Identification of hub genes and their correlation with immune infiltration in coronary artery disease through bioinformatics and machine learning methods
Introduction
Coronary artery disease (CAD) is a multifactorial chronic disease with complex pathology resulted from environmental and genetic factors as well as their interactions (1,2). CAD is characterized by the formation of plaques in the coronary arteries then atherosclerosis occurs. The mechanisms contributing to atherosclerosis are diverse, including dyslipidemia, hypercoagulability, endothelial dysfunction, oxidative stress and inflammation (2). There are multiple factors associated with CAD, containing age, gender, dyslipidemia, hypertension, smoking, diabetes mellitus, obesity and family history (3). In the previous study, researchers have found some biomarkers related to CAD, for instance cardiac troponin T, lipoprotein(a) [Lp(a)], C-reactive protein (CRP) and high-sensitive C-reactive protein (hs-CRP) (4-6). As the most common cardiovascular disease (CVD), CAD causes a heavy burden on human health globally (7-9). The morbidity and mortality of CAD has been continually rising in low- and middle-income countries and is now close to the level of that in developed counties, making it a global issue. According to the 2018 China CVD report, approximately 290 million people suffer from CVD and about 3.79% of them are CAD patients (10).
With rapid advancements in technology, the management of CAD is constantly being remodeled and is now more efficiently based on scientific classification and targeted treatment (11). Microarray analysis has been used as a practical method for studying changes in gene expression (12). The Gene Expression Omnibus (GEO) database (13) is a publicly available website supported by the National Center for Biotechnology Information (NCBI) and is used to identify key genes and potential mechanisms of the onset and development of diseases. Therefore, we can detect the gene expression information more efficiently and time-saving by conducting bioinformatics analysis.
CAD is life-threatening and in the stage of CAD initiation experts may miss diagnosis for the absence of typical symptoms (14). Meanwhile, the mechanisms of CAD remain still complicated and unclear. While coronary angiography is the gold standard diagnostic technique for CAD, it is invasive and costly. Therefore, combinations of more biomarkers need to be integrated using various methods for creating predictive, diagnostic, or prognostic tools for CAD. Machine learning (ML) has undergone an expansion in its application as a component of artificial intelligence (AI) and has enhanced the efficiency of the health care system (15). A previous study has shown that ML algorithms are effective for risk prediction, diagnosis, and imaging analysis of CVD (16). ML provides a more intelligent approach and increases confidence in the investigation of potential biomarkers compared to traditional methods.
The results of accumulating studies are helping researchers better understand the crucial role of immune cell infiltration in the onset and progression of CAD (17,18). CIBERSORT is widely used to investigate the expression of 22 subgroups of immune cells in order to determine the proportions of these immune cells in study samples (19).
The purpose of our study is to investigate the potential predictive biomarkers and provide fresh insights into the pathogenesis of CAD and direction for future studies of innovative therapies. If these potential biomarkers indicate the probability of occurrence of CAD accurately then early prevention can be carried out.
Methods
Overview of research procedures
In the present study, CAD-related gene chip data were obtained from GEO open resources. The GEO database was used to conduct bioinformatic analysis of differentially expressed genes (DEGs) between CAD patients and normal controls. Subsequently, we utilized 3 ML algorithms, including least absolute shrinkage and selection operator (LASSO), support vector machine recursive feature elimination (SVM-RFE), and random forest (RF) classifier, to screen optimal feature genes (OFGs) from DEGs for CAD. In addition, we investigated the correlation of OFGs with immune infiltration. The study was conducted in accordance with the Declaration of Helsinki (as revised in 2013).
Data collection and preprocessing
The gene expression profiles of datasets GSE66360, GSE61144, GSE60993, and GSE42418 were downloaded from the GEO (13) database (https://www.ncbi.nlm.nih.gov/geo/). GSE66360, GSE61144, and GSE60993, based on GPL570, GPL6106, and GPL6884, respectively, were used to identify the DEGs. GSE66360 contained 49 CAD samples and 50 control samples, GSE61144 consisted of 14 CAD and 10 samples, and GSE60993 was made up of 26 CAD and 7 control samples. The GSE66360, GSE61144, and GSE60993 datasets were normalized and the batch effect was eliminated using the “sva” package in R. We merged the 3 datasets to enlarge the sample size. Subsequently, the merged data became a gene expression profile of 89 CAD patients and 67 normal controls. The GSE42418 dataset, based on GPL13607, was used as the validation set and included 13 CAD samples and 11 control samples.
