necroptosis-related lncRNA signature to predict the prognosis and immune microenvironment of head and neck squamous cell carcinoma. J Clin Lab Anal 2022;36:e24480. [Crossref] [PubMed]
- Liu J, Geng R, Ni S, et al. Pyroptosis-related lncRNAs are potential biomarkers for predicting prognoses and immune responses in patients with UCEC. Mol Ther Nucleic Acids 2022;27:1036-55. [Crossref] [PubMed]
- Wang Z, Cao L, Zhou S, et al. Construction and Validation of a Novel Pyroptosis-Related Four-lncRNA Prognostic Signature Related to Gastric Cancer and Immune Infiltration. Front Immunol 2022;13:854785. [Crossref] [PubMed]
- Zhang Z, Zhang Y, Xia S, et al. Gasdermin E suppresses tumour growth by activating anti-tumour immunity. Nature 2020;579:415-20. [Crossref] [PubMed]
- Yatim N, Jusforgues-Saklani H, Orozco S, et al. RIPK1 and NF-κB signaling in dying cells determines cross-priming of CD8+ T cells. Science 2015;350:328-34. [Crossref] [PubMed]
- Ma X, Xiao L, Liu L, et al. CD36-mediated ferroptosis dampens intratumoral CD8+ T cell effector function and impairs their antitumor ability. Cell Metab 2021;33:1001-1012.e5. [Crossref] [PubMed]
- Biswas SK, Mantovani A. Macrophage plasticity and interaction with lymphocyte subsets: cancer as a paradigm. Nat Immunol 2010;11:889-96. [Crossref] [PubMed]
- Chen L, Niu X, Qiao X, et al. Characterization of Interplay Between Autophagy and Ferroptosis and Their Synergistical Roles on Manipulating Immunological Tumor Microenvironment in Squamous Cell Carcinomas. Front Immunol 2021;12:739039. [Crossref] [PubMed]
- Liao P, Wang W, Wang W, et al. CD8+ T cells and fatty acids orchestrate tumor ferroptosis and immunity via ACSL4. Cancer Cell 2022;40:365-378.e6. [Crossref] [PubMed]
- Hu FF, Liu CJ, Liu LL, et al. Expression profile of immune checkpoint genes and their roles in predicting immunotherapy response. Brief Bioinform 2021;22:bbaa176. [Crossref] [PubMed]
- Wang Z, Wang Y, Yang T, et al. Machine learning revealed stemness features and a novel stemness-based classification with appealing implications in discriminating the prognosis, immunotherapy and temozolomide responses of 906 glioblastoma patients. Brief Bioinform 2021;22:bbab032. [Crossref] [PubMed]
- Huang X, Ke K, Jin W, et al. Identification of Genes Related to 5-Fluorouracil Based Chemotherapy for Colorectal Cancer. Front Immunol 2022;13:887048. [Crossref] [PubMed]
(English Language Editor: B. Draper)