Effects of common polymorphisms in miR-146a and miR-196a2 on lung cancer susceptibility: a meta-analysis
Introduction
An estimated 1.8 million new lung cancer (LC) cases occurred in 2012, accounting for about 13% of total cancer diagnoses and becoming the most frequently diagnosed cancer (1). LC is the leading cause of cancer death among males in both more and less developed countries, and has surpassed breast cancer as the leading cause of cancer death among females in more developed countries (1). Both environmental exposure factors and the genetic factors play important roles in the development of LC. The researchers have identified a great number of genetic variants suggesting to be potentially associated with LC risk (2-5). However, the associations between many genetic alterations and LC susceptibility were inconclusive and the reliable markers are still lacking.
MicroRNAs (miRNAs) are a class of small non-coding, single-stranded RNA molecules that form base-pairs with target messenger RNA (mRNAs), leading to negatively regulate their translational stability and efficiency (6). MiRNAs are considered to be involved in crucial biological processes, including organ development, cell differentiation, apoptosis, proliferation, cell growth regulation and tumorigenesis (7). More than 50% of miRNA genes are located in cancer-associated genomic regions or in fragile sites, suggesting that miRNAs may play an important role in the pathogenesis of human cancers, including LC, by regulating the expression of tumor suppressor genes or proto-oncogenes (8-10). Single nucleotide polymorphisms (SNPs) occurring in the miRNA gene region may have effects on the property and function of miRNAs, consequently contributing to cancer susceptibility by altering miRNA expression and/or maturation (11,12). Two common SNPs rs11614913 and rs2910164 have attracted wide attention in recent years. Rs11614913 (Homo sapiens, cytosine to thymine, C>T), located in precursor of hsa-miR-196a2, was reported to be associated with the risks of multiple kind of cancers, including LC (13-17). Rs2910164 (Homo sapiens, guanine to cytosine, G>C) of miR-146a was suggested to be related with the susceptibility of LC in some studies (13,16-19).
Until now, the role of SNPs in miRNAs on LC susceptibility remains unknown. There are some meta-analysis reports on the association between miRNA SNPs and risks of combined multiple kind of cancers (20-25). Some studies have implied that polymorphisms in miRNAs may have different effect on carcinogenesis in different organs, reflecting the diversities of the susceptible factors for different tumor types (25). Although there are subgroup analyses in different types of cancer, but LC is not included. In addition few meta-analyses were conducted among specific type of cancer, especially in LC. The published results of original studies on the association between miR-196a2 rs11614913 and miR-146a rs2910164 with LC risks were contradictory and inconclusive. So we perform this updated meta-analysis on all available studies to assess the LC risk with both rs2910164 in miR-146a and rs11614913 in miR-196a2.
Methods
Data sources
We conducted a systematic search using PubMed, EMBASE, ISI Web of Science, Cochrane library, ScienceDirect, Wiley Online Library, Chinese Biomedical Literature Database (CBM) and Chinese National Knowledge Infrastructure (CNKI) databases with the last search updated on April 1, 2016. The following searching terms were used: “microRNA OR miRNA OR miR-146a OR miR-196a2”, “lung cancer OR lung carcinoma OR lung tumor OR lung neoplasm” and “polymorphism OR SNP OR variation”. Searching was carried on without restriction on publication date and language. We evaluated potentially relevant publications by examining their titles and abstracts and all of the studies matching the eligible criteria were retrieved.
Study selection and data extraction
Eligible studies were selected according to the following inclusion criteria: evaluation of the rs11614913 and/or rs2910164 and LC risks; using an independent case-control or cohort study; offering the size of the samples in cases and controls; presenting useful allele and genotype frequencies for computing the odds ratios (ORs) with 95% confidence intervals (95% CIs). The major exclusion criteria were as follows: duplication of the published results; review and meta-analysis; studies on cell lines and gene expression.