Identification of DEGs
To screen DEGs between patients and controls, the “limma” package in R was used. A P value <0.05 and fold change (FC) ≥2 (|log2FC| >1) was considered statistically different. Those with log2FC >0 were considered upregulated genes and log2FC <0 downregulated genes. Heatmaps and volcano plots for DEGs were carried out in R using “pheatmap” and “ggplot2” packages, respectively.
Functional enrichment analysis
Gene ontology (GO) analysis [comprising biological processes (BP), molecular functions (MF), and cellular components (CC)], Kyoto Encyclopedia of Genes and Genomes (KEGG) analysis, and Disease Ontology (DO) analysis were carried out using “clusterProfile”, “enrichplot”, “ggplot2”, “org.Hs.eg.db”, “GOplot”, and “DOSE” packages in R. A P value <0.05 was used as the threshold to screen significantly enriched GO terms, DO terms, and KEGG pathways.
Screening of OFGs
Three ML methods were used to screen OFGs. LASSO binomial logistic regression was used to select the OFGs from DEGs by applying the “glmnet” package in R. Optimal penalty parameter λ was determined by minimal binomial deviance. The SVM-RFE algorithm was used with “e1071”, “kernlab”, and “caret” packages in R to investigate the point with the smallest cross-validation error to select OFGs. The “randomForest” package in R was used for implementing the RF algorithm to identify the point where error was the most minimal. MeanDecreaseGini score >2 was used as the threshold to determine whether a gene was an OFG. In addition, a Venn diagram visualized the key OFGs obtained from the results of the 3 ML methods (LASSO, SVM-REF, and RF).
Construction of receiver operating characteristic (ROC) curves
The R package “pROC” was used to construct ROC curves and calculate the area under the curve (AUC) for hub genes.
Validation of the OFGs and ROC
The expression matrix of the GSE42418 dataset was used to verify each OFG as well as the ROC.
Infiltrating differential analysis of 22 immune cells
CIBERSORT algorithm in R was used to quantify the proportion of 22 types of immune cells in the merged dataset. We filtered out samples with P<0.05. A bar plot and violin diagram were used to visually represent differences in immune cells between CAD and normal samples.
Correlation analysis between OFGs and infiltrating immune cells
Relationships between the hub genes and infiltrating immune cells were investigated using R software. The analysis results were visualized using the “ggpubr” package in R.
Statistical analysis
All statistical analysis and graphics were conducted with R software (version 4.1.2). Differential expression analysis was performed with the cut-off threshold of P<0.05 and FC ≥2 or |log2FC| >1. A P value of less than 0.05 was two-sided and considered statistically significant.
Results
Identification of DEGs
Figure 1 shows an overview of the present study. We performed differential gene expression analysis (Figure 2) to investigate gene expression in CAD patients and normal controls. When comparing the blood samples of 89 CAD patients and 67 normal controls, 100 upregulated and 5 downregulated genes were identified in the merged dataset (GSE66360, GSE61144, and GSE60993). Figure 2 shows the volcano plot and heatmap for DEGs of the merged dataset.
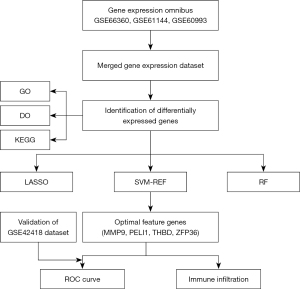
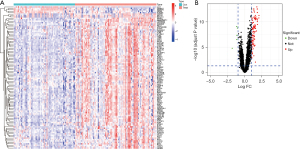
Functional enrichment analysis
GO analysis of the DEGs in the merged dataset revealed the top 10 most significantly enriched BP, CC, and MF items (Figure 3). In the BP category, upregulated DEGs were enriched in lipopolysaccharide (LPS); for CC, upregulated DEGs were significantly enriched in tertiary granule; and for MF, upregulated DEGs were enriched in pattern recognition receptor activity. KEGG pathway analysis was also performed and upregulated DEGs were abundantly enriched in the interleukin (IL)-17 signaling pathway, nuclear factor (NF)-kappa B signaling pathway, and tumor necrosis factor (TNF) signaling pathway. DO analysis indicated that the DEGs were enriched in lung disease, arteriosclerotic CVD, and atherosclerosis.
