Diagnosis, clustering, and immune cell infiltration analysis of m6A-related genes in patients with acute myocardial infarction—a bioinformatics analysis
Introduction
According to the World Health Organization, cardiovascular disease has one of the highest mortality rates worldwide, and accounts for about 45% of all deaths worldwide (1). It is ranked first in terms of mortality in developing countries, such as China, and developed countries, such as European countries and the United States. Acute myocardial infarction (AMI) has been reported to account for half of all cardiovascular deaths (2,3). AMI is characterized by the rupture, ulceration, fissure, or erosion of atherosclerotic plaque, resulting in 1 or more intracoronary thrombi, which causes reduced myocardial blood flow and/or distal embolism and subsequent myocardial necrosis (4). The timely and correct diagnosis and early reperfusion are major factors affecting the prognosis and clinical outcome of AMI patients (5); however, in some AMI patients, the clog of the coronary artery cannot be observed via electrocardiogram or coronary angiography, which can lead to delayed or failed myocardial reperfusion, heart failure, malignant arrhythmias, myocardial reinfarction, and other major adverse cardiovascular events (6,7). Thus, early identification plays an important role in reducing the morbidity and mortality of AMI patients.
The diagnosis of AMI is currently based on physical examination and ischemic symptoms, electrocardiographic examination (ECG), and circulating levels of cardiac troponins (cTns). However, the diagnostic value of cTns may be limited by modest increases in their levels in the first few hours from AMI onset, since slight rises may be associated with non-ischemic elevation due to concomitant conditions such as heart and kidney failure (8). One of the most promising novel diagnostic biomarkers for AMI is miRNA (9,10). Ribonucleic acid (RNA) methylation modifications account for >60% of all RNA modifications, and N6-methyladenosine (m6A), which was discovered in 1974 (11), is the most prevalent modification of messenger RNAs (mRNAs) and long non-coding RNAs of higher organisms. Research has shown that m6A modifications occur in microRNAs, circular RNAs, ribosomal RNAs, transfer RNAs, and small nucleolar RNAs (12,13). M6A modifications mainly occur on adenines in PRACH sequences (R=A or G, H=A, Cm or U) (14), and their dynamic reversible processes (15) affect the splicing, transcription, translation, and degradation of mRNAs, which in turn regulate mammalian cell differentiation, immunity and metabolism. The biological function of m6A modification is mainly regulated by a combination of m6A methyltransferases (writers), m6A demethylases (erasers), and methylation recognition proteins (readers)(16).
A previous study found that m6A methylation is widely involved in the development of various types of cardiovascular diseases and plays an important regulatory role in heart failure, myocardial hypertrophy, atherosclerosis, and ischemic cardiomyopathy (17). m6A modification, as a pivotal regulator of messenger RNA stability, protein expression, and cellular processes, exhibits important roles in the development of cardiac remodeling and cardiomyocyte contractile function (18). AMI leads to cell death, which promotes the immune inflammatory response. Immune cells play an important role in the occurrence and development of AMI (19,20). A study had been conducted on the mechanism and relationship between m6A and AMI (14); however, no previous study has examined molecular typing based on m6A-related gene expression. In this study, we established a model for the diagnosis of AMI with m6A-related genes using a machine-learning method, and also performed molecular typing based on m6A-related gene expression and immune cell differences between type A and type B AMI by using clustering analysis. Our findings could provide a basis for the timely diagnosis and personalized treatment of patients with AMI. We present the following article in accordance with the TRIPOD reporting checklist (available at https://jtd.amegroups.com/article/view/10.21037/jtd-22-569/rc).
Methods
Study design
This is a case-control study. The study was conducted in accordance with the Declaration of Helsinki (as revised in 2013).
Participants
The GSE66360 data set comprised a total of 99 patients (49 patients with AMI and 50 normal control subjects). The inclusion criteria of the study group included: 1) adult patients; and 2) diagnosed with AMI.
Data source and preliminary processing
The platform data and probe data of the GSE66360 data set were downloaded from the Gene Expression Omnibus (GEO) database. The data were then organized by Perl software, and were subsequently analyzed by the R software with package “limma”.