The PRISMA checklist was conducted as the guideline and protocol of the meta-analysis (Figure 1) (26). Duplicate and obviously unrelated articles were eliminated by two investigators (YG Ren, XM Zhou). Three investigators (YG Ren, ZG Cui, G Hou) evaluated the abstracts of the remaining articles independently to determine whether the full-text article should be obtained and then extracted the data of included studies. The following information was sought from each publication: the name of the first author, year of publication, country origin, ethnicity, control characteristics, total number of cases and controls, allele and genotype frequencies for cases and controls, and genotyping method.
Statistical methods
Hardy-Weinberg equilibrium was firstly assessed for each study using Chi-square test in control groups and a P value <0.05 was considered significant disequilibrium. The strength of association between miRNA polymorphisms and susceptibility to LC was determined by calculating ORs with 95% CIs. The significance of the pooled ORs was assessed by the Z-test, and the results with P value <0.05 were considered statistically significant. Pooled ORs were obtained from combination of single study by heterozygote comparison [GC versus (vs.) GG for rs2910164; TC vs. TT for rs11614913], homozygote comparison (CC vs. GG for rs2910164; CC vs. TT for rs11614913), dominant model (CC/GC vs. GG for rs2910164; CC/CT vs. TT for rs11614913), recessive model (CC vs. GC + GG for rs2910164; CC vs. CT/TT for rs11614913) and allelic model (C vs. G for rs2910164; C vs. T for rs11614913) respectively. Subgroup analyses were investigated according to ethnicity, genotyping method and control characteristics for each genetic comparison model.
The heterogeneity among different studies were assessed by the Cochran’s Q test and quantified by I2 index and the results with P value <0.10 and/or I2≥50% indicated the existence of significant heterogeneity among the studies. To obtain summary statistics for ORs of miRNA polymorphism and cancer risk, we performed initial analyses with a fixed-effect model and confirmatory analyses with a random-effect model if there was significant heterogeneity. If the heterogeneity was significant, the result of the random-effect model was used, otherwise the result of the fixed-effect model was adopted.
The effect of publication bias was examined by Begg’s inverted funnel plots and Egger’s test. The significance of the intercept was determined by the t-test as suggested by Egger’s test. A P value <0.05 was considered representative of statistically significant publication bias.
All of P values were two-sided and all analyses were performed using the STATA software version 11.0 (STATA Corporation, College Station, TX, USA).
Results
Characteristics of eligible studies
A total of 95 articles were retrieved by literature search using different combinations of key terms. Flow chart of the study selection process was shown in Figure 1. Eight studies about cancer survival were excluded. Four cell line studies and nine gene expression studies were excluded. Ten studies of review and meta-analysis were excluded. One study was excluded because of data missing. Finally seven articles were included and used in quantitative synthesis for systematic review (13-19). All studies were published in English. These studies included ten data sets of two SNPs. Five data sets were about miR-146a (rs2910164) SNP, including 3,225 cases and 3,268 controls. There were 2,794 cases and 2,840 controls in five data sets for miR-196a2 (rs11614913) SNP. Of the seven studies, sample sizes ranged from 230 to 2,093. Almost all of the cases were histologically confirmed. Controls were mainly frequency matched by gender and age. The distribution of genotypes in the controls was all in Hardy-Weinberg equilibrium (HWE). The main characteristics of the included studies were presented in Table 1.
