Breaking the mould, a first parse at natural language processing in aspergillosis diagnosis
Aspergillus sp. are a genus of ubiquitous mould of which the most common species is Aspergillus fumigatus. Exposure to Aspergillus is common, however disease, or aspergillosis, is largely a condition of the immunocompromised or those with underlying lung disease. Although the exact number of cases is difficult to determine, cases are rising with global estimates from 2017 suggesting that aspergillosis affects more than 14 million people worldwide (1).
The characteristics of aspergillosis depend on the interaction between the pathogen and the host. At one end of the spectrum is invasive aspergillosis (IA). This is most common in those with severe immunocompromise or critical illness. At particularly high risk are those with prolonged neutropenia, such as patients undergoing allogeneic haematopoietic stem cell transplant or chemotherapy, however solid organ transplant (particularly lung), prolonged exposure to high-dose corticosteroid, critical illness including influenza and coronavirus, chronic granulomatous disease, advanced acquired immune deficiency syndrome (AIDS), and advanced chronic obstructive pulmonary disease (COPD) are also well-documented risk factors (2,3). The manifestation of IA can be insidious in onset, variable, and is often challenging to discriminate from bacterial pneumonia. Haematogenous dissemination may result in presentation in multiple organs in the body including the kidneys, liver, spleen and the central nervous system. Confirmed diagnosis of IA is only possible through histopathological verification, however sampling is often impractical and risky. As such diagnosis instead relies on the amalgamation of multiple sources of information including a determination of pre-test probability based on identification of risk factors and clinic parameters, radiology, microbiological culture and indirect antigen testing (4-6). In practice it is often presumptive with a reliance on expert clinicians with adequate experience and a high index of suspicion to diagnose and treat IA. IA is often associated with diagnostic delay and in turn poor outcomes (7). It is the most common missed infection-related diagnosis on autopsy in intensive care unit (ICU) patients (8). Empirical use of anti-fungals for invasive fungal infections has been reported to be as high as 66% in a study of French onco-haematology patients (9). This has significant pharmacoeconomic impact, with azole resistance in Aspergillus additionally increasing (10).
Chronic pulmonary aspergillosis (CPA) is a spectrum of infections that are most common in those with underlying lung disease. The most common underlying condition worldwide is tuberculosis, however other associations include non-tuberculous mycobacteria, COPD, previously treated lung cancer, allergic bronchopulmonary aspergillosis (ABPA), sarcoid and pneumothorax (11). Although estimates of worldwide disease burden are challenging, there is likely a significant burden of undiagnosed disease in the developing world (12). Diagnosis is again based on an amalgamation of symptoms or radiology with serological or microbiological evidence of Aspergillus (13). The main challenges of CPA are the non-specific and indolent nature of the symptoms, high levels of respiratory co-morbidity and radiological changes that are often assigned to other, more common, pathologies. As for IA, this leads to diagnostic delay and disease progression.
At the other end of the spectrum to IA, is a hypersensitivity reaction to Aspergillus antigens. ABPA manifests with poorly controlled asthma, haemoptysis and systemic symptoms such as fever and malaise (14). Similar to IA and CPA, diagnosis is based on a combination of clinical, serological and radiological features (14). Early diagnosis is essential for preventing serious and potentially irreversible lung damage, however it is hampered by under-recognition due to significant cross-over with other, more common conditions (e.g., tuberculosis).
A recurring theme in all forms of aspergillosis its under-recognition and need for a ‘clinical expert’ to assimilate and enact complex diagnostic criteria and management. Although increasing in prevalence, aspergillosis, alongside other fungal diseases, is not something a general clinician is likely to have much experience or knowledge of. Reliance on an expert will obviously lead to delays, disparities and inequalities in healthcare, both within and between countries.
Li et al. attempt to overcome some of these challenges through utilisation of natural language processing (NLP) techniques and artificial intelligence (AI) to develop a quality control system for pulmonary aspergillosis (QCSA) (15). The overarching aim is to extract clinically relevant information from a patient record and present any perceived diagnostic or treatment deficits based on quality control points set by the system. The aim is that this would greatly reduce the need for time-consuming manual inspection of records by the clinician and help standardise diagnostic and treatment behaviours by making guideline-based suggestions.
They were able to test their system on 415 patient records covering 6 subtypes of pulmonary aspergillosis (noting that those with an unspecified subtype were excluded). A stratified sample of 200 records were then manually verified by three experts. They demonstrate an overall accuracy (proportion of correct predictions i.e., true positive or true negative) of 0.94. The positive predictive value for the QCSA correctly identifying a defect was 0.96.