Differential analysis of m6A-related gene expression in the peripheral blood of the normal subjects and patients with AMI
Based on the literature, 26 m6A-related genes (comprising 9 writers, 2 erasers, and 15 readers) were selected for this study (see Table 1). The expression data of the m6A-related genes were extracted by the R software limma package, and a differential gene expression analysis was conducted to compare the m6A-related genes in normal patients to those of patients with AMI. A |logFC| value >1, and a P<0.05 were considered statistically significant. The results were visualized by the pheat and ggplot2 packages. The chromosomal location of the m6A-related genes was depicted in circle diagram.
Table 1
Gene | Type |
---|---|
METTL3 | Writers |
METTL14 | Writers |
METTL16 | Writers |
WTAP | Writers |
VIRMA | Writers |
ZC3H13 | Writers |
RBM15 | Writers |
RBM15B | Writers |
CBLL1 | Writers |
YTHDC1 | Readers |
YTHDC2 | Readers |
YTHDF1 | Readers |
YTHDF2 | Readers |
YTHDF3 | Readers |
HNRNPC | Readers |
FMR1 | Readers |
LRPPRC | Readers |
HNRNPA2B1 | Readers |
IGFBP1 | Readers |
IGFBP2 | Readers |
IGFBP3 | Readers |
RBMX | Readers |
ELAVL1 | Readers |
IGF2BP1 | Readers |
FTO | Erasers |
ALKBH5 | Erasers |
Establishment of a diagnostic model of AMI based on the expression of the m6A-related genes
Random forest and support vector machine (SVM) are two common machine-learning methods for screening key factors. Random forest is an algorithm that integrates multiple trees through the idea of integrated learning. The basic unit of integrated learning is decision tree. It belongs to a major branch of machine learning; that is, the ensemble-learning method (21), which is also a method that is often used for omics analyses and to screen characteristics (22). SVM is a generalized linear classifier that performs the binary classification of data according to supervised learning, and its decision boundary is the maximum-margin hyperplane that solves the learning sample (23). SVM was also an early machine-learning method used in feature screening.
To obtain more accurate results, 2 models were calculated based on the m6A-related differentially expressed genes (DEGs). The superiority of the models was determined depending on the residual error, the reverse cumulative distribution of the residual error, and the area under the receiver operating characteristic (ROC) curve (AUC) (24). After the model was established, an importance distribution of the genes, a nomogram, a calibration plot, and a decision curve analysis (DCA) were visualized based on the results. The importance of the screened genes was evaluated, and the mean decrease in Mean Decrease Gini (MDG) was determined. The higher the value, the greater the importance of the gene in the model; a score >2 was used as the threshold for screening in this study.
Molecular typing based on the expression of m6A-related genes
“ConsensusClusterPlus”, which is usually used to determine the optimal number of K clusters for a data set, was used for the consensus clustering. The rationality of the consensus clustering was determined by a re-sampling–based approach for assessing the stability of the clusters (25). In this study, the conditions for clustering were as follows: maxK =9, reps =50, pItem =0.8, pFeature =1, clusterAlg = “pam”, seed =123456, and distance = “Euclidean” The optimal number of clusters was selected according to the heatmap of the consistency matrix after typing, the cumulative distribution function (CDF) of agreement, and the Delta plot judgment, and the values of the consistency matrix were expressed from white to dark blue, and ranged from 0 (impossible to cluster together) to 1 (always clustered together).
The consistency matrix was arranged according to the consistency classification (the tree above the heat map). The long bar between the tree diagram and the heat map was the category. The consistency cumulative distribution function plot indicated the cumulative distribution function when K takes different values, which was used to determine what value K takes when the CDF reaches an approximate maximum, when the clustering analysis results are the most reliable. The relative change of the area under the CDF de-line compared to k and k-1 is shown in the Delta area plot. The AUC increased slightly with the increase of K value of 1 score, and the value of K is the most appropriate (25). The DEGs associated with the diagnosis that had been obtained in the previous step were subjected to a principal component analysis (PCA) to construct the m6a-related gene markers. The m6a-related gene markers were also analyzed for each patient with AMI (i.e., a m6A score was calculated) (26).
Gene ontology (GO) and Kyoto Encyclopedia of Genes and Genomes (KEGG) analyses were conducted for the DEGs of the two types to observe the functions or pathways in which the DEGs were enriched. The GO annotation included the cellular components (CCs), molecular functions (MFs), and biological processes (BPs). The condition for enrichment was a q value filter <0.05.