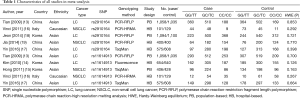
Full table
Meta-analysis of the association between SNP rs2910164 and susceptibility to lung cancer
The association between SNP rs2910464 in miR-146a and susceptibility to LC was evaluated in five independent studies. The results of the meta-analysis and heterogeneity are shown in Table 2. Q-tests in all of the five genetic models did not suggest notable heterogeneity. Therefore, the results of the fixed-effect models were used. The overall ORs with their 95% CIs showed the statistically significant association between SNP rs2910164 and LC risk in all of the genetic models except for heterozygote comparison (GC vs. GG: OR =1.08, 95% CI: 0.95–1.21, P=0.417 for heterogeneity, I2=0%; CC vs. GG: OR =1.30, 95% CI: 1.13–1.50, P=0.621 for heterogeneity, I2 = 0%; CC+GC vs. GG: OR =1.15, 95% CI: 1.02–1.29, P=0.419 for heterogeneity, I2=0%; CC vs. GC + GG: OR =1.27, 95% CI: 1.13–1.42, P=0.673 for heterogeneity, I2=0%; C vs. G: OR =1.15, 95% CI: 1.08–1.24, P=0.380 for heterogeneity, I2=0%). Figure 2 showed the meta-analysis result of the association between rs2910164 polymorphism and LC risk under the allele model (C vs. G).
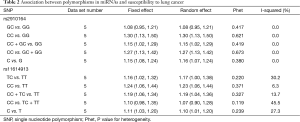
Full table
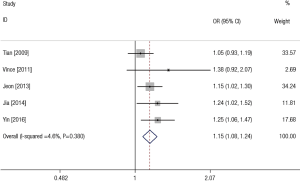
Table 3 showed the results of stratified analyses by ethnicity, genotyping method and control characteristics. The Asian carriers with CC genotype had 1.30-fold (OR =1.30, 95% CI: 1.13–1.50) increased risk of LC compared with the GG genotype carriers. Other comparisons also showed significantly increased LC risks (dominant model: OR =1.13, 95% CI: 1.00–1.27; recessive model: OR =1.27, 95% CI: 1.13–1.42; allele contrast: OR =1.15, 95% CI: 1.07–1.24). In the subgroup analyses based on genotyping method, the four studies using sequence-tagged techniques (PCR-restriction fragment length polymorphism and PCR-high resolution melting analysis) were combined and the statistically significant ORs were showed in three comparison (CC vs. GG: OR =1.25, 95% CI: 1.06–1.46; CC vs. GC + GG: OR =1.24, 95% CI: 1.09–1.41; C vs. G: OR =1.14, 95% CI: 1.05–1.23). In the subgroup analysis by control characteristics, the similar results were presented for the two studies using population-based control and three papers using hospital-based control, that is the significant associations in the four models except for the heterozygote comparison.
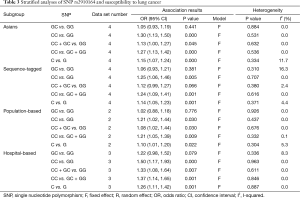
Full table
Meta-analysis of the association between rs11614913 and susceptibility to lung cancer
Overall, we found the significant associations of SNP rs11614913 with LC risk in all genetic models except recessive comparison (TC vs. TT: OR =1.16, 95% CI: 1.02–1.32, P=0.220 for heterogeneity, I2 =30.2%; CC vs. TT: OR =1.24, 95% CI: 1.06–1.44, P=0.371 for heterogeneity, I2 =6.3%; CC + TC vs. TT: OR = 1.19, 95% CI: 1.06–1.34, P=0.327 for heterogeneity, I2=13.7%; CC vs. TC + TT: OR =1.10, 95% CI: 0.98–1.35, P=0.119 for heterogeneity, I2=45.5%; C vs. T: OR =1.11, 95% CI: 1.03–1.20, P=0.239 for heterogeneity, I2=27.3%) (Table 2). Figure 3 showed the meta-analysis result of the association between rs11614913 polymorphism and LC risk under the allele model (C vs. T).