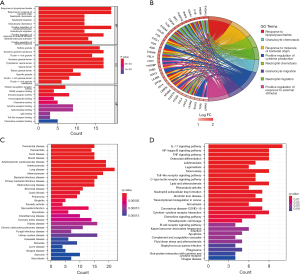
Screening OFGs
Eighteen genes were identified from the CAD-related DEGs using the LASSO algorithm (Figure 4), and 40 genes were selected using the SVM-REF algorithm. In addition, 8 genes were screened by the RF algorithm. After overlapping the hub genes obtained from the 3 ML methods, 4 candidate hub genes were identified: thrombomodulin (THBD), Pellino E3 ubiquitin protein ligase 1 (PELI1), matrix metallopeptidase 9 (MMP9), and zinc finger protein 36 (ZFP36). The AUC of ROC analysis (Figure 5) was 0.870 for THBD, 0.872 for PELI1, 0.847 for MMP9, and 0.839 for ZFP36.
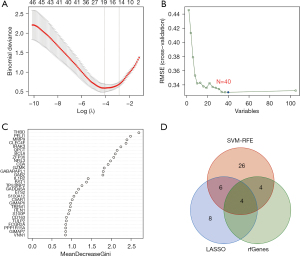
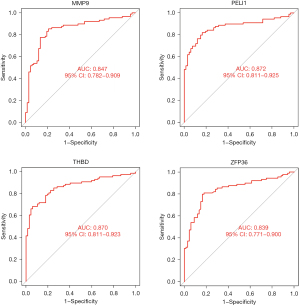
Verification of the OFGs
Validation was performed using the GSE42418 dataset [CAD patients (n=13), normal controls (n=11)] to evaluate whether the 4 hub genes were differentially expressed in CAD samples when compared with normal controls (Figure 6). The expression levels of both PELI1 and ZFP36 were higher in CAD patients than normal controls (P<0.05). However, there was no significant difference in the expression levels of MMP9 and THBD between CAD patients and normal controls. The results of ROC analysis (Figure 6) showed that AUC was 0.552 for THBD, 0.832 for PELI1, 0.727 for MMP9, and 0.769 for ZFP36.
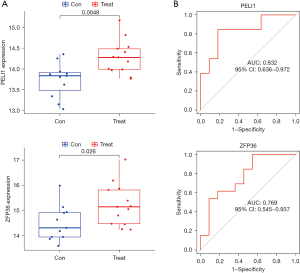
Immune infiltration analysis
The CIBERSORT algorithm was used to explore the relative proportion of 22 types of immune cells in samples from 88 CAD patients and 67 normal controls (Figure 7). The bar plot shows the contents of varied subpopulations in each individual clearly (Figure 7). The violin diagram shows that the proportion of memory B cells (P=0.019), CD8 T cells (P<0.001), resting memory CD4 T cells (P<0.001), regulatory T cells (P=0.028), and gamma delta T cells (P<0.001) in CAD samples were significantly lower than in normal control samples. However, activated memory CD4 T cells (P=0.014), resting natural killer (NK) cells (P<0.001), monocytes (P<0.001), M0 macrophages (P=0.023), activated mast cells (P<0.001), and neutrophils (P<0.001) in CAD samples were significantly higher than in normal control samples (Figure 7).
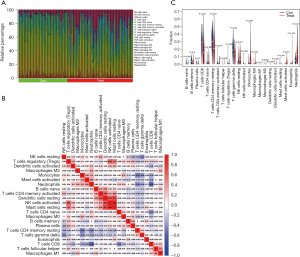
The correlation between MMP9, PELI1, THBD, and ZFP36 and immune cells
Correlation analysis between hub genes and immune cells (Figure 8) revealed that MMP9 had a significant positive correlation with neutrophils (r=0.652, P<0.001), monocytes (r=0.405, P<0.001), and M0 macrophages (r=0.402, P<0.001) and a negative correlation with CD8 T cells (r=−0.426, P<0.001). PELI1 was positively correlated with neutrophils (r=0.583, P<0.001) and activated mast cells (r=0.436, P<0.001) and negatively correlated with CD8 T cells (r=−0.405, P<0.001). THBD was positively correlated with neutrophils (r=0.573, P<0.001) and monocytes (r=0.432, P<0.001) and negatively correlated with CD8 T cells (r=−0.412, P<0.001). ZFP36 was positively correlated with neutrophils (r=0.555, P<0.001) and activated mast cells (r=0.432, P<0.001) and negatively correlated with CD8 T cells (r=−0.405, P<0.001). It can be concluded that MMP9, PELI1, THBD, and ZFP36 were all correlated with immune cells.