Correlation between m6A-related gene expression molecular typing and immune cells
A single-sample gene set enrichment analysis (ssGSEA) is a special type of analyses that combined the immune.gmt data set with the “single sample gene set enrichment analysis (ssGSEA)” method and “Gaussian Discriminant Analysis (GDA)” to calculate the score of each sample (27). Using this approach, the degree of immune cell infiltration in each sample was determined, and the correlations between m6A-related gene expression and the infiltration amount of the immune cells were determined.
Statistical analysis
The diagnostic performance of the genes was assessed using area under the receiver operating characteristic (ROC) curve (AUC). The distribution of the differentially expressed genes was shown by heatmap and volcano map. A two-tailed P value <0.05 was considered as statistical significance. All the statistical analyses were performed by using R software (Version 4.1.1).
Results
Comparison of the differential expression analysis of the m6A-related genes in the peripheral blood between the patients with AMI and control group
The GSE6636 data set, which comprised 99 study subjects (49 patients with AMI and 50 normal control subjects), was downloaded from the GEO database. A flow chart of the study is shown in Figure 1. The DEG analysis identified the following 10 m6A-related genes by comparing the peripheral blood of the patients with AMI to that of the normal control subjects: 5 writers [Methyltransferase-like 3 (METTL3), Methyltransferase-like 14 (METTL14), Wilms tumor 1-associated protein (WTAP), Zinc Finger CCCH-Type Containing 13 (ZC3H13), and Casitas B-lineage proto-oncogene like 1 (CBLL1)], 4 readers [YT521-B homology domain-containing family 3 (YTHDF3), Fragile X mental retardation type 1 (FMR1), YT521-B homology-domain-containing protein 1 (YTHDC1), and insulin-like growth factor binding protein 3 (IGFBP3)] and 1 eraser [fat mass and obesity associated (FTO) gene]. The results are shown in Figure 2A,2B. As the circle diagram (see Figure 2C) shows, the m6A genes associated with AMI were mainly distributed on Chromosomes 4, 6, 13, 14, 16, 17, and X.
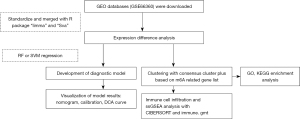
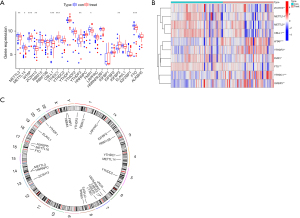
Establishment of the diagnostic model for AMI expressed by m6A-associated genes
The random-forest and SVM regression screening features were selected to build the model in this study. Based on the residual plot (see Figure 3A), the reverse cumulative distributions of the residuals (see Figure 3B), and the AUCs (the AUC of the model established by SVM was 0.918, and that of the model established by the random-forest method was 1.000) (see Figure 3C), the random-forest method was finally selected as the method for the establishment of the diagnostic model. In the random-forest model, which was established by selecting 135 trees with the MDG values of genes (see Figure 4A), 10 genes had MDG values >2. After considering many gene variables, the highest 5 genes (i.e., FTO, WTAP, YTHDC1, IGFBP3, and CBLL1) were selected for inclusion in the model. The model results are shown in Table 2. After the C-index was calculated as 0.842, the calibration (Figure 4B), nomogram (Figure 4C), and DCA curve (Figure 4D) showed the good fit of the model. The forest-tree diagram and clinical impact map are shown in Figure S1.