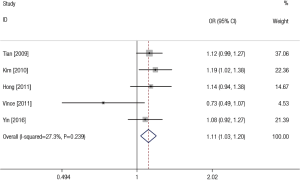
In the subgroup analysis by ethnicity, the results in all genetic models were statistically significant in Asians (Table 4). The subgroup analyses results were completely different between two types of genotyping methods. There were no significant associations between rs11614913 and LC risks combining the studies using sequence-tagged techniques to detect polymorphism. However, meta-analyses of the studies with fluorescence-based techniques presented the significant results in most of the comparisons. As for the subgroup analyses of control characteristics, the individual with CC genotype were more likely to develop LC than those carrying TT genotype (OR =1.26, 95% CI: 1.03–1.55) and C allele was suggested to be risk allele of LC (OR =1.13, 95% CI: 1.02–1.25) when the controls of the study were population-based. There were no significant results in the meta-analyses of the studies using hospital-based controls. Above results of stratified analyses by ethnicity, genotyping method and control characteristics were showed in Table 4.
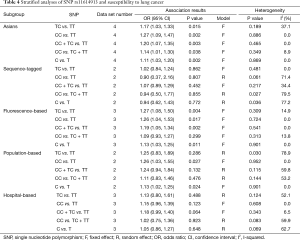
Full table
Sensitivity analysis
Every one single study included in our meta-analysis was sequentially deleted to detect the influence on the pooled ORs. The sensitivity analysis suggested no obvious effects from each study, which supported the robustness and reliability of our results.
Publication bias
No publication bias was detected by either the Begg’s inverted funnel plot or Begg’s test. The inverted funnel plot for the comparison of the two alleles of both rs2910164 and rs11614913 all seemed approximately symmetrical. The results were further identified by Egger’s test, which did not show significantly statistical evidence of publication bias (all P>0.05).
Discussion
Both environmental risk factors and individual susceptibility plays important role in the development of LC. Polymorphisms or mutations of genes involved in carcinogenesis may have accounted for the individual genetic susceptibility. Therefore, the association between SNP of genes and risks of LC has become a research focus in scientific community for a long time. However, the key gene is poorly understood after these years’ research. Recently miRNA has drawn increasing attention of scientists and some studies have been done to figure out the role of SNPs in precursor and mature miRNA as well as their influences on susceptibility of LC. The most common and widely studied SNPs in miRNAs were rs2910164 in miR-146a and rs11614913 in miR-196a2. Growing number of studies have been adopted to discuss the relationship between these two SNPs and the risks of LC. However, the results are contradictive and inconclusive. In order to better understand the association between these polymorphisms and LC risk, a meta-analysis with larger sample size and subgroup analysis is necessary. The present study is the largest meta-analysis of the association between miR-146a rs2910164 and miR-196a2 rs11614913 polymorphisms with the risk of LC.
There are some meta-analysis reports on the association between these two SNPs and risks of overall cancer without the analyses among LC (20-23,25). For LC, three meta-analyses were conducted to investigate miR-196a2 rs11614913 and only one to study miR-146a rs2910164 (27-29). However, the most recent update time of these three meta-analyses was September 2012 and there were not subgroup analyses in their results. So we perform an updated meta-analysis on all available studies to assess the LC risk with both rs2910164 in miR-146a and rs11614913 in miR-196a2 as well as the subgroup analyses by ethnicity, genotyping method and control characteristics. This study found the statistical evidence for the associations between these two SNPs (rs2910164 in miR-146a and rs11614913 in miR-196a2) and susceptibility to LC in both overall analysis and subgroup analyses. The three advantages of our meta-analysis, which are including new publications, undergoing subgroup analyses and without significant heterogeneity in our results, have enhanced the credibility of our study.