This current study is commendable in many aspects. Firstly, it attempts to address an area with clear variability and poor outcomes in clinical practice, therefore potentially leading to significant clinical benefit. Secondly, they are attempting to use NLP and AI to address this problem, which is a rapidly growing field of clinical medicine and increasingly likely to become part of everyday clinical practice. Thirdly, the overall process by which they develop and validate their system is well described, allowing researchers to translate the methodology to other applicable areas of medicine. There are, however, some significant limitations in the study beyond those mentioned by the authors. They have attempted to develop a system that covers the whole spectrum of pulmonary aspergillosis. 26 quality control points are used to cover this large spectrum of disease, however it is not clear how these are distributed across the different subtypes. Furthermore, the stratified sample that was used for validation contains only very small numbers for some of the subgroups. Secondly, the system relies on a diagnostic coding reference for a pulmonary aspergillosis diagnosis, however it is not clear how the system manages records where an appropriate diagnostic code is not entered in patients with positive test results, therefore it is unclear how the system will help improve diagnostic yield. While the ambition of the authors is commendable in taking on this challenging topic, perhaps focusing on validating each Aspergillus related disease in turn (e.g., IA, CPA, ABPA) would enable a more detailed reflection of accuracy and clinical translatability.
Machine learning techniques and AI are an ever-growing aspect of clinical medicine, both in its application and research. NLP is a growing field within this, where the machine learns from specialists about how to process human language. Named entity recognition (NER) is a technique used to extract, identify, classify and find the attributes of various entities in unstructured human text. Together these methods can be used to extract data from unstructured text in electronic health records, such as demographics, co-morbidities, investigation results or radiological feature. They have the potential to consolidate vast amounts of information into a much more usable format, or to make models that can replicate aspects of medical expertise (Figure 1). This would allow doctors to allocate their time to patients more effectively, avoid mistakes, and ultimately provide improved outcomes and healthcare experience for patients. Furthermore, in less developed regions with limited medical resources they may provide access expertise not previously available. They also have a key role in research. Through processing millions of records a day they may reveal novel demographic, genomic, geographical or longitudinal trends and patterns which can be used, for example, to identify risk factors, endotypes for precision therapeutics or facilitate stewardship intervention (16-18).
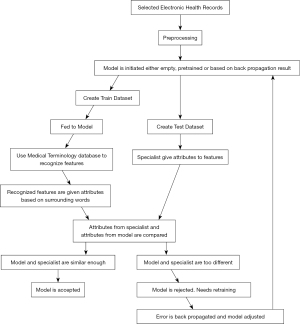
A major challenge of techniques such as NLP is the standardisation of terminology both between individuals, different healthcare environments and across different languages. For example, even within the United Kingdom, several different terminology databases exist, such as systematised nomenclature of medicine clinical terms (SNOMED) or Unified Medical Language System (UMLS). Different terminology databases might have different entries for the same concept. While synonymous terminologies are not a problem for the specialist, NLP and NER might not recognise the similarity between concepts. Despite this, however, techniques such as NLP have already shown promising results and outcomes across different hospitals, countries and languages, despite the grammatical, structural or lexicography differences. For example, it has been implemented in Germanic languages like English and German, Sinitic languages like Chinese and Italic languages like French (16-20).
Another challenge is overcoming the ambiguity or uncertainties in diagnosis or management guidelines, particularly those that depend heavily on the judgement of specialists. As such, the training and test datasets for the NLP might be very different from one hospital to another. At present NLP is largely deployed and trained at a small number of hospitals, with small sample sizes and a small number of specialists acting as validators. Great care and attention will be required to successfully navigate its transition across a wider remit. New systems and infrastructure will need to be built. Although the development and deployment of NLP is significantly cheaper and more scalable than training a specialist, it still requires a reliable system and network infrastructure. While cloud computing presents a possible solution to cheaply deploy and scale, cloud computing also leads to a higher risk for data security that will need to addressed.
Finally, machine learning and AI are not infallible. Even the best trained networks can sometimes display unexpected and erratic behaviour. If we want to build trust in our algorithms, they must be explainable and interpretable to both engineers and specialists to allow prompt recognition and correction of strange behaviour and biases, and to help design better systems in the future.
Fungal disease is a rapidly progressing field with great potential to incorporate NLP NER. By integrating machine learning of data such as radiological features or genomics databases, with newly emerging diagnostic techniques and clinical profiling, there is the potential to develop models to rapidly enhance the speed and accuracy of diagnosis, particularly in areas with limited access to specialists. Furthermore, standardising medical terminology and language into guidelines will allow the development of multicenter, international datasets, which are crucial to improving our understanding of what otherwise remains an under-researched group of diseases.
The QCSA developed by Li et al. demonstrates the utility of incorporating NLP NER into the challenging area of pulmonary fungal disease, and plants the seed for further research in this exciting area.
Acknowledgments
Funding: This work was supported by the MRC Clinical Academic Research Partnership award (MR/TOO5572/1 to AS) and by an MRC centre grant (MR/R015600/1 to AS).
Footnote
Provenance and Peer Review: This article was commissioned by the editorial office, Journal of Thoracic Disease. The article did not undergo external peer review.
Conflicts of Interest: All authors have completed the ICMJE uniform disclosure form (available at https://jtd.amegroups.com/article/view/10.21037/jtd-22-1393/coif). AS reports research grants from Vertex pharmaceuticals, Pfizer and Gilead Sciences. ZS reports research grant from Pfizer. AS and JP received speaker fees from Gilead Sciences and AS received speaker fees from Pfizer. The other author has no conflicts of interest to declare.