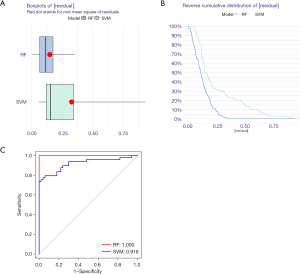
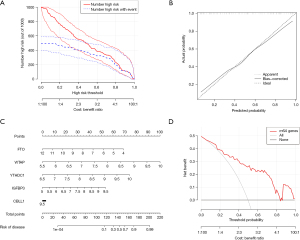
Table 2
Genes | Coefficient | 95% confidence interval of coefficient | P |
---|---|---|---|
Intercept | –28.48 | –44.16 to –12.81 | 0.0004 |
FTO | –0.69 | –1.27 to –0.11 | 0.0204 |
WTAP | 1.78 | 0.54 to 3.02 | 0.0049 |
YTHDC1 | 1.69 | 0.34 to 3.05 | 0.0142 |
IGFBP3 | 0.95 | 0.32 to 1.59 | 0.0033 |
CBLL1 | –0.04 | –0.49 to 0.42 | 0.8754 |
Results of molecular typing of patients with AMI based on m6A-related gene expression
Clusters 1–9 were analyzed by the R package “ConsensusClusterPlus”. The patients with AMI were divided into two subtypes by clustered heatmap (see Figure S2), CDF plot, and Delta area plot (see Figure S2) selection; 29 patients had Type A AMI, and 20 patients had Type B AMI (see Figure 5A). The PCA analysis showed that Type A and Type B AMI were well differentiated (see Figure 5B). The m6A-related genes were expressed in Type A and Type B (see Figure 5C,5D). Specifically, METTL3, ZC3H13, WTAP, and CBLL1 were highly expressed in Type A AMI, and YTHDF3 was highly expressed in Type B AMI. The differential gene GO annotation and the KEGG pathway analyses of Type A and Type B AMI showed that the BPs mainly included heart morphogenesis, the regulation of neuron differentiation, the regulation of the receptor signaling pathway via Janus Kinase-signal transducer and activator of transcription (JAK-STAT), the CCs mainly included the endoplasmic reticulum lumen, the intrinsic component of postsynaptic specialization, and germ plasm, and the MFs mainly included the germ plasm, signaling receptor activator activity, and receptor ligand activity (see Figure 5E,5F). The pathway mainly included the Herpes simplex virus 1 infection.
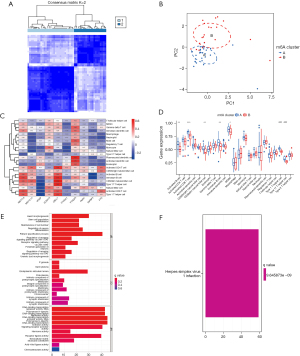
Results of the immune cell infiltration analysis based on molecular typing
The results of the ssGEEA analysis demonstrated that activated B cells, activated CD8+Tcells, CD56dim natural killer cells, immature B cells, myeloid-derived suppressor cells (MDSCs), Type 1 T helper cells, and Type 17 T helper cells had a higher proportion of infiltration in Type A AMI than in Type B (see Figure 6A). Further, METTL3, CBLL1, and YTHDF3 were positively correlated with immune cell infiltration (see Figure 6B), and the correlation was >0.4. A further analysis of the 3 genes of METTL3, CBLL1, and YTHDF3 in the high- and low-expression groups of the infiltration of immune cells (see Figure 6C-6E) showed that activated dendritic cells, eosinophils, gamma delta T cells, immature B cells, MDSCs, macrophages, plasmacytoid dendritic cells, and regulatory T cells were highly infiltrated in the YTHDF3 high-expression group. Additionally, activated B cells, activated CD4 T cells, activated CD8 T cells, CD56dim natural killer cells, immature B cells, Type 1 T helper cells, and Type 17 T helper cells were highly infiltrated in the CBLL1 high-expression group, while activated B cells, activated CD8 T cells, CD56dim natural killer cells, B cells, immature dendritic cells, MDSCs, Type 1 T helper cells, and Type 17 T helper cells were highly infiltrated in the METTL3 high-expression group.
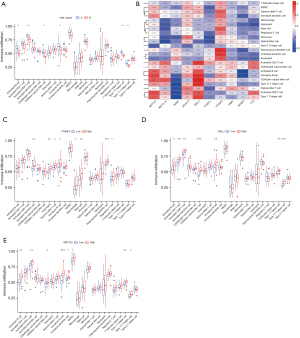
Discussion
In this study, the m6A-related genes were analyzed using a random-forest approach to establish a model for the diagnosis of AMI, for which the C index was 0.842. The calibration and DCA plots also showed the very good fit of the model. Similar to previous findings (18,28), the model showed that methylation was an important molecular change in the development of AMI. Under the model, the 5 most important genes were FTO, WTAP, YTHDC1, IGFBP3, and CBLL1. Further, we found that under a hypoxic environment, the decreased expression of FTO and demethylation activity led to increased m6A in RNA, and reduced the contraction of hypoxic cardiomyocytes, which in turn caused heart failure (29). WTAP (30), a component of methyltransferase, promoted endoplasmic reticulum stress and apoptosis by regulating the m6A modification of ATF4 mRNA, which in turn promoted myocardial injury. The YTHDC1YTH domain can recognize and bind to the m6A site in RNA, accelerate mRNA nuclear export, and promote the decay of specific transcripts (31). Long-term hypoxia enhanced IGFBP3 protein synthesis and induced its secretion. The accumulated IGFBP3 sequestered Insulin growth factor 1 (IGF-1) away from the type I IGF receptor (IGF-1 R), which blocked the IGF1R/PI3K/Akt survival signaling pathway, resulting in cell apoptosis (32).