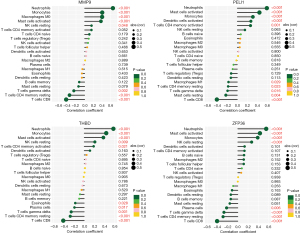
Discussion
Coronary heart disease (CHD) is characterized as a multifactorial disease and has become an economic burden globally (7). With rapid advances in technology, ML algorithms are being used to achieve a deeper understanding of clinical diagnoses, prediction and treatments through gene expression data (20). In the current study, we investigated the key feature genes associated with CAD by comparing differences in gene expression chips between CAD patients and normal controls. In this study, we combined 3 CAD datasets and then identified 105 DEGs and 4 upregulated hub genes (MMP9, PEIL1, THBD, and ZFP36) using bioinformatics analyses and ML methods, respectively. In addition, we performed functional enrichment. Further, we used the CIBERSORT algorithm to reveal that the 4 OFGs participated in immune cell infiltration.
GO enrichment analysis showed that the DEGs screened from the merged dataset were mainly related to LPS, tertiary granule, and pattern recognition receptor activity. Previous studies have demonstrated that LPS is related to heart injury. A study by Lepper et al. reported that the serum LPS-binding protein concentration in CAD patients was significantly increased compared with individuals without coronary atherosclerosis (21). A study conducted by Justo-Junior et al. found higher levels of chemokine and pattern-recognition receptor expressed in patients with unstable angina (22). According to KEGG pathways analysis, DEGs were abundantly enriched in the IL-17 signaling pathway, NF-kappa B signaling pathway, and TNF signaling pathway. It is already known that interferon gamma (IFN-γ) secreted by T cells is highly expressed in atherosclerotic lesions, and that regulation of the IL-17 signaling pathway plays a key role in atherosclerosis (23). NF-kappa B regulates the expression of genes targeting the initiation and progression of atherosclerosis. Under the action of NF-kappa B, multiple processes are integrated in the formation of atherosclerotic plaques (24). As one of the inflammatory markers, TNF was confirmed to be an indicator of increasing risk of CAD. The results of DO analysis indicated that the DEGs were enriched in lung disease, arteriosclerotic CVD, and atherosclerosis.
In recent years, the application of AI technology in CVD has made significant progress (20,25). It is important to consider whether the features selected at each point are true biomarkers or false positives (15). The OFGs obtained from ML algorithms may improve the efficiency of clinical diagnosis and prediction and provide more clues to guide doctors to make a diagnosis as ML methods can identify more complex, nonliner relationships. LASSO is a regression-based methodology, and it has the unique feature of penalizing the absolute value of a regression coefficient (26,27). SVM is a powerful ML method for building a classifier (28), and the present study used SVM-REF to screen OFGs because it can select relevant features using a separating hyperplane (28-30). The RF algorithm was used to screen feature items to obtain their importance ranking and is not vulnerable to overfitting. “Gini importance” is a measure of feature importance and is available in RF implementations (31,32). Our main aim in the present study was to screen the OFGs, not to develop a diagnostic or predictive tool. In this study, we preprocessed the original GEO data first, then investigated the OFGs through multiple feature selection algorithms. Therefore, by intergrading with LASSO, RF, and SVM-REF algorithms, 4 OFGs (MMP9, PELI1, THBD, and ZFP36) were eventually selected. Additionally, the SVM-REF and LASSO algorithms reduced the risk of overfitting through cross-validation. The selected 4 OFGs ranked highly in terms of importance.