In the stratified analysis by ethnicity, the similar results were found for the two SNPs in Asians compared those in all population. The results in Caucasians have not presented because there is only one study in Caucasian (16). It is widely accepted that a sufficient sample is essential for genetic association studies so more and larger sample size studies in Caucasians are required in future. Similarly, for rs2910164 subgroup analysis was only done in studies using sequence-tagged techniques to detect SNPs but not done in studies using fluorescence-based techniques. Conversely, for rs11614913 subgroup analysis was adopted for both SNP techniques and the results were greatly different. We did not find any risk in sequence-tagged studies but the significant results were found in most of the results among Fluorescence-based studies. The reason may be that there is difference in the accuracy of these two techniques in SNP detecting or included studies are too few to conclude the stable results. As for stratified analyses by control source, the significant results were found both in in population-based studies and in hospital-based studies for rs2910164, however for rs11614913 the significant results were suggested in population-based studies but not in hospital-based studies. All studies included in this meta-analysis were retrospective and were significantly different in their study designs (population-based or hospital-based cases and control selection), which would have caused between-study heterogeneity and affected the conclusions. In addition, the observed different effects could be likely due to chance because studies with small sample size may have insufficient statistical power to detect a slight effect or may have generated a fluctuated risk estimate. So studies with larger sample size and different study designs are necessary to fully understand the relationship between the polymorphism and the risk of LC.
Despite our efforts in performing a comprehensive analysis, weakness of current meta-analysis was identified. First, our analysis used published international studies, which might arise publication bias, although the results for publication bias in our study were not statistically significant. Second, two SNPs were identified by case-control studies and no further validation was available. Third, lacking the original data of included studies limited our further evaluation of potential interactions, such as age, gender, family history, environmental factors and lifestyle. Fourth, there was only one study in Caucasian population and no study in African population.
In conclusion, our meta-analysis supports that the rs2910164 in miR-146a and the rs11614913 in miR-196a2 more likely contribute to LC risk. Future well-designed and larger sample size studies are of great value to confirm the findings. Moreover, interaction of genetic factors with environmental exposures should also be examined. The rs2910164 in miR-146a and the rs11614913 in miR-196a2 might be associated with susceptibility to LC.
Acknowledgements
None.
Footnote
Conflicts of Interest: The authors have no conflicts of interest to declare.
References
- Torre LA, Bray F, Siegel RL, et al. Global cancer statistics, 2012. CA Cancer J Clin 2015;65:87-108. [Crossref] [PubMed]
- Shieh Y, Eklund M, Sawaya GF, et al. Population-based screening for cancer: hope and hype. Nat Rev Clin Oncol 2016. [Epub ahead of print]. [Crossref] [PubMed]
- Sekido Y, Fong KM, Minna JD. Molecular genetics of lung cancer. Annu Rev Med 2003;54:73-87. [Crossref] [PubMed]
- Granville CA, Dennis PA. An overview of lung cancer genomics and proteomics. Am J Respir Cell Mol Biol 2005;32:169-76. [Crossref] [PubMed]
- Minna JD, Roth JA, Gazdar AF. Focus on lung cancer. Cancer Cell 2002;1:49-52. [Crossref] [PubMed]
- Bartel DP. MicroRNAs: genomics, biogenesis, mechanism, and function. Cell 2004;116:281-97. [Crossref] [PubMed]