In the clustering analysis, 49 patients with AMI were successfully divided into two subtypes. The m6A-related gene expression and immune cell infiltration differed significantly between the two subtypes. Notably, the m6A-related genes were generally more highly expressed in Type A AMI than Type B AMI, and immune cell infiltration was also more frequent in Type A AMI. The correlation between m6A modification and immune cell infiltration has also been studied in tumors(33,34). The present study showed that m6A modification was related to high immune cell infiltration and a poor prognosis. In a study by Yi et al. (35), the degree of infiltration of B cells, CD4+ T cells, and CD8+ T cells was negatively correlated with the risk score and positively correlated with the level of neutrophil, macrophage, and dendritic cell infiltration (P<0.001), which were in accordance with the results of our present study.
The correlation between the immune cell infiltration and 3 genes (i.e., METTL3, CBLL1, and YTHDF3) was around 0.6. However, few studies have been conducted on non-neoplastic diseases, and AMI have only recently received attention. For example, a study by Dubey et al. (36) reported that lipopolysaccharide leads to an increase in m6A RNA methylation and a corresponding decrease in FTO expression in mouse myocardium, which may promote myocardial inflammatory cytokine and immune system responses (37). Immune cells play an important role in the pathogenesis of myocardial infarction; for example, MDSCs (38) may play a beneficial and protective role in the process of ventricular remodeling after AMI by inhibiting the local inflammatory response and apoptosis mediated by inflammation. T cells are key regulators of the immune response in the development of many diseases (39), and T cell-mediated pathogenic immune responses play an important role in the inflammatory process of atherogenesis, which may contribute to plaque instability in patients with acute coronary syndromes (40).
The study was limited by the small number of cases in the selected data set. We had retrieved potential relevant data with larger sample size in the GEO database, but could not find eligible data regarding molecular types. In the development of bioinformatics, abundant bioinformatic analyses on tumors have been conducted. However, the relationship between m6A and common non-tumor diseases with very high mortality pathways and myocardial infarction diseases was established and satisfactory results were obtained.
In conclusion, a diagnostic model of AMI was established based on m6A-related gene expression. Additionally, 2 molecular subtypes were successfully identified, and the immune cell infiltration of each type was analyzed in this study. Our findings identified a novel biomarker for the accurate diagnosis of AMI and provide a theoretical basis for the personalized treatment of patients.
Acknowledgments
Funding: None.
Footnote
Reporting Checklist: The authors have completed the TRIPOD reporting checklist. Available at https://jtd.amegroups.com/article/view/10.21037/jtd-22-569/rc
Conflicts of Interest: All authors have completed the ICMJE uniform disclosure form (available at https://jtd.amegroups.com/article/view/10.21037/jtd-22-569/coif). The authors have no conflicts of interest to declare.
Ethical Statement: The authors are accountable for all aspects of the work in ensuring that questions related to the accuracy or integrity of any part of the work are appropriately investigated and resolved. The study was conducted in accordance with the Declaration of Helsinki (as revised in 2013).
Open Access Statement: This is an Open Access article distributed in accordance with the Creative Commons Attribution-NonCommercial-NoDerivs 4.0 International License (CC BY-NC-ND 4.0), which permits the non-commercial replication and distribution of the article with the strict proviso that no changes or edits are made and the original work is properly cited (including links to both the formal publication through the relevant DOI and the license). See: https://creativecommons.org/licenses/by-nc-nd/4.0/.