MMP9, also known as GELB, CLG4B, MMP-9, and MANDP2, is a member of the matrix metalloproteinase family and is abundant in the interruption of extracellular matrix in normal physiological processes. MMP9 also plays an important role in disease processes, including in embryonic development, reproduction tissue remodeling, arthritis, and metastasis (33). Goerg et al. (34) reported that downregulation of MMP9 protein expression in the heart by empagliflozin could improve systolic heart function after myocardial infarction (MI) in rats. A study by Mujumdar et al. demonstrated that the activation of MMP9 decreased cardiac tensile strength (35). PELI1 can facilitate the activity of ubiquitin protein ligase, and it participates in negative regulation of necroptotic procedure, protein polyubiquitination, and reaction to LPS (36). A mouse model of MI confirmed that PELI1 was an important downstream target of vascular endothelial growth factor (VEGF), which can salvage impaired collateral blood vessel formation, diminish fibrosis, and improve myocardial function (37). Another study reported that PELI1 was a potential clinical marker for therapies to repair the damaged heart following MI in humans (38). THBD is an intronless gene and is also known as TM, THRM, AHUS6, BDCA3, CD141, BDCA-3, and THPH12. THBD encodes a protein that is an endothelial-specific type I membrane receptor and can bind thrombin. The combination of this protein and thrombin results in the activation of protein C, which degrades clotting factors Va and VIIIa and decreases the amount of thrombin generated. Mutations in THBD are a driver of thromboembolic disease, also known as inherited thrombophilia (39). A study from Iran (40) investigated the association of the rs1042579 single nucleotide polymorphism (SNP) in THBD with the risk of CVD and found that rs1042579 SNP could increase the risk of CVD. A study from Pakistan showed that the relationship between THBD and inflammatory cytokines in CAD helped to identify new prognostic and therapeutic targets for CVD treatments (41). ZFP36 is also known as TTP, G0S24, GOS24, TIS11, NUP475, zfp-36, and RNF162A, and it enables several functions, including 14-3-3 protein binding activity, heat shock protein binding activity, and mRNA 3'-UTR AU-rich region binding activity. Moreover, ZFP36 is extensively involved in cellular response to cytokine stimulation and growth factor stimulation as well as regulation of gene expression (42). Zhang et al. reported that ZFP36 was expressed in vascular endothelial cells and macrophage foam cells of atherosclerosis, and thereby ZFP36 expression may reduce vascular inflammation and prevent or treat atherosclerosis (43).
Atherosclerosis is characterized by hyperlipidemia and inflammation, and it is a major cause of CAD (11). Previous studies have shown that inflammatory macrophages and foam cell formation are crucial factors in atherosclerotic plague progression (44,45). T cells target the vessel wall in line with macrophages and react to antigens in the arterial wall (46). After activation of T cells, proinflammatory mediators are produced, exaggerating the inflammatory response, and disease development is worsened (47). In addition to macrophages and T cells, other innate and immune cells contribute to the pathogenesis of atherosclerosis, including neutrophils, B cells, and NK cells (48). Another study showed that CAD patients presented a highly activated CD+CXCR5+T cell subset (49). However, Olson et al. (50) evaluated innate and adaptive immune cells subsets in CHD patients and found that peripheral blood monocyte subsets were not strongly associated with CD4+ naive, memory, CD28−, or T helper cell subsets in MI or MI angina cases. CIBERSORT evaluation in the present study suggested that memory B cells, CD8 T cells, resting memory CD4 T cells, regulatory T cells, and gamma delta T cells in CAD samples infiltrated less than in normal control samples. On the contrary, activated memory CD4 T cells, resting NK cells, monocytes, M0 macrophages, activated mast cells, and neutrophils in CAD samples infiltrated more than in normal control samples. One previous study found that the number of neutral endopeptidase positive neutrophils was higher in acute MI patients with ruptured plaques compared with eroded plaques (51).
To further discover the relationship between OGFs and the main pathogenic mechanism of CAD, we analyzed the correlation between immune cells and the 4 selected OFGs. The present study revealed that MMP9 had a significant positive correlation with neutrophils, monocytes, and M0 macrophages, and a negative correlation with CD8 T cells. PELI1 was positively correlated with neutrophils and activated mast cells, and negatively correlated with CD8 T cells. THBD was positively associated with neutrophils and monocytes, while negatively correlated with CD8 T cells. ZFP36 was positively correlated with neutrophils and activated mast cells, and negatively correlated with CD8 T cells. It can be concluded from the results that MMP9, PELI1, THBD, and ZFP36 were all correlated with immune cells.