- Bartel DP, Chen CZ. Micromanagers of gene expression: the potentially widespread influence of metazoan microRNAs. Nat Rev Genet 2004;5:396-400. [Crossref] [PubMed]
- Zhang B, Pan X, Cobb GP, et al. microRNAs as oncogenes and tumor suppressors. Dev Biol 2007;302:1-12. [Crossref] [PubMed]
- Yanaihara N, Caplen N, Bowman E, et al. Unique microRNA molecular profiles in lung cancer diagnosis and prognosis. Cancer Cell 2006;9:189-98. [Crossref] [PubMed]
- Calin GA, Croce CM. MicroRNA signatures in human cancers. Nat Rev Cancer 2006;6:857-66. [Crossref] [PubMed]
- Saunders MA, Liang H, Li WH. Human polymorphism at microRNAs and microRNA target sites. Proc Natl Acad Sci U S A 2007;104:3300-5. [Crossref] [PubMed]
- Chen K, Song F, Calin GA, et al. Polymorphisms in microRNA targets: a gold mine for molecular epidemiology. Carcinogenesis 2008;29:1306-11. [Crossref] [PubMed]
- Tian T, Shu Y, Chen J, et al. A functional genetic variant in microRNA-196a2 is associated with increased susceptibility of lung cancer in Chinese. Cancer Epidemiol Biomarkers Prev 2009;18:1183-7. [Crossref] [PubMed]
- Kim MJ, Yoo SS, Choi YY, et al. A functional polymorphism in the pre-microRNA-196a2 and the risk of lung cancer in a Korean population. Lung Cancer 2010;69:127-9. [Crossref] [PubMed]
- Hong YS, Kang HJ, Kwak JY, et al. Association between microRNA196a2 rs11614913 genotypes and the risk of non-small cell lung cancer in Korean population. J Prev Med Public Health 2011;44:125-30. [Crossref] [PubMed]
- Vinci S, Gelmini S, Pratesi N, et al. Genetic variants in miR-146a, miR-149, miR-196a2, miR-499 and their influence on relative expression in lung cancers. Clin Chem Lab Med 2011;49:2073-80. [Crossref] [PubMed]
- Yin Z, Cui Z, Ren Y, et al. Association between polymorphisms in pre-miRNA genes and risk of lung cancer in a Chinese non-smoking female population. Lung Cancer 2016;94:15-21. [Crossref] [PubMed]
- Jeon HS, Lee YH, Lee SY, et al. A common polymorphism in pre-microRNA-146a is associated with lung cancer risk in a Korean population. Gene 2014;534:66-71. [Crossref] [PubMed]
- Jia Y, Zang A, Shang Y, et al. MicroRNA-146a rs2910164 polymorphism is associated with susceptibility to non-small cell lung cancer in the Chinese population. Med Oncol 2014;31:194. [Crossref] [PubMed]
- Qiu LX, He J, Wang MY, et al. The association between common genetic variant of microRNA-146a and cancer susceptibility. Cytokine 2011;56:695-8. [Crossref] [PubMed]
- Wang J, Bi J, Liu X, et al. Has-miR-146a polymorphism (rs2910164) and cancer risk: a meta-analysis of 19 case-control studies. Mol Biol Rep 2012;39:4571-9. [Crossref] [PubMed]
- Xu W, Xu J, Liu S, et al. Effects of common polymorphisms rs11614913 in miR-196a2 and rs2910164 in miR-146a on cancer susceptibility: a meta-analysis. PLoS One 2011;6:e20471. [Crossref] [PubMed]
- Tian T, Xu Y, Dai J, et al. Functional polymorphisms in two pre-microRNAs and cancer risk: a meta-analysis. Int J Mol Epidemiol Genet 2010;1:358-66. [PubMed]
- Wang L, Qian S, Zhi H, et al. The association between hsa-miR-499 T>C polymorphism and cancer risk: a meta-analysis. Gene 2012;508:9-14. [Crossref] [PubMed]
- Yin Z, Yan L, Cui Z, et al. Effects of common polymorphisms rs2910164 in miR-146a and rs3746444 in miR-499 on cancer susceptibility: a meta-analysis. Mol Biol Rep 2013;40:3003-13. [Crossref] [PubMed]
- Moher D, Liberati A, Tetzlaff J, et al. Preferred reporting items for systematic reviews and meta-analyses: the PRISMA statement. PLoS Med 2009;6:e1000097. [Crossref] [PubMed]
- Chen Z, Xu L, Ye X, et al. Polymorphisms of microRNA sequences or binding sites and lung cancer: a meta-analysis and systematic review. PLoS One 2013;8:e61008. [Crossref] [PubMed]
- Yuan Z, Zeng X, Yang D, et al. Effects of common polymorphism rs11614913 in Hsa-miR-196a2 on lung cancer risk. PLoS One 2013;8:e61047. [Crossref] [PubMed]
- Wang G, Wang W, Gao W, et al. Two functional polymorphisms in microRNAs and lung cancer risk: a meta-analysis. Tumour Biol 2014;35:2693-9. [Crossref] [PubMed]