References
- Townsend N, Nichols M, Scarborough P, et al. Cardiovascular disease in Europe 2015: epidemiological update. Eur Heart J 2015;36:2673-4. [Crossref] [PubMed]
- Mullasari AS, Balaji P, Khando T. Managing complications in acute myocardial infarction. J Assoc Physicians India 2011;59:43-8. [PubMed]
- Wang L, Yu F. SCD leads to the development and progression of acute myocardial infarction through the AMPK signaling pathway. BMC Cardiovasc Disord 2021;21:197. [Crossref] [PubMed]
- Wereski R, Kimenai DM, Bularga A, et al. Risk factors for type 1 and type 2 myocardial infarction. Eur Heart J 2022;43:127-35. [Crossref] [PubMed]
- Park J, Choi KH, Lee JM, et al. Prognostic Implications of Door-to-Balloon Time and Onset-to-Door Time on Mortality in Patients With ST -Segment-Elevation Myocardial Infarction Treated With Primary Percutaneous Coronary Intervention. J Am Heart Assoc 2019;8:e012188. [Crossref] [PubMed]
- Lee SI, Lee SY, Choi CH, et al. Left Heart Decompression in Acute Complicated Myocardial Infarction During Extracorporeal Membrane Oxygenation. J Intensive Care Med 2017;32:405-8. [Crossref] [PubMed]
- Graf T, Desch S, Eitel I, et al. Acute myocardial infarction and cardiogenic shock: pharmacologic and mechanical hemodynamic support pathways. Coron Artery Dis 2015;26:535-44. [Crossref] [PubMed]
- Recchioni R, Marcheselli F, Olivieri F, et al. Conventional and novel diagnostic biomarkers of acute myocardial infarction: a promising role for circulating microRNAs. Biomarkers 2013;18:547-58. [Crossref] [PubMed]
- Zhou SS, Jin JP, Wang JQ, et al. miRNAS in cardiovascular diseases: potential biomarkers, therapeutic targets and challenges. Acta Pharmacol Sin 2018;39:1073-84. [Crossref] [PubMed]
- Wang Q, Liu B, Wang Y, et al. The biomarkers of key miRNAs and target genes associated with acute myocardial infarction. PeerJ 2020;8:e9129. [Crossref] [PubMed]
- Chen B, Khodadoust MS, Liu CL, et al. Profiling Tumor Infiltrating Immune Cells with CIBERSORT. Methods Mol Biol 2018;1711:243-59. [Crossref] [PubMed]
- Lee M, Kim B, Kim VN. Emerging roles of RNA modification: m(6)A and U-tail. Cell 2014;158:980-7. [Crossref] [PubMed]
- Bhat SS, Bielewicz D, Jarmolowski A, et al. N6-methyladenosine (m6A): Revisiting the Old with Focus on New, an Arabidopsis thaliana Centered Review. Genes (Basel) 2018;9:596. [Crossref] [PubMed]
- Dominissini D, Moshitch-Moshkovitz S, Schwartz S, et al. Topology of the human and mouse m6A RNA methylomes revealed by m6A-seq. Nature 2012;485:201-6. [Crossref] [PubMed]
- Dixit D, Prager BC, Gimple RC, et al. The RNA m6A Reader YTHDF2 Maintains Oncogene Expression and Is a Targetable Dependency in Glioblastoma Stem Cells. Cancer Discov 2021;11:480-99. [Crossref] [PubMed]
- Shi H, Wei J, He C. Where, When, and How: Context-Dependent Functions of RNA Methylation Writers, Readers, and Erasers. Mol Cell 2019;74:640-50. [Crossref] [PubMed]
- Li T, Zhuang Y, Yang W, et al. Silencing of METTL3 attenuates cardiac fibrosis induced by myocardial infarction via inhibiting the activation of cardiac fibroblasts. Faseb j 2021;35:e21162. [Crossref] [PubMed]
- Shi X, Cao Y, Zhang X, et al. Comprehensive Analysis of N6-Methyladenosine RNA Methylation Regulators Expression Identify Distinct Molecular Subtypes of Myocardial Infarction. Front Cell Dev Biol 2021;9:756483. [Crossref] [PubMed]
- Peet C, Ivetic A, Bromage DI, et al. Cardiac monocytes and macrophages after myocardial infarction. Cardiovasc Res 2020;116:1101-12. [Crossref] [PubMed]
- Weil BR, Neelamegham S. Selectins and Immune Cells in Acute Myocardial Infarction and Post-infarction Ventricular Remodeling: Pathophysiology and Novel Treatments. Front Immunol 2019;10:300. [Crossref] [PubMed]