The current study had several strengths. Firstly, our approach identified 4 OFGs for CAD patients using 3 ML feature selection algorithms. The combined application of LASSO, SVM-RFE, and RF in this study to screen the OFGs associated with CAD reduced bias to the maximum extent. Secondly, we merged 3 different datasets to enlarge the sample size and removed the unqualified samples. In addition, we eliminated the batch effect between GEO datasets to make the statistical analyses more trustworthy. Finally, the expression levels of the 4 OFGs were validated in another independent dataset and showed good performance. However, several limitations in this study should be addressed. This was a bioinformatic analysis, and the identified hub genes as well as the interaction of these genes and immune cells need to be confirmed by functional validation in vitro and in vivo. Moreover, despite merging 3 GEO datasets, a larger sample is still needed for better results in the future.
Conclusions
In conclusion, 105 DEGs were identified using bioinformatics analyses, and 4 OFGs were obtained using 3 ML methods, providing a focus for further investigation of prediction for CAD. We investigated immune infiltration in CAD samples using CIBERSORT analysis and found a significant difference in immune infiltration between CAD and normal control samples. The relationship between OFGs and immune infiltration in the occurrence and development of CAD needs more in-depth study.
Acknowledgments
We thank the GEO datasets for providing data support to this study.
Funding: None.
Footnote
Conflicts of Interest: All authors have completed the ICMJE uniform disclosure form (available at https://jtd.amegroups.com/article/view/10.21037/jtd-22-632/coif). The authors have no conflicts of interest to declare.
Ethical Statement: The authors are accountable for all aspects of the work in ensuring that questions related to the accuracy or integrity of any part of the work are appropriately investigated and resolved.
Open Access Statement: This is an Open Access article distributed in accordance with the Creative Commons Attribution-NonCommercial-NoDerivs 4.0 International License (CC BY-NC-ND 4.0), which permits the non-commercial replication and distribution of the article with the strict proviso that no changes or edits are made and the original work is properly cited (including links to both the formal publication through the relevant DOI and the license). See: https://creativecommons.org/licenses/by-nc-nd/4.0/.
References
- Kuulasmaa K, Tunstall-Pedoe H, Dobson A, et al. Estimation of contribution of changes in classic risk factors to trends in coronary-event rates across the WHO MONICA Project populations. Lancet 2000;355:675-87. [Crossref] [PubMed]
- Mallika V, Goswami B, Rajappa M. Atherosclerosis pathophysiology and the role of novel risk factors: a clinicobiochemical perspective. Angiology 2007;58:513-22. [Crossref] [PubMed]
- Malakar AK, Choudhury D, Halder B, et al. A review on coronary artery disease, its risk factors, and therapeutics. J Cell Physiol 2019;234:16812-23. [Crossref] [PubMed]
- Saunders JT, Nambi V, de Lemos JA, et al. Cardiac troponin T measured by a highly sensitive assay predicts coronary heart disease, heart failure, and mortality in the Atherosclerosis Risk in Communities Study. Circulation 2011;123:1367-76. [Crossref] [PubMed]
- Gaubatz JW, Heideman C, Gotto AM Jr, et al. Human plasma lipoprotein [a]. Structural properties. J Biol Chem 1983;258:4582-9. [Crossref] [PubMed]
- Zakynthinos E, Pappa N. Inflammatory biomarkers in coronary artery disease. J Cardiol 2009;53:317-33. [Crossref] [PubMed]
- Virani SS, Alonso A, Benjamin EJ, et al. Heart Disease and Stroke Statistics-2020 Update: A Report From the American Heart Association. Circulation 2020;141:e139-596. [Crossref] [PubMed]