Ethical Statement: The authors are accountable for all aspects of the work in ensuring that questions related to the accuracy or integrity of any part of the work are appropriately investigated and resolved.
Open Access Statement: This is an Open Access article distributed in accordance with the Creative Commons Attribution-NonCommercial-NoDerivs 4.0 International License (CC BY-NC-ND 4.0), which permits the non-commercial replication and distribution of the article with the strict proviso that no changes or edits are made and the original work is properly cited (including links to both the formal publication through the relevant DOI and the license). See: https://creativecommons.org/licenses/by-nc-nd/4.0/.
References
- Bongomin F, Gago S, Oladele RO, et al. Global and Multi-National Prevalence of Fungal Diseases-Estimate Precision. J Fungi (Basel) 2017;3:57. [Crossref] [PubMed]
- Kousha M, Tadi R, Soubani AO. Pulmonary aspergillosis: a clinical review. Eur Respir Rev 2011;20:156-74. [Crossref] [PubMed]
- Armstrong-James D, Youngs J, Bicanic T, et al. Confronting and mitigating the risk of COVID-19 associated pulmonary aspergillosis. Eur Respir J 2020;56:2002554. [Crossref] [PubMed]
- Patterson TF, Thompson GR 3rd, Denning DW, et al. Practice Guidelines for the Diagnosis and Management of Aspergillosis: 2016 Update by the Infectious Diseases Society of America. Clin Infect Dis 2016;63:e1-60. [Crossref] [PubMed]
- Ullmann AJ, Aguado JM, Arikan-Akdagli S, et al. Diagnosis and management of Aspergillus diseases: executive summary of the 2017 ESCMID-ECMM-ERS guideline. Clin Microbiol Infect 2018;24:e1-38. [Crossref] [PubMed]
- Bassetti M, Azoulay E, Kullberg BJ, et al. EORTC/MSGERC Definitions of Invasive Fungal Diseases: Summary of Activities of the Intensive Care Unit Working Group. Clin Infect Dis 2021;72:S121-7. [Crossref] [PubMed]
- Falcone M, Massetti AP, Russo A, et al. Invasive aspergillosis in patients with liver disease. Med Mycol 2011;49:406-13. [Crossref] [PubMed]
- Winters B, Custer J, Galvagno SM Jr, et al. Diagnostic errors in the intensive care unit: a systematic review of autopsy studies. BMJ Qual Saf 2012;21:894-902. [Crossref] [PubMed]
- des Champs-Bro B, Leroy-Cotteau A, Mazingue F, et al. Invasive fungal infections: epidemiology and analysis of antifungal prescriptions in onco-haematology. J Clin Pharm Ther 2011;36:152-60. [Crossref] [PubMed]
- Risum M, Hare RK, Gertsen JB, et al. Azole resistance in Aspergillus fumigatus. The first 2-year's Data from the Danish National Surveillance Study, 2018-2020. Mycoses 2022;65:419-28. [Crossref] [PubMed]
- Smith NL, Denning DW. Underlying conditions in chronic pulmonary aspergillosis including simple aspergilloma. Eur Respir J 2011;37:865-72. [Crossref] [PubMed]
- Denning DW, Pleuvry A, Cole DC. Global burden of chronic pulmonary aspergillosis as a sequel to pulmonary tuberculosis. Bull World Health Organ 2011;89:864-72. [Crossref] [PubMed]
- Denning DW, Cadranel J, Beigelman-Aubry C, et al. Chronic pulmonary aspergillosis: rationale and clinical guidelines for diagnosis and management. Eur Respir J 2016;47:45-68. [Crossref] [PubMed]
- Agarwal R, Chakrabarti A, Shah A, et al. Allergic bronchopulmonary aspergillosis: review of literature and proposal of new diagnostic and classification criteria. Clin Exp Allergy 2013;43:850-73. [Crossref] [PubMed]
- Li Z, Wang X, Xu M, et al. Development and clinical application of an electronic health record quality control system for pulmonary aspergillosis based on guidelines and natural language processing technology. J Thorac Dis 2022;14:3398-407. [Crossref] [PubMed]
- Shickel B, Tighe PJ, Bihorac A, et al. Deep EHR: A Survey of Recent Advances in Deep Learning Techniques for Electronic Health Record (EHR) Analysis. IEEE J Biomed Health Inform 2018;22:1589-604. [Crossref] [PubMed]
- Liang H, Tsui BY, Ni H, et al. Evaluation and accurate diagnoses of pediatric diseases using artificial intelligence. Nat Med 2019;25:433-8. [Crossref] [PubMed]
- Wang H, Li Y, Khan SA, et al. Prediction of breast cancer distant recurrence using natural language processing and knowledge-guided convolutional neural network. Artif Intell Med 2020;110:101977. [Crossref] [PubMed]
- Wang Y, Sun Y, Ma Z, et al. Named Entity Recognition in Chinese Medical Literature Using Pretraining Models. Scientific Programming 2020;2020:1-9.
- Bannour N, Wajsbürt P, Rance B, et al. Privacy-preserving mimic models for clinical named entity recognition in French. J Biomed Inform 2022;130:104073. [Crossref] [PubMed]