- L B. Random forests. Mach Learn 2001;45:5-32. [Crossref]
- Acharjee A, Larkman J, Xu Y, et al. A random forest based biomarker discovery and power analysis framework for diagnostics research. BMC Med Genomics 2020;13:178. [Crossref] [PubMed]
- Halloran JT, Rocke DM. A Matter of Time: Faster Percolator Analysis via Efficient SVM Learning for Large-Scale Proteomics. J Proteome Res 2018;17:1978-82. [Crossref] [PubMed]
- Muschelli J. ROC and AUC with a Binary Predictor: a Potentially Misleading Metric. J Classif 2020;37:696-708. [Crossref] [PubMed]
- Wilkerson MD, Hayes DN. ConsensusClusterPlus: a class discovery tool with confidence assessments and item tracking. Bioinformatics 2010;26:1572-3. [Crossref] [PubMed]
- David CC, Jacobs DJ. Principal component analysis: a method for determining the essential dynamics of proteins. Methods Mol Biol 2014;1084:193-226. [Crossref] [PubMed]
- Xiao B, Liu L, Li A, et al. Identification and Verification of Immune-Related Gene Prognostic Signature Based on ssGSEA for Osteosarcoma. Front Oncol 2020;10:607622. [Crossref] [PubMed]
- Fernández-Sanlés A, Sayols-Baixeras S, Subirana I, et al. DNA methylation biomarkers of myocardial infarction and cardiovascular disease. Clin Epigenetics 2021;13:86. [Crossref] [PubMed]
- Mathiyalagan P, Adamiak M, Mayourian J, et al. FTO-Dependent N6-Methyladenosine Regulates Cardiac Function During Remodeling and Repair. Circulation 2019;139:518-32. [Crossref] [PubMed]
- Wang J, Zhang J, Ma Y, et al. WTAP promotes myocardial ischemia/reperfusion injury by increasing endoplasmic reticulum stress via regulating m6A modification of ATF4 mRNA. Aging (Albany NY) 2021;13:11135-49. [Crossref] [PubMed]
- Zhang B, Jiang H, Dong Z, et al. The critical roles of m6A modification in metabolic abnormality and cardiovascular diseases. Genes Dis 2021;8:746-58. [Crossref] [PubMed]
- Chang RL, Lin JW, Hsieh DJ, et al. Long-term hypoxia exposure enhanced IGFBP-3 protein synthesis and secretion resulting in cell apoptosis in H9c2 myocardial cells. Growth Factors 2015;33:275-81. [Crossref] [PubMed]
- Xu S, Tang L, Dai G, et al. Expression of m6A Regulators Correlated With Immune Microenvironment Predicts Therapeutic Efficacy and Prognosis in Gliomas. Front Cell Dev Biol 2020;8:594112. [Crossref] [PubMed]
- Tang R, Zhang Y, Liang C, et al. The role of m6A-related genes in the prognosis and immune microenvironment of pancreatic adenocarcinoma. PeerJ 2020;8:e9602. [Crossref] [PubMed]
- Yi L, Wu G, Guo L, et al. Comprehensive Analysis of the PD-L1 and Immune Infiltrates of m6A RNA Methylation Regulators in Head and Neck Squamous Cell Carcinoma. Mol Ther Nucleic Acids 2020;21:299-314. [Crossref] [PubMed]
- Dubey PK, Patil M, Singh S, et al. Increased m6A-RNA methylation and FTO suppression is associated with myocardial inflammation and dysfunction during endotoxemia in mice. Mol Cell Biochem 2022;477:129-41. [Crossref] [PubMed]
- Wang T, Tian J, Jin Y. VCAM1 expression in the myocardium is associated with the risk of heart failure and immune cell infiltration in myocardium. Sci Rep 2021;11:19488. [Crossref] [PubMed]
- Reddy K, Khaliq A, Henning RJ. Recent advances in the diagnosis and treatment of acute myocardial infarction. World J Cardiol 2015;7:243-76. [Crossref] [PubMed]
- Alroqi FJ, Chatila TA. T Regulatory Cell Biology in Health and Disease. Curr Allergy Asthma Rep 2016;16:27. [Crossref] [PubMed]
- Zhong Z, Wu H, Zhang Q, et al. Characteristics of T cell receptor repertoires of patients with acute myocardial infarction through high-throughput sequencing. J Transl Med 2019;17:21. [Crossref] [PubMed]
(English Language Editor: L. Huleatt)