- Ades PA, Gaalema DE. Coronary heart disease as a case study in prevention: potential role of incentives. Prev Med 2012;55:S75-9. [Crossref] [PubMed]
- Roth GA, Johnson C, Abajobir A, et al. Global, Regional, and National Burden of Cardiovascular Diseases for 10 Causes, 1990 to 2015. J Am Coll Cardiol 2017;70:1-25. [Crossref] [PubMed]
- Ma LY, Chen WW, Gao RL, et al. China cardiovascular diseases report 2018: an updated summary. J Geriatr Cardiol 2020;17:1-8. [PubMed]
- Weber C, Noels H. Atherosclerosis: current pathogenesis and therapeutic options. Nat Med 2011;17:1410-22. [Crossref] [PubMed]
- Davis S, Meltzer PS. GEOquery: a bridge between the Gene Expression Omnibus (GEO) and BioConductor. Bioinformatics 2007;23:1846-7. [Crossref] [PubMed]
- Barrett T, Wilhite SE, Ledoux P, et al. NCBI GEO: archive for functional genomics data sets--update. Nucleic Acids Res 2013;41:D991-5. [Crossref] [PubMed]
- Kwok CS, Satchithananda D, Mallen CD. Missed opportunities in coronary artery disease: reflection on practice to improve patient outcomes. Coron Artery Dis 2022;33:233-8. [Crossref] [PubMed]
- Fernández-Ruiz I. Artificial intelligence to improve the diagnosis of cardiovascular diseases. Nat Rev Cardiol 2019;16:133. [Crossref] [PubMed]
- Bertsimas D, Mingardi L, Stellato B. Machine Learning for Real-Time Heart Disease Prediction. IEEE J Biomed Health Inform 2021;25:3627-37. [Crossref] [PubMed]
- Fernández-Ruiz I. Immune system and cardiovascular disease. Nat Rev Cardiol 2016;13:503. [Crossref] [PubMed]
- Fernandez DM, Giannarelli C. Immune cell profiling in atherosclerosis: role in research and precision medicine. Nat Rev Cardiol 2022;19:43-58. [Crossref] [PubMed]
- Newman AM, Steen CB, Liu CL, et al. Determining cell type abundance and expression from bulk tissues with digital cytometry. Nat Biotechnol 2019;37:773-82. [Crossref] [PubMed]
- Mathur P, Srivastava S, Xu X, et al. Artificial Intelligence, Machine Learning, and Cardiovascular Disease. Clin Med Insights Cardiol 2020;14:1179546820927404. [Crossref] [PubMed]
- Lepper PM, Schumann C, Triantafilou K, et al. Association of lipopolysaccharide-binding protein and coronary artery disease in men. J Am Coll Cardiol 2007;50:25-31. [Crossref] [PubMed]
- Justo-Junior AS, Villarejos LM, Lima XTV, et al. Monocytes of patients with unstable angina express high levels of chemokine and pattern-recognition receptors. Cytokine 2019;113:61-7. [Crossref] [PubMed]
- Taleb S, Tedgui A, Mallat Z. IL-17 and Th17 cells in atherosclerosis: subtle and contextual roles. Arterioscler Thromb Vasc Biol 2015;35:258-64. [Crossref] [PubMed]
- Baker RG, Hayden MS, Ghosh S. NF-κB, inflammation, and metabolic disease. Cell Metab 2011;13:11-22. [Crossref] [PubMed]
- Johnson KW, Torres Soto J, Glicksberg BS, et al. Artificial Intelligence in Cardiology. J Am Coll Cardiol 2018;71:2668-79. [Crossref] [PubMed]
- McEligot AJ, Poynor V, Sharma R, et al. Logistic LASSO Regression for Dietary Intakes and Breast Cancer. Nutrients 2020;12:2652. [Crossref] [PubMed]
- Omranian N, Eloundou-Mbebi JM, Mueller-Roeber B, et al. Gene regulatory network inference using fused LASSO on multiple data sets. Sci Rep 2016;6:20533. [Crossref] [PubMed]
- Huang S, Cai N, Pacheco PP, et al. Applications of Support Vector Machine (SVM) Learning in Cancer Genomics. Cancer Genomics Proteomics 2018;15:41-51. [PubMed]
- Brown MP, Grundy WN, Lin D, et al. Knowledge-based analysis of microarray gene expression data by using support vector machines. Proc Natl Acad Sci U S A 2000;97:262-7. [Crossref] [PubMed]
- Jiang H, Gu J, Du J, et al. A 21-gene Support Vector Machine classifier and a 10-gene risk score system constructed for patients with gastric cancer. Mol Med Rep 2020;21:347-59. [PubMed]
- Su X, Xu Y, Tan Z, et al. Prediction for cardiovascular diseases based on laboratory data: An analysis of random forest model. J Clin Lab Anal 2020;34:e23421. [Crossref] [PubMed]
- Strobl C, Malley J, Tutz G. An introduction to recursive partitioning: rationale, application, and characteristics of classification and regression trees, bagging, and random forests. Psychol Methods 2009;14:323-48. [Crossref] [PubMed]
- Barleon B, Sozzani S, Zhou D, et al. Migration of human monocytes in response to vascular endothelial growth factor (VEGF) is mediated via the VEGF receptor flt-1. Blood 1996;87:3336-43. [Crossref] [PubMed]
- Goerg J, Sommerfeld M, Greiner B, et al. Low-Dose Empagliflozin Improves Systolic Heart Function after Myocardial Infarction in Rats: Regulation of MMP9, NHE1, and SERCA2a. Int J Mol Sci 2021;22:5437. [Crossref] [PubMed]
- Mujumdar VS, Smiley LM, Tyagi SC. Activation of matrix metalloproteinase dilates and decreases cardiac tensile strength. Int J Cardiol 2001;79:277-86. [Crossref] [PubMed]
- Chang M, Jin W, Sun SC. Peli1 facilitates TRIF-dependent Toll-like receptor signaling and proinflammatory cytokine production. Nat Immunol 2009;10:1089-95. [Crossref] [PubMed]
- Thirunavukkarasu M, Selvaraju V, Joshi M, et al. Disruption of VEGF Mediated Flk-1 Signaling Leads to a Gradual Loss of Vessel Health and Cardiac Function During Myocardial Infarction: Potential Therapy With Pellino-1. J Am Heart Assoc 2018;7:e007601. [Crossref] [PubMed]
- Zhao Q, Yang J, Chen H, et al. Peli1 induction impairs cardiac microvascular endothelium through Hsp90 dissociation from IRE1α. Biochim Biophys Acta Mol Basis Dis 2019;1865:2606-17. [Crossref] [PubMed]
- Conway EM. Thrombomodulin and its role in inflammation. Semin Immunopathol 2012;34:107-25. [Crossref] [PubMed]
- Khosravi E, Sadeghian L, Mohamadynejad P, et al. Association study of polymorphism in Thrombomodulin gene rs1042579 with cardiovascular disease. Acta Biomed 2022;92:e2021282. [PubMed]
- Rafiq M, Liaquat A, Saeed N, et al. Gene expression of thrombomodulin, TNF-α and NF-KB in coronary artery disease patients of Pakistan. Mol Biol Rep 2020;47:7575-82. [Crossref] [PubMed]
- Mukherjee N, Jacobs NC, Hafner M, et al. Global target mRNA specification and regulation by the RNA-binding protein ZFP36. Genome Biol 2014;15:R12. [Crossref] [PubMed]
- Zhang H, Taylor WR, Joseph G, et al. mRNA-binding protein ZFP36 is expressed in atherosclerotic lesions and reduces inflammation in aortic endothelial cells. Arterioscler Thromb Vasc Biol 2013;33:1212-20. [Crossref] [PubMed]
- Ilhan F, Kalkanli ST. Atherosclerosis and the role of immune cells. World J Clin Cases 2015;3:345-52. [Crossref] [PubMed]
- Cochain C, Zernecke A. Macrophages and immune cells in atherosclerosis: recent advances and novel concepts. Basic Res Cardiol 2015;110:34. [Crossref] [PubMed]
- Gao J, Shi L, Gu J, et al. Difference of immune cell infiltration between stable and unstable carotid artery atherosclerosis. J Cell Mol Med 2021;25:10973-9. [Crossref] [PubMed]
- Spitz C, Winkels H, Bürger C, et al. Regulatory T cells in atherosclerosis: critical immune regulatory function and therapeutic potential. Cell Mol Life Sci 2016;73:901-22. [Crossref] [PubMed]
- Riksen NP, Stienstra R. Metabolism of innate immune cells: impact on atherosclerosis. Curr Opin Lipidol 2018;29:359-67. [Crossref] [PubMed]
- Ding R, Gao W, He Z, et al. Circulating CD4+CXCR5+ T cells contribute to proinflammatory responses in multiple ways in coronary artery disease. Int Immunopharmacol 2017;52:318-23. [Crossref] [PubMed]
- Olson NC, Sitlani CM, Doyle MF, et al. Innate and adaptive immune cell subsets as risk factors for coronary heart disease in two population-based cohorts. Atherosclerosis 2020;300:47-53. [Crossref] [PubMed]
- Naruko T, Ueda M, Haze K, et al. Neutrophil infiltration of culprit lesions in acute coronary syndromes. Circulation 2002;106:2894-900. [Crossref] [PubMed]